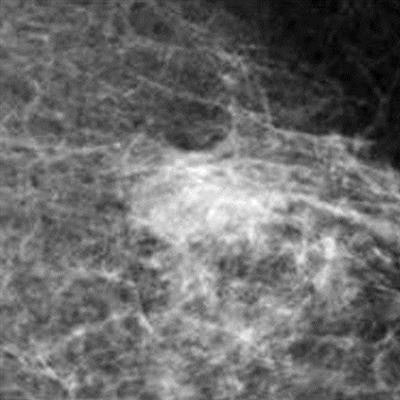
A convolutional neural network (CNN) trained on a large dataset of mammographic lesions outperformed a conventional mammography computer-aided detection (CAD) scheme, according to a new Dutch study published this month. CNNs can be fine-tuned based on radiologists' knowledge.
Aiming to develop a system that can read mammograms independently, researchers from Radboud University in Nijmegen and Amsterdam University Medical Center offered a head-to-head comparison between a state-of-the-art mammography CAD system and a CNN -- both of which were trained on about 45,000 images. They analyzed the results to determine which manually developed features such as location and patient information can improve the CNN's accuracy. A reader study found no significant difference between the CNN and three readers.
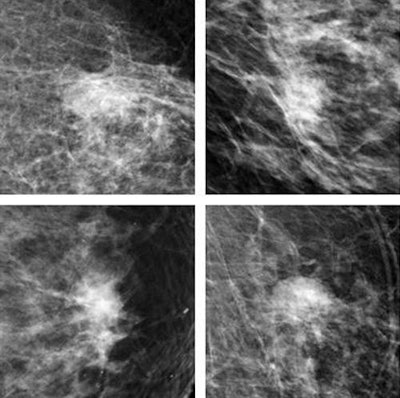
"We believe deep learning methods signify a paradigm shift in CAD and expect to see a lot of breakthroughs in the next couple of years," lead author Thijs Kooi, a doctoral candidate in Radboud University's Diagnostic Image Analysis Group, told AuntMinnieEurope.com. "We have shown we can get state-of-the art performance and operate at a level of expert human readers with relatively little engineering effort. Results also show the performance of the CNN increases with more training examples, and we expect it to become even better if more data is available."
By outperforming a state-of-the-art CAD system on a large dataset, CNNs have shown great potential to advance the field of research, wrote Kooi and colleagues Dr. Geert Litjens, Dr. Bram van Ginneken, Dr. Albert Gubern-Mérida, and Nico Karssemeijer PhD (Medical Image Analysis, 2 August 2016).
Reading some 40 million mammograms performed in the U.S. each year is expensive and time-consuming, and even the best-trained readers make mistakes, so even conventional CAD, now used in the majority of breast screening exams, has been helpful.
"Computers do not suffer from drops in concentration, are consistent when presented with the same input data, and can potentially be trained with an incredible amount of training samples, vastly more than any radiologist will experience in his lifetime," the authors noted.
Traditionally, CAD systems were created from meticulously hand-crafted features, combined with a learning algorithm to map each feature to a decision variable. Radiologists are often consulted in the process of feature design to evaluate qualities such as lesion contrast, spiculation patterns, and border sharpness in the case of mammography.
These feature transformations provide a platform for human knowledge, but they bias the results "towards how we humans think the task is performed," they wrote. The development of artificial intelligence (AI) has witnessed a "shift from rule-based problem-specific solutions to increasingly generic."
By distilling information directly from training samples, deep learning (i.e., incorporation of layered nonlinearities in a learning system) enables the use of ever-larger data troves, reducing human bias. As a result, many pattern-recognition tasks are meeting or exceeding human performance.
Modern CAD systems are trained to recognize the two components of breast cancers: malignant soft tissues and microcalcifications. There has been good progress in microcalcifications, but studies suggest that soft-tissue analysis needs further improvement.
Does CNN beat CAD?
This study compared CNN with a CAD system created from a comprehensive set of manually designed features to determine whether CNN outperforms it on a large dataset of 45,000 images. The analysis focused on the detection of solid malignant lesions, including architectural distortions, considering benign abnormalities such as cysts or fibroadenomas as false positives.
"The goal of this paper is not to give an optimally concise set of features, but to use a complete set where all descriptors commonly applied in mammography are represented and provide a fair comparison with the deep learning method," Kooi and colleagues wrote. To that end, a candidate detector obtains a set of suspicious locations, which is then subjected to scrutiny via the classical CAD system or the CNN. Next, the team looked at the extent to which CNN is still complementary to traditional descriptors by combining learned representation with features such as location, contrast, and patient information. Finally, a reader study compared CNN scores to those of experienced radiologists on patches of mammographic data.
Candidate detection, deep CNN, and analysis
Detection follows a two-stage classification process in which candidates are identified and further scrutinized by an algorithm designed for mammographic lesions that extracts five features, two designed to find the center of a focal mass, and two looking for spiculation patterns characteristic of malignant lesions, as well as a fifth feature indicating the size of the optimal response.
Inspired by human visual processing, CNNs learn hierarchies of filter kernels, creating a more abstract data representation in each layer. Deep CNNs are the most proficient for vision and have been shown to be quite powerful, the authors explained. The architecture comprises convolutional, pooling, and fully connected layers. Every convolution produces a feature map that is down sampled in the pooling layer. Data augmentation is performed by generating new samples from existing data in an effort to lessen data scarcity and prevent overfitting.
The present system employs a mass segmentation system that has proved superior to region growing and active contour segmentation. In mass segmentation, the image is transformed to a polar domain around the center of the candidate, and dynamic programming is used to find an optimal contour. A cost function is then used to find an optimal segmentation, the authors wrote.
A problem with this and other knowledge-driven segmentation methods is that it is conditioned on a false prior: The size constraint is based on data from malignant lesions. The methodology deals with this by assuming malignancy, inasmuch as the goal is an accurate diagnosis, not an exact delineation of tumors.
After segmentation, the researchers extracted and categorized 74 features, including contrast features, texture features, geometry features, and context features that capture information about the rest of the breast and convey background information. The mammography data were collected from a large-scale Dutch screening program (bevolkingsonderzoek midden-west) acquired on a digital mammography system (Selenia, Hologic).
Tumors were biopsy-proven and annotated. Images for each case were typically acquired over two years. The training and validation set included 44,090 mammographic views, including 39,872 used for training and 4,219 for validation. The test set included 18,180 images of 2,064 patients with 271 malignant lesions.
The group experimented with several classifiers for second-stage classification, including support vector machines (SVM) with several different kernels, gradient boosted trees, and multilayered perceptrons on a validation set, but nearly always the random forest classifier outperformed the others.
"We subsequently investigated to what extent features such as location and patient information and commonly used manual features can still complement the network and see improvements at high specificity over the CNN, especially with location and context features, which contain information not available to the CNN," they wrote.
CNN improves analysis
In the study, CNN outperformed conventional CAD at low sensitivity and performed comparably at high sensitivity. A reader study also was created to compare the network with certified screening radiologists reading patches; it found no significant difference between CNN and individual readers, but a significant difference between CNN and the mean of all readers.
Performance of convolutional neural network vs. readers | |
CNN vs. readers | P-value |
Reader 1 | p = 0.1008 |
Reader 2 | p = 0.6136 |
Reader 3 | p = 0.64 |
Mean of all readers | p = 0.001 |
CNN's major advantage is it learns from data and does not rely on domain experts, making development easier and faster than that of conventional systems, the authors wrote. The results also show the addition of local information and context can be added easily to the network, and manually designed features can offer small improvements by taking advantage of common-sense instructions.
When the group compared the CNN with a group of three experienced readers, they demonstrated that human readers and the CNN have similar performance.
"The current setup works really well but is still limited in the sense that it only looks at a region of interest, and this is not how radiologists read an exam," Kooi explained in an email. "We are currently working on extending the CNN to also compare left and right breast and look for temporal change. Initial results are promising."
Ideally, however, a CAD system should be able to reason about different findings in an exam -- for example, the fact that the presence of microcalcifications makes a potential mass more suspicious.
"I am currently visiting [Johns Hopkins University] and am working on machine learning techniques that can reason about these different findings," he added. "The goal is to make one decision about an exam that takes into account all available information."