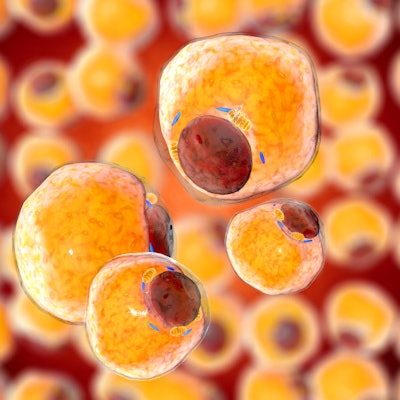
An artificial intelligence (AI) algorithm can be used to provide automated body composition analysis on routine abdominal CT exams, enabling these valuable biomarkers to be accessed as part of routine clinical imaging, according to research published online on 18 September in European Radiology.
A team of researchers led by Sven Koitka of the University Hospital Essen in Germany trained a deep-learning model to provide automated quantitative 3D volumetry of body tissue composition from standard abdominal CT exams. Their algorithm was found to be highly reliable on a test case set.
"Our results show that fully automated body composition analysis on routine CT imaging can provide stable biomarkers across the whole abdomen and not just on L3 slices, which is historically the reference location for analyzing body composition in the clinical routine," the authors wrote.
The researchers trained their 3D semantic segmentation convolutional neural network using an internal dataset of 40 CT exams. Testing was performed using an additional 10 CT studies.
The abdominal cavity, bones, muscle, subcutaneous tissue, and thoracic cavity were fully annotated on every fifth axial slice for all 50 CT exams. The researchers applied multiresolution U-Net 3D neural networks to first segment these body regions and then subclassify adipose tissue and muscle using known Hounsfield unit limits.
In testing, the algorithm yielded an average Sørensen Dice score over all semantic regions of 0.9553. What's more, the intraclass correlation coefficients for subclassified tissues were above 0.99.
External validation of the system is now required in order to prove its stability and generalizability, according to the researchers.
"In the future, we plan to extend the system to thoracic examinations and to add important tissue classes such as pericardial adipose tissue and myocardium," the authors wrote.