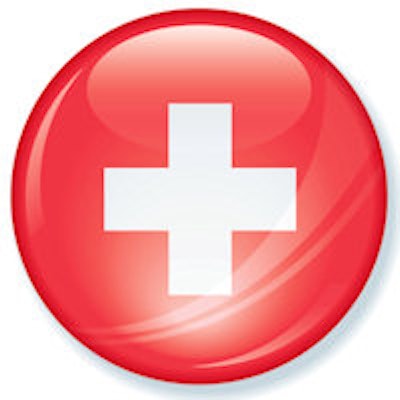
Deep-learning methods have potential for finding imaging biomarkers in relatively uncommon diseases such as nasopharyngeal cancer, Swiss researchers reported at the recent RSNA 2016 meeting in Chicago.
As part of an effort to better understand this rare cancer, a team from University Hospital Basel in Switzerland and Swiss image analysis software developer 4Quant developed and trained a deep-learning model that could find and extract important tumor features as well as classify MR images.
"Deep learning is capable of incorporating texture, position, and morphological information in very impressive -- if poorly understood -- ways that previous techniques could not," said presenter Kevin Mader, PhD, of 4Quant."Deep learning will not replace radiologists any time soon, but it can accelerate boring, tedious tasks significantly, and provide high-level screening to large groups of patients."
A rare cancer
The research stems from an initial collaboration between University Hospital Basel and 4Quant on a project exploring nasopharyngeal cancer, a rare cancer worldwide but unusually common in southern China. Early detection plays a crucial role in successful outcomes with nasopharyngeal cancer. A number of different treatment options are available with varying levels of side effects; both staging and grading are critical for optimizing patient care, according to the researchers, who shared their work in a poster presentation at RSNA 2016.
In that initial project, MR images from 14 patients were manually and painstakingly analyzed in a process that took over two hours per patient, Mader said. However, the study was ultimately inconclusive due to the small number of patients. A new study recruited 200 patients, but the researchers were overwhelmed by the analysis time of two hours per patient.
"We decided to apply a big-data and deep-learning approach to learn the most important features and how to extract them and classify images accurately," he told AuntMinnieEurope.com.
Deep-learning model
In the hope of finding tumors and predicting outcomes, the team sought to build a deep-learning model using 12,000 images from head MR examinations. First, the MR images were preprocessed through two different neural networks: a "neural image sorter" and a "neural position estimator." The 20-layer neural image sorter created an estimate of the type of image being input, while the 20-layer neural position estimator helped to pinpoint the position of the image within the frame, according to the group.
Next, the output of the first two neural networks was combined with the original image data and processed in two other neural networks: a "staging network" and an "outcome network." The staging network outputs a T, N, and M stage, while the outcome neural network provides an estimate of recurrence or remission.
"The setup allows for training piecemeal or 'end-to-end,'" the authors wrote. "Examining thousands of images causes the performance of the staging to improve drastically."
In testing, the researchers found their deep-learning model turned in a very strong performance in detecting nasopharyngeal cancer and measuring tumor features. The model's tumor volume calculations had a 96% correlation with the radiologist's calculations.
Room for improvement
There was room for improvement, however, when it came to making a diagnosis and staging the cancer. Only 45.5% of the tumors were staged correctly by the model, although more than 90% were staged within one category:
- Understaged by one category: 25.8%
- Correct staging: 45.5%
- Overstaged by one category: 21.2%
- Overstaged by two categories: 7.6%
The group noted that while current deep-learning technology is ready for radiology, the black box nature of artificial intelligence and data acquisition pose serious challenges.
"Understanding and tracing the decisions from a large neural network is a very much open, unsolved problem and is essential for clinical acceptance," Mader noted.
4Quant is a spin-off of the ETH Zurich and the Paul ScherrerInstitut, and its work is based on the PhD thesis of Kevin Mader done in the X-Ray Microscopy Laboratory of Marco Stampanoni. The business is funded by the Pioneer Fellowship of the ETH Zurich and is a member of the ETH Innovation and Entrepreneurship Lab.