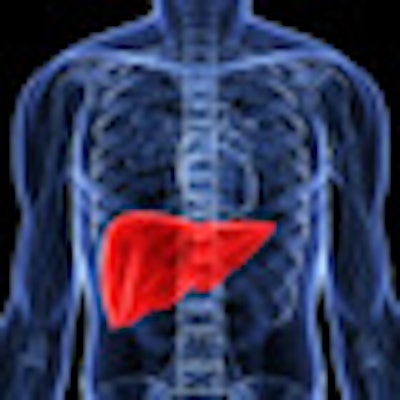
German researchers have found that a computer-aided diagnosis (CADx) system can do better than radiologists for characterizing indeterminate liver lesions, but they believe CAD systems should work more like radiologists rather than relying too heavily on image analysis.
"CAD systems are not used broadly in the hospital because if the doctors use all of their information available, they are way better than the CAD systems," Dr. Peter Dankerl, from University Hospital Erlangen, told delegates at RSNA 2012 in Chicago. "So what we have to do is integrate all possible information into CAD systems, and they have to be able to ... take it into consideration."
Based on computerized image analysis alone, a CADx system developed under the German Theseus-Medico project outperformed radiologists in discriminating liver lesions. The performance of radiologists can be drastically inferior to the system when rating liver lesions with image information limited to the liver and without additional patient-specific information such as previous scans, additional imaging exams, and laboratory values, he noted.
"According to our findings, we may suggest that the computer-based decision-support system of the future should not be based exclusively on image-processing algorithms," Dankerl said. "Rather, it is of paramount importance for it to have access to and make benefit of related medical knowledge and all relevant patient-specific information from the patient record, in addition to being able to make use of all images of the examination, instead of focusing on a single body region of a single scan."
Often-missed lesions
Important liver lesions are often missed on commonly used modalities such as CT and MR, with reported sensitivity rates ranging between 50% and 87%. In addition to challenges with detection, characterization and risk assessment are also difficult, particularly for small lesions, Dankerl said.
Based on the principles of machine learning, the Theseus-Medico CADx system operates much like an image search engine with the possibility of decision support in lesion characterization, Dankerl said. The radiologist supplies a volume of interest (VOI) by drawing around an indeterminate liver lesion and selects a certain similarity criteria to provide context. The radiologist also has the opportunity to provide additional semantic information about the image and the lesion in question, such as contrast agent phase, lesion focality, the presence of enhanced rim, etc., he said.
"Then, according to the selected similarity context and the lesion in question, the system retrieves the lesions of likely similar histology (benign/malignant), texture appearance (hypodense/hyperdense/mixed), and type (cyst/hemangioma/metastasis) from a database of over 2,000 preannotated liver lesions corresponding to over 600 CT scans," Dankerl told AuntMinnieEurope.com.
For each of these characterization factors, the system's retrieval performance was found to reach 90% in terms of area under the receiver operator characteristics (ROC) curve. The researchers then sought to compare the predictive performance of the CADx system with radiologist characterization performance in cases in which both the system and the radiologist only had access to the same amount of image information (axial CT slices displaying only the liver).
Three radiologists -- with two, three, and 16 years of experience, respectively -- independently rated 83 liver lesions (20 hemangiomas, 20 metastases, 20 cysts, 20 hepatocellular carcinomas, and three focal nodular hyperplasias) according to their histology and type. For the purposes of the experiment, the researchers extracted only the axial CT slices containing the liver.
"The rest of the examination was not presented to the readers, and either the native, arterial, or portal venous phase was selected," he said.
Reader accuracy
|
In other findings, interreader agreement by lesion type and malignancy was higher for the two more experienced readers (63% and 71%, respectively, with a Cohen's kappa value of 0.42) than for the most experienced and least experienced readers (43% and 58%, respectively, with a Cohen's kappa value of 0.15).
Also, the most reliable diagnosis was hemangioma (70% agreement), followed by hepatocellular carcinoma (53% agreement), cyst (52% agreement), metastasis (41% agreement), and focal nodular hyperplasia (22% agreement).