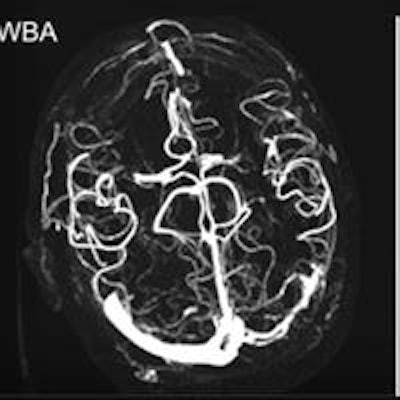
Visualization of cerebral vessels at CT angiography can be vastly improved with the use of a novel technique called wavelet transform, which boosts signal-to-noise ratios as much as ninefold in 3D datasets and improves image quality overall, according to German researchers.
Compared with a temporal maximum intensity projection (tMIP), an advance on a MIP, the mean contrast-to-noise ratio (CNR) for wavelet construction (WBA) was significantly better in every vessel type. Judged qualitatively on a Likert scale, WBA was superior in most categories, including vascular noise, vascular contour, small details, visualization of collaterals, and overall image quality, reported radiologists from Ludwig-Maximilians University Munich in Germany.
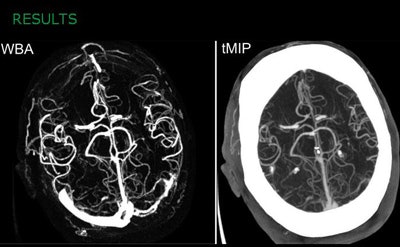
"Our proposed WBA method provides ninefold better CNR compared to tMIP," said lead study author Lukas Havla, PhD in a presentation at the RSNA 2014 congress. "It removes nonenhanced structures such as bone, and qualitative reading of WBA gives us results rated better than tMIP in five of eight categories."
In acute ischemic stroke, information about the hemodynamics of cerebral vasculature of is essential for good decision-making, he noted. CT angiography and CT perfusion (CTP) are the most common imaging sequences in cerebrovascular CT.
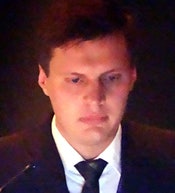
Acquiring images in a single phase, however, can help optimize bolus timing, so there are advantages to calculating angiographic images using CTP data; in 1998, it was shown that a proposed tMIP reconstruction could improve visualization of leptomeningeal collaterals, he said.
"In our study, we wanted to introduce a new method for calculating angiographic images based on wavelet transform postprocessing of CT perfusion data, and we wanted to compare our new method to tMIP quantitatively and qualitatively," Havla said.
The study originally included 15 patients with suspected stroke, but one subject was eliminated due to severe motion artifact, leaving 14 patients with a mean age of 77 years. Images were acquired on CT with a volume of 99 axial slices and an increment of 1.0 mm. In all, 32 phases were acquired at 80 kV/200 mAS following the intravenous administration (typically) of 35 mL or iomeprol 350 (Imeron-350, Bracco Diagnostics) at 4.5 mL/s.
Havla and colleagues Dr. Maximilian Reiser, Dr. Kolja Thierfelder, Sebastian Beyer, Dr. Wieland Sommer, and Olaf Dietrich, PhD, from the radiology department at Ludwig-Maximilians-University in Munich, calculated angiographic datasets after rigid-body motion correction using tMIP as well as the proposed wavelet method, WBA, performed with the commonly used Paul-wavelet. The team calculated the wavelet power spectrum of the time-attenuation curves for each pixel and displayed the maximum of this spectrum as angiographic signal intensity.
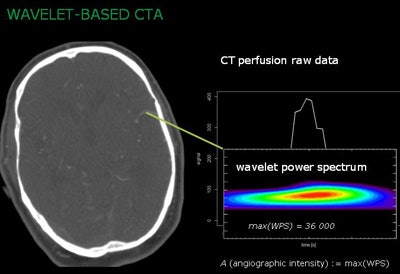
They then compared both tMIP and wavelet transform to the CNR relative to surrounding tissue of 16 different vessel segments. CNR was defined as the signal in the vessel minus the signal in the background divided by the standard deviation.
"The wavelet transform yields a 3D angiographic representation of our 4D dataset," Havla explained.
The group evaluated the images qualitatively using a five-point Likert scale (0 meaning nondiagnostic, 4 excellent) for image quality by two blinded and experienced radiologists. For rigid body motion correction, they used the elastix toolbox (Klein et al, 2010), performing all other calculations on software developed in-house (based on IDL 8.3), including calculation of tMIP and WBA images.
"The first step of wavelet transform is to select the proper function as a mother wavelet," Havla said. "We use a Gaussian curve for the wavelet transform; that is in contrast to the Fourier transform where you have a sinus curve as a basic function. In wavelet transform, you have two degrees of freedom: the translation and the scaling of the wavelet. So the wavelet transform of any curve is defined as an integral of our input signal times the translated and scaled and normalized version of the wavelet."
"So what we have done is define the maximal value as angiographic," he said. "In comparison, a noisy voxel has much less signal, resulting in a less angiographic entity."
The results showed a CNR for the wavelet reconstruction (580.2 ± 474.5) that was significantly higher than the tMIP approach (60.7 ± 31.0, Wilcoxon test p < 0.00001). Compared quantitatively, the new method performed significantly better than the tMIP approach, with a mean score of 3.7 (both readers), and interobserver Cohen's Κ = 1 versus tMIP scores of 2.8 Κ = 0.594 (p < 0.001).
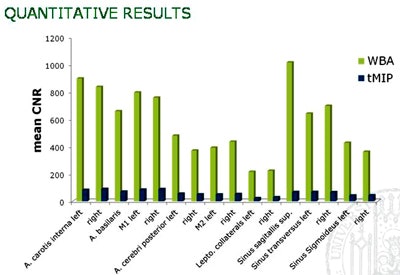
In particular, small vessels that could barely be seen on tMIP images were more easily visualized on WBA images, he said. Reader confidence was improved reading WBA versus tMIP images. Qualitatively, there was no difference between the two methods in middle superimpositions or motion artifacts, Havla said.
"To conclude, our proposed WBA method provides ninefold better CNR compared to tMIP images," he said. The method also intrinsically removes bony structures, and it can be used to classify other vessel features, he said.