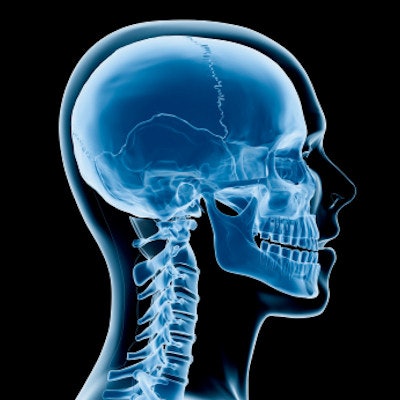
Deep-learning technology can be a useful tool for identifying cases that contain critical imaging findings, potentially expediting the interpretation of these exams and the communication of results, according to a presentation at the recent RSNA 2016 meeting in Chicago.
A team of researchers from Ohio State University developed a deep-learning model that achieved high accuracy in detecting critical findings on head CT studies. The algorithm could then flag the study as potentially critical and automatically rank it higher on the radiologists' worklist, while also paging the covering neuroradiologist for immediate review, according to the group.
"Based on our preliminary experience, we foresee that there's going to be a rapid adoption of this technology, with further expansion of this type of methodology in order to improve decisions and workflow in radiology in order to streamline the processes," said Dr. Luciano Prevedello, division chief of medical imaging informatics at Ohio State University Wexner Medical Center.
Identifying critical results
A major obstacle to communicating critical findings in busy radiology practices relates to the delay in recognizing which examinations contain a critical imaging finding; as many as 40% of inpatient imaging examinations could be designated as "stat" in the worklist, Prevedello said. Some exams that are not marked as stat may also have critical findings.
To address this problem, the researchers developed an automated artificial intelligence (AI) screening tool utilizing deep learning. Training and validation were performed on a dataset of 500 noncontrast head CT scans obtained between January and March 2015. The 500 cases included 250 consecutive cases with documented emergent intracranial findings: acute intracranial hemorrhage, intracranial mass effect, and hydrocephalus. In addition, the dataset included 250 consecutive studies with no emergent findings: normal studies, chronic microvascular disease, and chronic encephalomalacia.
The team did not include any exams that were performed for evaluation of stroke or with suspected cerebral infarct. Radiologists immediately review those cases following completion of the examination. Of the 500 cases, 376 (80%) were randomly assigned to a training set and 124 (20%) became the validation set.
The CT studies were generated on seven different scanner types from three vendors. The dataset included image slices with 4.8- and 5-mm thickness. Next, the cases were all processed with a deep-learning algorithm -- a convolutional neural network -- running on the Caffe deep-learning platform.
The test set of 80 cases included 40 consecutive CT studies with documented emergent intracranial findings and 40 consecutively acquired studies that contained no emergent findings between July and August 2015. For the purposes of the study, the gold standard was determined by the radiology report and review by the neuroradiologist.
Validation and testing
After being trained, the model produced 91% accuracy during validation for determining which exams contained emergent findings. In subsequent evaluation on the test set of 80 different cases, the algorithm yielded 95% sensitivity, 88% specificity, and 91% accuracy.
Performance of AI algorithm for emergent findings | ||
Cases with actual emergent findings | Cases with no emergent findings | |
AI finds emergent findings | 38 cases (true positives) | 5 cases (false positives) |
AI did not find emergent findings | 2 cases (false negatives) | 35 cases (true negatives) |
Both false-negative cases involved lesions smaller than 5 mm; the algorithm had 100% sensitivity for lesions larger than 5 mm. Prevedello noted that the training dataset included relatively thick slices.
It might be worth considering to "redo the study or re-evaluate with thin-section data to see if it would improve accuracy," he said.
Prevedello acknowledged the limitations of their work, including the use of seven scanner types. It's possible that a validation set including a new scanner type might not yield the same results. Also, they need to expand the case mix to include all possible diseases that could be found on noncontrast head CT, he said. They also haven't evaluated the performance of the model for individual findings.
Nonetheless, the researchers hope to implement their algorithm to screen head CT scans in the clinical setting and then notify radiologists when there is a positive finding, he said.