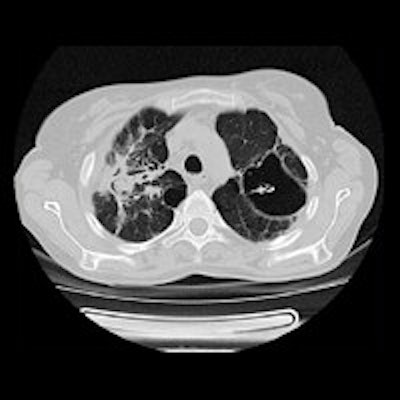
Researchers in Belarus have developed a computer-aided diagnosis (CAD) scheme that can fairly accurately predict drug resistance in tuberculosis (TB) patients. The results show that the prediction tool could potentially improve triage and care of TB patients.
The study applied a texture analysis tool to two groups of patients: one group of 107 patients who either had known resistance or lack of resistance to TB drugs, and second group of 62 patients whose resistance status was unknown.
The algorithm was able to predict TB drug resistance with almost 76% accuracy, according to the researchers, who presented their study at the Computer-Assisted Radiology and Surgery congress (CARS 2015) in Barcelona.
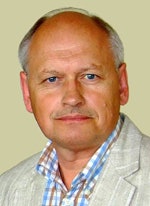
"We have demonstrated that it is possible to distinguish ... the drug-sensitive and drug-resistant cases of lung tuberculosis based on an in-depth computer analysis of radiological lung images," study author Vasiliy Kovalev wrote in an e-mail to AuntMinnieEurope.com. Kovalev is head of biomedical image analysis at the United Institute of Informatics Problems at the National Academy of Sciences of Belarus.
The results suggest "statistically significant links between the computerized ... features extracted from radiological images and drug resistance status of lung tuberculosis patients," they wrote in the study abstract (International Journal of Computer Assisted Radiology and Surgery, June 2015, Vol. 10:1 suppl, pp. S129-S130).
Drug resistance rises
Tuberculosis remains a serious public health problem in many countries due to the rising incidence of drug-resistant cases. To distinguish drug-sensitive from drug-resistant cases, this study looked at the use of features on CT and x-ray images to predict drug resistance in tuberculosis patients.
Any development that contributes toward the fast, computerized prediction of resistance status based on morphology of routine medical images is highly desirable, the research team wrote. Having a workable methodology could also open doors to new applications for drug resistance assessment.
"Since there are certain correlations between the morphology of lung images and the tuberculosis resistance phenomenon, we may hypothesize that such links may be discovered also between the drug-resistance status of some other diseases and the structure of biomedical images taken from corresponding organs and/or functional systems of patients," Kovalev wrote.
Known and unknown status
The investigators relied on two separate groups of tuberculosis patients. Group 1 consisted of 107 patients with known drug resistance status (64 men, mean age 42.7 years; 43 women, mean age 48.3 years). Forty-one patients were coded 0, meaning they were sensitive to TB drugs, or coded 1, denoting any level of drug resistance, the authors explained.
Group 2 (the validation group) consisted of 62 TB patients (38 men, age 43.4 years; 24 women, mean age 41.9 years) with unknown resistance to TB drugs.
Predicting drug resistance was handled as a pattern recognition problem with the aid of several classifiers, the authors wrote.
The chest radiographs were acquired using a commercially available computed radiography (CR) system (Point-of-Care CR 260 system, Carestream Health) with 2,248 x 2,248-pixel resolution, while CT scans were acquired on a 16-detector-row scanner (LightSpeed Pro 16, GE Healthcare) at a slice thickness of 2.5 mm.
Lung regions were segmented semiautomatically on CT and manually on radiographic images, with final inspections performed by a radiologist, Kovalev and colleagues wrote.
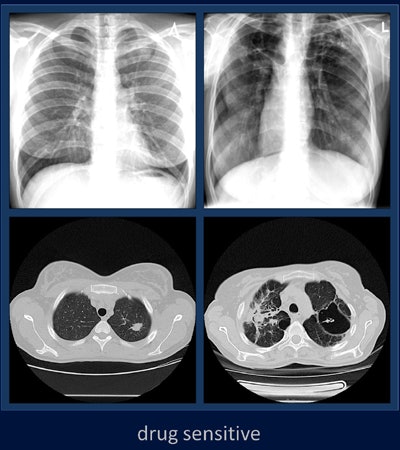
Feature analysis
The CAD algorithm performed an analysis on the data from 107 patients, developing a prediction as to each patient's drug resistance status.
The technique predicted the resistance status of each case in the validation group based on several steps:
- Train all classifiers using all 107 patients of the study group to be used for predicting the target drug resistance of patients of the validation group.
- Calculate quantitative image descriptors based on texture. The investigators applied an extended multisort, multidimensional co-occurrence matrix approach flexible enough to capture a wide range of structural properties of both 2D and 3D medical images.
- Create feature table for the validation group. The co-occurrence matrix elements of each image were stored on a standard object-feature table consisting of 62 rows and some columns representing pairwise-uncorrelated principal components.
- Predict resistance status using data table and pattern-recognition methods.
- Store predicted resistance status and obtain anonymous patients' identification to determine true resistance status and calculate results.
The best prediction performance (75.7%) was found in the logistic regression classifier applied to both x-ray and CT image features in addition to the binary feature of whether treatment was recurring.
The accuracy for predicting TB drug resistance was 73%, with sensitivity of 82% and specificity of 58% based on blinded results in the 62 patients in the validation group obtained using the corresponding receiver operator characteristic (ROC) curve patient categorization matrix, the authors wrote.
The results suggest that the drug resistance of lung tuberculosis can be predicted based on radiological images with accuracy of about 70%, the team concluded. Incorporating the prediction model into easily retrievable nonimaging clinical data may increase the prediction accuracy further, Kovalev said.
The group believes accuracy could be increased by incorporating easily available nonimaging data into the technique.
"If so, the suggested method can be potentially used as one more source of information for quick prognosis of the resistance status of new coming patients instead/along with the long-lasting microbiology tests," the study authors wrote.
Some sensitivity gains might be realized by scanning with CT slices thinner than 2.5 mm, "but probably not much," Kovalev wrote. Of note, the in-slice, X-Y resolution of the images remains high, and the features appear to be smaller than nodules or other visible features.
Looks like drug resistance
Can radiologists see signs of drug resistance by patterns in the lung parenchyma?
Some manifestation of the differences between drug-sensitive and drug-resistant cases in the lung parenchyma and lesion structure may be visible, Kovalev wrote. But a deeper multidisciplinary study of resistance will still be necessary to identify and isolate any signs in radiologic images.
Even so, "my experience suggests that it is often the case in biomedical image analysis studies that computerized features and radiological (human) visual features could be very different," Kovalev wrote.
Doctors can be excellent at detecting lesions, calcifications, etc., but diseases can manifest nonlocally -- for example, just by changing the proportions of structures occupied by light or dark pixels on an image. What humans can't see, computers often can, Kovalev wrote.
The work was funded by the U.S. National Institutes of Health in Bethesda, MD.