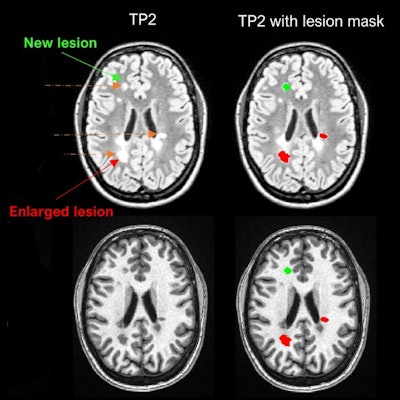
Automated clinical decision support integrated into the radiological routine flow can assist in the diagnosis and follow-up of multiple sclerosis (MS) patients, a multicenter Swiss study has found.
Researchers used clinical data settings acquired at 1.5 and 3-tesla from five different facilities and involving a variety of MRI protocols. They published their results on 28 January in the Journal of Magnetic Resonance Imaging (JMRI).
"Detecting new and enlarged lesions in MS patients is needed to determine their disease activity," explained joint first authors Dr. Alexandra Ramona Todea, a neuroradiologist at the University Hospital of Basel, and Lester Melie-Garcia, PhD, a postdoctoral researcher at Swiss Federal Institute of Technology Lausanne (EPFL).
Lesion location and the identification of new and enlarging white-matter lesions contribute to the diagnostic criteria for MS -- i.e., dissemination in space and time -- and also to an evaluation of activity, which might lead to escalation of the immunomodulatory therapy. Identifying changes in lesion number is prone to intraobserver and interobserver variability.
According to the authors, "Most existing tools dedicated to evaluating MS lesion evolution allow a cross-sectional analysis, and only a few support the longitudinal assessment. These tools can be roughly classified into two main groups: lesion segmentation-based methods and change detection-based methods."
Study logistics
The main goal of the Swiss investigators was to assess the diagnostic accuracy of software for detecting new and enlarging white-matter lesions in MS patients.
Between 2012 and 2019, they recruited a total of 206 patients with a definitive MS diagnosis and at least two follow-up MRI studies from five centers that were participating in the Swiss MS Cohort Study. The mean age at first follow-up was 45.2 (range, 36.9-52.8 years), and 136 were women.
Fluid attenuated inversion recovery (FLAIR) and T1-weighted magnetization prepared rapid gradient echo (T1-MPRAGE) sequences at 1.5 tesla and 3 tesla. The study included 313 MRI pairs of datasets. Data were analyzed with LeMan-PV and compared with a manual "reference standard" provided by a neuroradiologist. A second person (a neurologist) performed the same analysis in a subset of MRI pairs to evaluate the rating accuracy.
The sensitivity, specificity, accuracy, F1-score, lesion-wise false-positive rate (aFPR), and other measures were used to assess software performance for the detection of new and enlarging white-matter lesions. The performance was also evaluated in the subgroup of 123 MRI pairs at 3-tesla MRI acquisitions were performed at 1.5-tesla (four centers, 190 pairs, 380 scans) and 3-tesla (two centers, 123 pairs, 246 scans) using the 20-channel commercial head coils on all scanners.
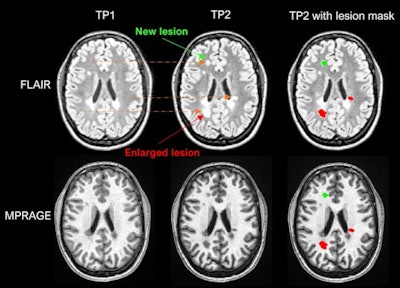
The interreader agreement was high for detecting new lesions and good for detecting enlarged lesions, the authors noted. Across all centers, scanner field strengths, and for NEL, LeMan-PV achieved an accuracy of 61%, sensitivity of 65%, and specificity of 60%. The F1-score was 0.44, and aFPR was 1.31. When both follow-ups were acquired at 3 tesla, the accuracy, sensitivity, and specificity were 66%, and the F1-score was 0.28 and aFPR was 3.03.
Assessment of software
While LeMan-PV performance is not optimal, overall, the software showed similar sensitivity in detecting new and enlarged lesions with respect to other recent 3-tesla multicentric studies based on neural networks, the authors stated.
"LeMan-PV is software that combines lesion segmentation and change detection-based approaches to perform longitudinal lesion detection in MS patients," they added. "This software is based on the k-nearest neighbor (k-NN) technique and is optimized to detect lesions with a volume as small as three connected voxels (typically 0.0036 mL)."
Embedded in the scanner reconstruction system, the software has been developed to estimate the partial volume, which improves the volume estimation of new lesions, they continued.
"Baseline and follow-up T1-weighted magnetization prepared rapid acquisition with gradient echo and 3D fluid-attenuated inversion recovery images are used as inputs for the segmentation method. The output provides a segmentation mask indicating the new, enlarged, and stable white-matter lesions," the authors wrote.
The advantage of this software is that it generates segmentation masks that permit visual assessment of its reliability, a fundamental characteristic of a clinical decision-support tool, they concluded.