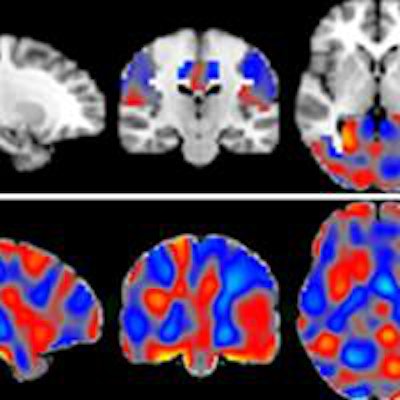
Could techniques like machine learning help radiologists diagnose Alzheimer's disease? Dutch researchers used machine-learning software to analyze arterial spin-labeled MRI scans for signs of abnormal perfusion that could be a predictor of Alzheimer's, according to a study published on July 6 in Radiology.
Alzheimer's disease is known to be a gradual process during which the brain experiences major functional changes prior to structural changes -- such as atrophy of the hippocampus -- that can be detected on imaging exams. Unfortunately, there is currently no way for clinicians to identify people with early dementia, or to tell which individuals with mild cognitive impairment will progress to Alzheimer's disease.
A research team led by Alle Meije Wink, PhD, from VU University Medical Center Amsterdam used machine-learning techniques to analyze arterial spin-labeled (ASL) MRI scans, which create perfusion maps that depict blood flow to various regions of the brain. A machine-learning application was trained to recognize patterns in the maps to distinguish between patients with varying levels of cognitive impairment and to predict the stage of Alzheimer's disease cases that were not yet diagnosed (Radiology, July 6, 2016).
Wink and colleagues studied a subgroup of 260 participants from a dementia cohort at the university's Alzheimer Center who underwent ASL-MRI scans with a 3-tesla scanner (Signa HDxt, GE Healthcare) between October 2010 and November 2012. The group included 100 patients with probable Alzheimer's disease, 60 patients with mild cognitive impairment (MCI), 100 patients with subjective cognitive decline (SCD), and 26 healthy controls.
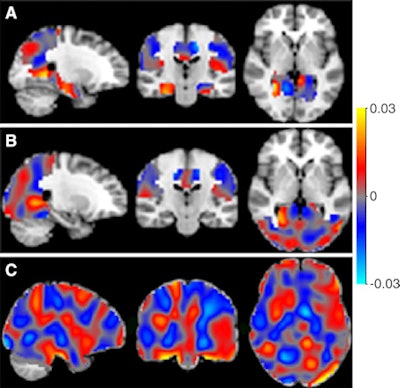
The researchers then analyzed images using an open-access software platform, Pattern Recognition for Neuroimaging Toolbox (Pronto), which enables multivariate analysis of neuroimaging data based on machine learning.
The Pronto application was able to differentiate between individuals with Alzheimer's disease, MCI, and SCD, the researchers reported. It was also able to predict the prognosis of patients with accuracy ranging from 82% to 90%, leading Wink et al to conclude that ASL is a "promising alternative functional biomarker" for the early diagnosis of Alzheimer's disease.
What's more, automated machine-learning methods could be used as a possible screening tool by identifying brain changes on ASL-MRI scans that occur early in the disease process, they concluded.