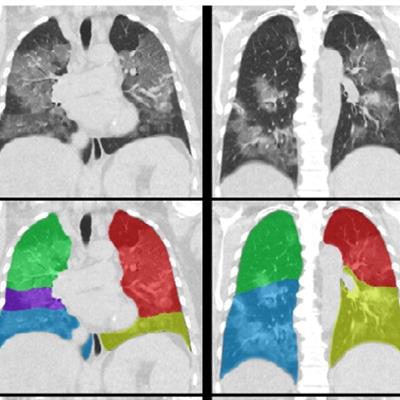
A freely available artificial intelligence (AI) algorithm can yield comparable diagnostic performance to radiologists for assessing the likelihood and severity of COVID-19 on noncontrast chest CT exams in patients suspected of having the disease, according to research published online in Radiology on 30 July.
CORADS-AI is a system of three deep-learning algorithms that automatically segments the five pulmonary lobes. It was developed by a team of researchers led by first author Nikolas Lessmann, PhD, and senior author Bram van Ginneken, PhD, of Radboud University Medical Center in Nijmegen, the Netherlands.
CORADS-AI assigns a COVID-19 Reporting and Data System (CO-RADS) score for suspicion of COVID-19 and provides a CT severity score (CTSS) for the degree of parenchymal involvement per lobe. In testing, CORADS-AI yielded high diagnostic accuracy, as well as severity assessments that had moderate to substantial agreement with radiologists.
"We believe the AI system may be of use to support radiologists in standardized CT reporting during busy periods," the authors wrote. "The automatically assessed CT severity scores per lobe may have prognostic information and could be used to quantify lung damage, also during patient follow-up."
Standardized scoring
Standardized CT scoring systems -- such as CO-RADS for assessing the likelihood of COVID-19 based on unenhanced chest CT exams, and the CTSS for reporting the extent of parenchymal involvement -- can enable fast and consistent clinical decision-making, according to the researchers. Meanwhile, a number of AI algorithms have been developed for automated reading of COVID-19 CT scans, but their practical value is debatable, according to the researchers.
"Without adhering to radiological reporting standards, it is doubtful whether these algorithms provide any real benefit in addition to or instead of manual reading, limiting their adoption in daily practice," the authors wrote.
As a result, the researchers sought to train and validate an AI algorithm that automatically scores chest CT scans of suspected COVID-19 patients based on the CO-RADS and CTSS systems. They developed CORADS-AI using data from 476 patients who had received chest CT for clinical suspicion of moderate to severe COVID-19 at an academic medical center in the Netherlands.
Internal testing of the algorithm was performed on a separate set of 105 patients that had been previously reported by seven chest radiologists and one radiology resident for an observer study assessing CO-RADS. The researchers also further tested the model on an external test set of 262 patients from a teaching hospital in the Netherlands.
Performance of CORADS-AI for distinguishing between positive and negative COVID-19 cases | ||||
Eight radiologists on internal test set | CORADS-AI on internal test set | Radiologist-reported CO-RADS scores on external test set | CORADS-AI on external test set | |
Area under the curve | n/a | 0.95 | n/a | 0.88 |
Sensitivity | 61.4% | 85.7% | 74.9% | 82% |
Specificity | 99.7% | 89.8% | 89.2% | 80.5% |
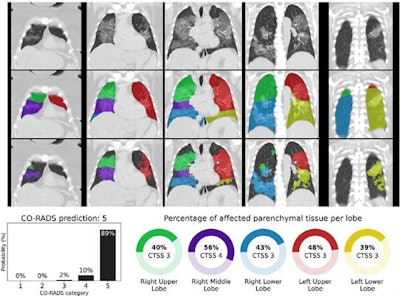
In the external test set, the algorithm's CO-RADS score was in absolute agreement with the median CO-RADS score of all combinations of seven of the eight readers in 64.1% of the cases, and within one category in 85.5% of cases. The researchers found moderate-to-substantial agreement (kappa = 0.69) between the AI CO-RADS scores and observers (kappa = 0.60 for the internal test set and 0.69 for the external test set).
Predicting disease severity
As for predicting the CT severity score, the algorithm was in absolute agreement with 17 (10.4%) of the 163 radiological reports in the external test set that included a severity score and within one point per lobe in 146 (89.6%) of the reports. Agreement was considered to be moderate (kappa = 0.49).
"An explanation [for the moderate agreement] may be that visually estimating the amount of affected lung parenchyma is subjective; studies have shown that human readers tend to overestimate the extent of disease," the authors wrote. "In the four cases where automatic measurements were >10 points higher than the reference, underlying causes were severe motion artifacts in three cases and one patient with opacifications caused by aspiration pneumonia. This underlines the importance of verification of automatically determined severity scores by humans."
The study demonstrates that an AI system can identify COVID-19 patients based on noncontrast chest CT images and provide comparable diagnostic performance to radiologists, according to the researchers.
"It is noteworthy that the algorithm was trained to adhere to the CO-RADS categories and is thus directly interpretable by radiologists," they wrote.