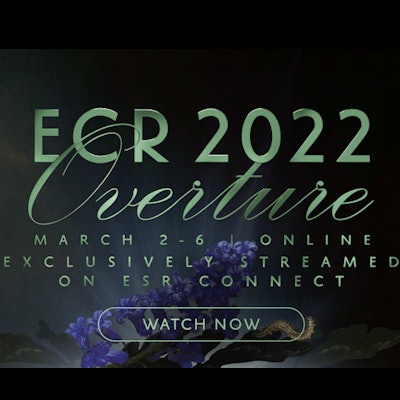
A European research project called Hypmed aims to develop a more cost-effective version of PET/MRI technology to better diagnose breast cancer. Dr. Christiane Kuhl and Volkmar Schulz, PhD, from Aachen, Germany, spoke about the initiative at the ECR Overture.
In a session on 3 March, the two researchers behind the Hypmed project provided updates on their PET/MRI device, which aims to make PET imaging available in conventional MRI scanners without the need for expensive upgrades.
"We set out to rewrite the history of hybrid PET/MR imaging," said Dr. Christiane Kuhl, professor and chair of radiology at University Hospital Aachen. "This device will be a proof of concept that will then also be transferable to other imaging areas, such as prostate imaging and cardiac imaging."
While technology and clinical practice have made progress over the years in detecting and diagnosing breast cancer early, the disease persists as one of the main causes of cancer death in women. Research has shown that diagnosing cancer early leads to better survival outcomes.
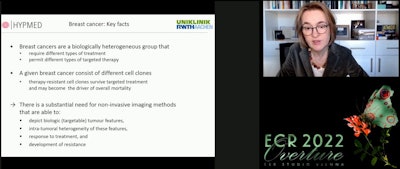
Current PET/MRI hybrid scanners use the same whole-body imaging concept that's commonplace in nuclear medicine. However, there are many applications for precision imaging in oncology that are targeted to specific areas of the body rather than general imaging.
Kuhl said that the whole-body approach used in commercially available PET and MRI scanners can limit their sensitivity, and thus limit their utility for targeted oncology applications.
"Because they pursue the traditional whole-body concept, state-of-the-art PET/MRI devices neither deliver optimal PET resolution and sensitivity, nor do they use the full potential of MR imaging," she said. "Moreover, whole-body hybrid PET/MRI systems are exceedingly cost-intensive."
That's what led researchers to lead the Hypmed project. Their goal was to develop a digital MRI-transparent PET detector into a multichannel MRI radiofrequency surface coil that doesn't interfere with the PET signal. The specialized coil can be used in a conventional clinical MR scanner.
“Our device is being constructed in a way that we have two moveable half rings that can allow us to access the breast,” said Volkmar Schulz, PhD, professor and head of the Department of Physics of Molecular Imaging (PMI) and Institute for Experimental Molecular Imaging (ExMI) at RWTH Aachen University.
This transforms conventional MRI scanners into hybrid PET/MRI systems that can identify cancer and track patient response to therapy, he said. The configuration is also much cheaper than incorporating a ring of PET detectors into a whole-body MRI scanner. The system also promises to clear the way for minimally invasive MRI and PET-guided targeted biopsy.
The future of Hypmed
So, what's needed before Hypmed can be used for clinical trials? Kuhl said the device needs to clear legal and regulatory hurdles before it can be used on patients.
"We have the device almost finalized, but we don't have a legal opportunity to use this brand new, promising device in clinical patients, not even in clinical volunteers," she said.
The team's goal is to have 100 patients participate in a clinical trial in which the Hypmed device will be compared with conventional whole-body PET/MRI. Information on histology, tumor grade, and molecular subtype among other characteristics will be taken for cohort matching. Data will initially be made available to Hypmed partners.
The Aachen group plans to present more data on Hypmed's developments at ECR 2022 in July.
Research in Vienna
During the same session at ECR Overture, Dr. Thomas Helbich, professor of radiology at the Medical University of Vienna, presented data from a protocol performed at the university involving multiparametric PET/MRI acquisition. The protocol was used in a 2021 study that Helbich was part of that showed promise for whole-body PET/MRI when used with artificial intelligence (AI).
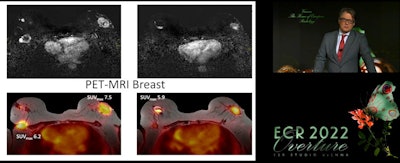
Here, the researchers found that the highest accuracy for breast cancer diagnosis using a regular PET/MRI system came from combining mean transit time for dynamic contrast-enhanced MRI and apparent diffusion coefficient maps with radiomics features extracted from PET and coefficient images, with an area under the curve of 0.983.
"In addition to that, we wanted to be able to assess the role of machine learning radiomics modeling for prediction of axillary lymph node status in breast cancer patients," Helbich said. "The combination of radiomics features alone is very valuable to predict lymph nodes, with an AUC close to 0.8."