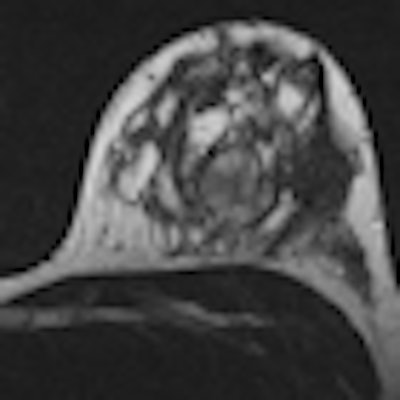
German researchers have developed a decision-support tool that can help radiologists differentiate lesions on breast MRI studies with a high degree of accuracy. Although currently available only as a paper-based manual decision tree, it could ultimately be automated or used with existing tools such as computer-aided detection (CAD).
The decision tree, developed by a team from the University Hospital Jena in Germany, provides percentage estimates of whether a lesion is benign or malignant based on subjective radiologist descriptions of five lesion characteristics on breast MRI. Based on these descriptors, the algorithm classified suspicious lesions with 90% accuracy during testing in more than 1,000 patients, the researchers reported in a study presented at the European Congress of Radiology (ECR). In nearly one-third of cases, classification accuracy exceeded 95%.
"This should facilitate lesion differentiation in clinical practice," said Dr. Pascal Baltzer, who presented the research.
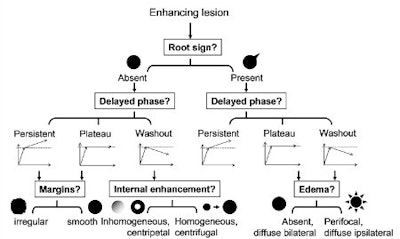
The algorithm is currently available only as a printout that could be consulted by radiologists during their reading process, but in the future it could be combined with a database system in which the observer selects diagnostic criteria from drop-down menus. The software could then present the probability of malignancy in a written structured report that could be included in the radiologist report, Baltzer told AuntMinnie.com.
It's also possible that the classification tree could evolve into a computer-assisted assessment tool that's integrated within CAD at some point, Baltzer said.
An aid for differential diagnosis
While breast MRI has been shown to be the most sensitive method for detecting breast cancer, the differential diagnosis of enhancing lesions can be challenging. There are a multitude of published qualitative and quantitative diagnostic criteria for breast MRI, and radiologists have to differentiate between benign and malignant lesions as accurately and quickly as possible when reporting studies, Baltzer said.
Many of these diagnostic criteria overlap, which also complicates the task.
"That's a problem, because in clinical application we have to use simple diagnostic criteria; everybody has to understand them," he said. "We have to avoid redundant information, and we have to know what our specific combination of diagnostic criteria means, because we want to be sure what diagnosis we are doing here."
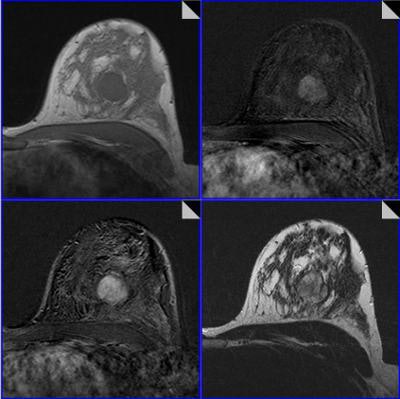
To help, the Jena team sought to develop an algorithm based on a classification tree, a concept in decision-support theory in which a treelike graph or model is developed to predict decisions and their possible consequences. The decision tree generates specific sensitivity and specificity values for each combination of diagnostic criteria, Baltzer said.
"The reader knows then if the diagnosis is certain or not," he said.
The algorithm was developed from a cross-sectional study on a database of consecutive patients over a time period of 12 years. Patients were included in the study if they had histopathologically verified lesions and were referred by the institution's clinic of gynecology. Exclusion criteria included intervention (biopsy, radiation, or chemotherapy) or a prior breast MRI, according to the researchers.
All MR scans were acquired on a 1.5-tesla scanner and included dynamic T1-weighted contrast-enhanced images and T2-weighted images with turbo-spin echo (TSE) sequences without fat saturation. A total of 1,012 patients and 1,084 histologically verified lesions (648 malignant, 436 benign) were included in the study.
Images were read in consensus by two of a total of six radiologists with experience reading more than 500 breast MRI studies. Readers were blinded to histopathological results, and the radiologists used a predefined lexicon of 17 categorical descriptors to describe what they saw on the images.
Of the 17 descriptors, four were related to contrast agent kinetics (initial phase, delayed phase, internal enhancement, and blooming sign), while the remaining 13 dealt with morphology. Because 12 descriptors were determined not to add diagnostic accuracy, the researchers elected to use only five in the classification tree:
- Root sign (absent or present)
- Delayed phase (persistent, plateau, or washout)
- Margins (irregular or smooth)
- Internal enhancement (inhomogenous, centripetal or homogenous, centrifugal)
- Edema (absent, diffuse bilateral or perifocal, diffuse ipsilateral)
After reading the image and describing their findings, the radiologists then consult the tree to determine the probability of lesion malignancy using the five descriptors they included in the report.
From receiver operator characteristics (ROC) analysis, the team found that radiologists using the algorithm had an overall classification accuracy of 88.4%. In more than one-third of all lesions (23.6% of benign cases and 40.9% of all malignant lesions), the classification tree method produced an accuracy of greater than 95%, according to Baltzer.
"The classification tree approach of this study is based on a large, representative database, needs just five of 17 lesion descriptors, is very simple and intuitive to follow, and is therefore applicable in clinical practice," he said.