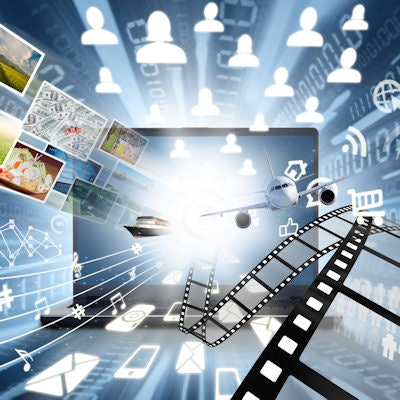
The COVID-19 pandemic has led to a sudden increase in data sharing, multicenter image data collection, online data annotation, deep learning, and the building of large repositories, according to informatics expert Peter van Ooijen, PhD, who calls for more consideration of these topics in radiology training.
"It is not 'just' about the deep learning itself, but also about infrastructure, legal issues, standardization, etc.," he told AuntMinnieEurope.com, noting that a multitude of initiatives from the imaging informatics side have occurred since March, although they are not always communicated as such.
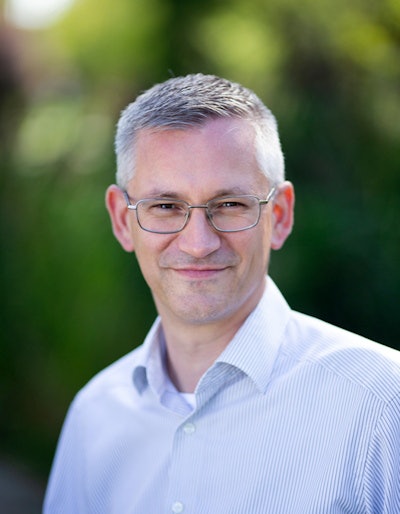
"At EuSoMII [European Society of Medical Imaging Informatics], we are involved in the imagingcovid19ai.eu initiative," added van Ooijen, who is coordinator of the Machine Learning Lab at the Data Science Center in Health (DASH) Department of Radiation Oncology, University of Groningen, University Medical Center Groningen, the Netherlands.
In the radiology learning curricula, there's a strong need to include more training sessions about imaging informatics, particularly artificial intelligence (AI), and medical students and radiologists in training are requiring a more extensive knowledge on imaging informatics issues, he said.
"In the Netherlands, we are covering imaging informatics in the formal training of our residents, but also residents start their own initiatives to organize meetings on these topics," Van Ooijen explained. "In my institution, medical students came to me asking if we could help them to learn more about AI, so they formed their own team, and at DASH, we joined up with them to increase the data science training for medical students."
EuSoMII proposed a plan to the European Society of Radiology (ESR) that is now part of the European Diploma in Radiology (EDiR) curriculum, although no formal examination is currently available. This curriculum has a wide spectrum of topics, ranging from the standards used, such as DICOM/HL7, to the ethical issues surrounding the implementation of decision-support systems and more in-depth knowledge of deep learning.
For the ESR curriculum, EuSoMII proposed different knowledge and skill levels on imaging informatics in the formal training of radiologists -- from the first year of training all the way up to a specialization in medical imaging informatics.
The impact of developments in imaging informatics on the day-to-day work of the radiology department is significant, and given the development of informatics and the implementation of an increasing number of automated software tools, the way radiologists are trained is becoming even more important, Van Ooijen and colleagues wrote in an editorial posted on 19 May by European Radiology.
"Currently, most radiologists lack knowledge and skills in the area of imaging informatics, although there is a clear will to learn about these topics," they noted. "Studies have shown that most radiologists and residents agree that academic training in imaging informatics should be implemented, although it is also recognized that time constraints during radiology training hampers the inclusion of imaging informatics."
Growth of radiomics
A major research trend is radiomics and texture analysis, the popularity of which is due to its symbiosis between high-throughput data and clinical decision-making, the authors continued.
Defined as a data-mining approach aiming to extract high-dimensional data in the form of a multitude of features from clinical images for building machine learning or statistical models, radiomics can be applied to various imaging modalities to answer relevant clinical questions in, for instance, head and neck masses, pancreatic fistulas, hip osteoporosis, lymph nodes, and lung disease.
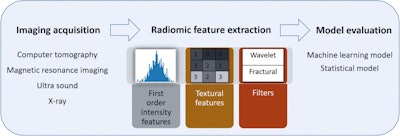
"The successful application of radiomics depends on the different stages in image analysis such as image acquisition, feature extraction, and model validation. Each stage needs to be carefully evaluated to achieve reliable construction of a model that can be transferred into clinical practice for the purposes of prognosis, disease prediction, and evaluation of disease response to treatment," they pointed out.
As part of the radiomics approach, machine-learning techniques can be employed to learn from given examples and detect hard-to-discern patterns from large and complex datasets. This approach leads to the selection of quantitative features that may not be straightforward for a human observer.
"The performance of radiomics models are fluctuating due to high-dimensionality features, some studies report performance that exceeds that of radiologists," the authors observed. "One of the additional advantages of radiomics is that the outcome is shown to be less susceptible for changes in the acquisition protocol."