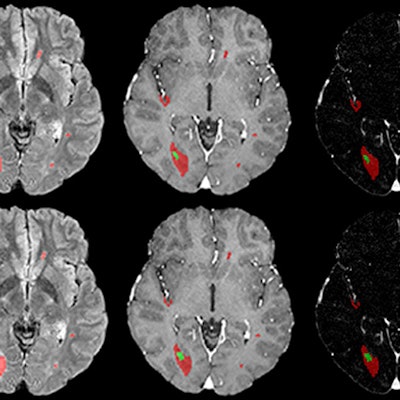
An artificial intelligence (AI) algorithm can accurately evaluate multiple sclerosis (MS) disease burden on MRI exams, potentially enabling better ongoing assessment of these patients, German researchers have reported.
A team led by Dr. Philipp Kickingereder of Heidelberg University trained an artificial neural network (ANN) to provide automated quantitative assessment of MS burden on MRI. After testing the algorithm on clinical patients, the group found that it yielded consistent and accurate performance for assessing volumetric lesion load.
"Computer-aided evaluation of MS with ANN could streamline both clinical and research procedures in the volumetric assessment of MS disease burden as well as in lesion detection," first author Dr. Gianluca Brugnara and colleagues wrote in an article published online on 3 January in European Radiology.
Time-consuming, subjective reads
MS patients regularly receive MRI exams for assessing disease burden, which typically consists of qualitative visual assessment of the spatial and temporal dynamics of the lesion load. However, these exams are time-consuming to read and are prone to intra- and interobserver variability, according to the researchers.
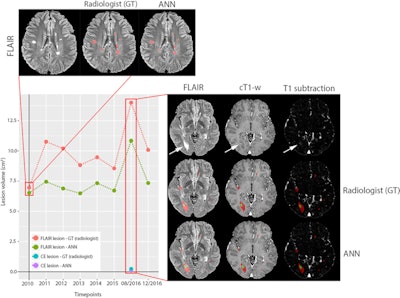
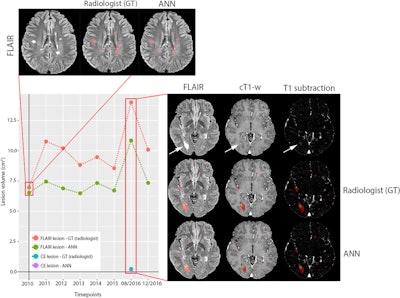
Example of a longitudinal comparison between radiologist-segmented MS lesions and ANN-segmented MS lesions, showing a good overlap between the two volumes. At the MRI follow-up of August 2016, the patient showed a large new lesion with a faint central contrast enhancement (both indicated by the arrows in FLAIR and T1 after contrast, respectively), which the ANN correctly detected. The lesion then regressed under therapy and was unremarkable at the next follow-up. While underestimating the volumetric size of the lesions, the ANN consistently reproduced the volumetric trend of the follow-up for this patient. Image courtesy of Drs. Gianluca Brugnara and Philipp Kickingereder and European Radiology.
To help, they sought to develop an ANN-based algorithm that could identify T2/fluid-attenuated inversion recovery (FLAIR) and contrast-enhancing lesions in MS patients. They then tested the algorithm in a patient population derived from clinical practice that was being longitudinally followed with MRI.
Their algorithm assesses disease burden by calculating the total number and volume of T2/FLAIR lesions and evaluates disease activity by the presence of contrast-enhancing lesions. To develop the model, they retrospectively gathered a dataset of 334 MS patients with 334 MRI exams to develop and train the algorithm to automatically identify and volumetrically segment T2/FLAIR hyperintense and contrast-enhancing lesions.
They tried out the algorithm on a dataset of 82 patients with 266 MRI exams. F1 scores were used to assess lesion detection performance, while Dice coefficients were utilized to measure lesion segmentation agreement in comparison with the radiologists on the original exams. Concordance correlation coefficients (CCC) were employed to evaluate lesion volume agreement.
Performance of AI algorithm for detecting, segmenting, and calculating the volume of MS lesions | ||
Training set | Test set | |
Mean F1 scores for T2/FLAIR lesions | 0.867 | 0.878 |
Mean F1 scores for contrast-enhancing lesions | 0.636 | 0.715 |
Dice coefficients for segmentation of T2/FLAIR lesions | 0.834 | 0.846 |
Dice coefficients for contrast-enhancing lesions | 0.878 | 0.908 |
Concordance correlation coefficients | ≥ 0.960 | ≥ 0.960 |
"Performance of the ANN was consistent in a clinically derived dataset, with patients presenting all possible disease stages in MRI scans acquired from standard clinical routine rather than with high-quality research sequences," the authors wrote.
Consistent, fast analysis
The researchers noted that, in contrast with a manual segmentation process, automated disease assessment is intrinsically consistent and fully reproducible, applying the same criteria for all exams without being influenced by intra- and/or interobserver variability.
"This has important implications, especially in the context of longitudinal patient follow-up, where small changes in disease burden might be missed due to this intrinsic human variability," the authors wrote. "The ANN, while maybe slightly over- or underestimating lesion volume, will do this consistently across the dataset, thus eliminating this possible bias. The ANN would thus be an important tool to aid reliability and standardization."
Furthermore, the ANN is much faster than manual assessment of MS lesions.
"Manual or semiautomatic segmentation of MS lesions is a very time-consuming and labor-intensive process whereas ANN can segment an entire case within a few seconds," they noted.
Brugnara and colleagues pointed out that unlike other studies, they did not only focus on T2/FLAIR lesions; contrast-enhancing lesions were also separately identified and segmented.
"This is important when it comes to the clinical applicability of ANN, since [contrast-enhancing] lesions are indicative of active disease and are also used to guide disease-modifying therapy decisions in MS," they stated.
They acknowledged, though, that recent investigations have suggested that almost all newly appearing contrast-enhancing lesions are also visible as new or enlarged lesions on FLAIR sequences.
"However, further confirmatory studies are required to determine the generalizability of these findings and several practical and methodical considerations remain to be addressed," the authors concluded. "Thus, for now, a truly generalizable algorithm that aims to perform automated assessment of disease burden in MS patients needs to be capable of identifying not only T2/FLAIR lesions but also [contrast-enhancing] lesions."