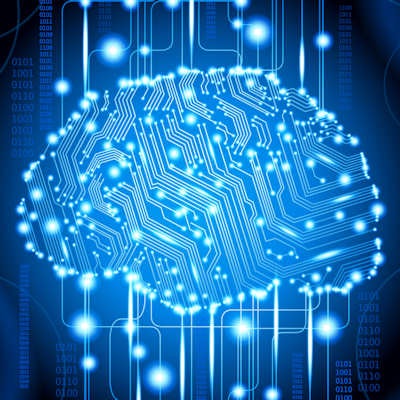
Can machine-learning techniques perform better than a radiologist in differentiating between malignant and thyroid nodules on ultrasound? It depends on the experience level of the radiologist, according to research published in the October issue of the American Journal of Roentgenology.
After developing and testing three machine-learning algorithms in a retrospective review of nearly 1,000 cases of thyroid nodules, a Chinese research team found that all three algorithms were more accurate than an inexperienced radiologist in differentiating between malignant and thyroid nodules on ultrasound. However, despite producing slightly higher sensitivity, the highest performing algorithm couldn't surpass the diagnostic performance of the experienced radiologist who provided the readings used to train the algorithms (AJR, October 2016, Vol. 207:4, pp. 859-864).
Such an algorithm could potentially facilitate decision-making and case education for inexperienced radiologists, according to the team led by Dr. Hongxun Wu of Jiangyuan Hospital Affiliated to Jiangsu Institute of Nuclear Medicine in Jiangsu, China.
Experience matters
Although thyroid nodules are very common, they are mostly benign. Several ultrasound features have been proposed as possible markers for malignancy, but no single feature is adequately sensitive or specific to identify all malignant nodules, according to the authors. As an added complication, ultrasound is subjective and operator-dependent; high inter- and intraobserver agreement for interpreting ultrasound findings related to thyroid nodules have only been achieved among experienced radiologists, they wrote.
As a result, the researchers sought to construct classifier models based on machine-learning algorithms and retrospectively assess their performance in differentiating between benign and malignant thyroid nodules on ultrasound. The study cohort included 970 histopathologically proven thyroid nodules in 970 patients seen between January 2012 and January 2014 at the Jiangsu Institute of Nuclear Medicine. Of the 970 nodules, 507 (52.3%) were malignant. The cancer cases included 487 papillary thyroid carcinomas, 12 follicular thyroid carcinomas, four medullary thyroid carcinomas, three well-differentiated carcinomas, and one clear-cell carcinoma.
A radiologist had performed thyroid ultrasound exams on these patients using an iU22 ultrasound scanner (Philips Healthcare) with a 5- to 12-MHz transducer. Static images were archived as JPEG image files for later evaluation.
Two radiologists -- one with 17 years of experience and one with three years of experience in thyroid ultrasound exams -- retrospectively read the studies and graded nodules according to a five-tier scoring system. The two radiologists were blinded to any subsequent cytologic or histologic diagnosis and the assessment by the performing radiologist. Based on the experienced radiologist's observations, the researchers obtained statistically significant variables from the cases and applied them as input nodes to build the classifier models for predicting nodule malignancy.
Three types of machine-learning algorithms were used: a naive Bayes classifier, the support vector machine, and the radial basis function neural network. After completing the training and testing process, the researchers compared the performances of the machine-learning algorithms and the radiologists for differentiating the thyroid nodules. Receiver operator characteristics (ROC) analysis was used to evaluate diagnostic performance.
The experienced radiologist produced the highest area under the curve, indicating the best diagnostic performance.
Performance for differentiating thyroid nodules on ultrasound | ||||
Sensitivity | Specificity | Accuracy | Area under the ROC curve | |
Experienced radiologist | 91.5% | 85.3% | 88.7% | 0.914 |
Inexperienced radiologist | 85.4% | 76% | 81% | 0.849 |
Naive Bayes classifier | 89.6% | 76% | 83.3% | 0.881 |
Support vector machine | 89.2% | 75.1% | 83.1% | 0.903 |
Radial basis function neural network | 92.3% | 76% | 84.7% | 0.910 |
The differences in area under the curve values between the experienced radiologist and the inexperienced radiologist and the three machine-learning techniques were statistically significant (p < 0.05). Notably, the radial basis function neural network had a statistically significant higher area under the curve than the inexperienced radiologist and the other two machine-learning algorithms (p < 0.05).
Differences in experience
The significant difference in diagnostic accuracy between the two radiologists may be attributable to variations in observer perception and interpretation on the basis of ultrasound features, according to the researchers.
"Inexperienced or nonspecialist radiologists may overestimate their knowledge and experience, making decisions on the basis of a limited number of conspicuous features," they wrote. "In addition, they may fail to consider all of the features systematically."
However, machine-learning algorithms have the capability to "consistently and comprehensively merge all of the variables and readily adjust in the context of noisy data," according to the group.
"Therefore, a classifier model based on a machine-learning algorithm could potentially facilitate decision-making and case education for inexperienced radiologists, which is consistent with the conclusion of a previous study [in the literature]," they wrote.
The researchers acknowledged a number of limitations to their study, including its use of a selected preoperative population with a relatively high malignancy rate. It also relied on a retrospective review of still images rather than using ultrasound images in real-time.
"Further studies are needed to evaluate the validity and interobserver consistency for the classifier models based on machine-learning algorithms," they wrote.
The researchers noted that they have begun preliminary work on building a visual interface for the algorithms.
"By inputting the ultrasound features given by an experienced radiologist, a malignancy risk estimation system for thyroid nodule based on the developed classifier model will provide a real-time calculation of the probability of malignancy, which will play a valuable role for management decision in clinical practice," they wrote.