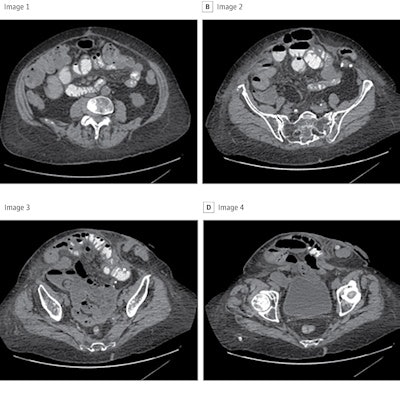
A deep-learning model used with preoperative CT helps predict the complexity and outcomes of abdominal surgery more accurately than surgeons' judgment, according to research published on 7 July in JAMA Surgery.
The study could help clinicians better plan hernia treatment, wrote a team led by Dr. Sharbel Adib Elhage of Franciscus Gasthuis en Vlietland Hospital in Rotterdam, the Netherlands.
"In the case of ventral hernia repair, deep-learning models may be able to objectively aid in delineating which patients can have surgery safely and effectively performed in a community hospital versus those who should have the hernia repaired at a specialized hernia referral center," the group wrote.
The repair of ventral hernias is a common surgery, but when it requires abdominal wall reconstruction, the incidence of wound complications and recurrences increases, the team noted.
Image-based deep-learning models have been used in other medical disciplines, but not necessarily to attempt to predict abdominal surgery outcomes.
Therefore, Elhage's group sought to assess the effectiveness of three models for predicting the complexity of abdominal surgery -- that is, the need to separate layers of the abdominal wall to treat the hernia -- as well as pulmonary or wound complications after the area has been reconstructed.
The study included data from 369 patients with ventral hernias who underwent abdominal wall reconstruction but had preoperative CT exams (9,303) between September 2019 and January 2020. Most patients were female (62.9%) and white (87.5%). The deep-learning models were created using an eight-layer convolutional neural network to analyze image characteristics; 80% of the images were used for training and 20% for test sets. The models' performance was compared with six abdominal wall reconstruction surgeons' predictions.
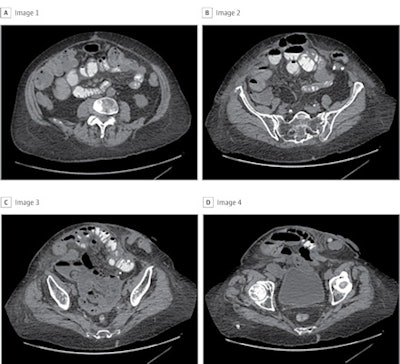
The surgical complexity deep-learning model validation set showed a receiver operating curve (ROC) of 0.74, and when its performance was compared with surgeons' predictions, it had higher accuracy (81.3% vs. 65%).
Deep-learning model vs. surgeon predictions for abdominal surgery complexity | ||
Measure | Surgeons | Deep learning model validation set |
ROC | 0.64 | 0.83 |
Accuracy | 65% | 81.3% |
Sensitivity | 53.3% | 88.9% |
Specificity | 76.7% | 73.5% |
The researchers found that the deep-learning model for estimating surgical site infection had an ROC of 0.89, accuracy of 85.2%, sensitivity of 78.5%, and specificity of 87.4%; the ROC for the model to forecast pulmonary failure was lower, at 0.54, with accuracy of 77.5%, sensitivity of 26.9%, and specificity of 84%. (Surgeon estimates of surgical site infection and pulmonary failure were not considered for these two models, as they aren't regularly predicted by surgeons based on preoperative imaging, according to the team.)
"[Our] study demonstrates that deep-learning models developed using only preoperative CT imaging inputs may successfully predict surgical complexity and the occurrence of surgical site infection in patients who underwent abdominal wall reconstruction," the authors concluded.
The study offers a vision for future patient care around this common surgical procedure, wrote Dr. Amin Madani, PhD, of Toronto General Hospital and colleague Dr. Liane Feldman of McGill University in Montreal.
"[This] proof-of-concept study will undoubtedly stimulate surgeons, innovators, and data scientists to continue investigating the role of machine-learning methods to improve care," Madani and Feldman wrote.