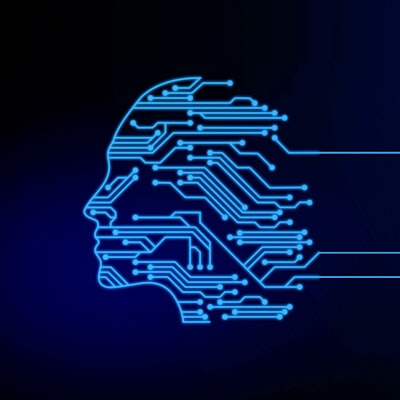
Can machine learning used with conebeam CT (CBCT) help clinicians to predict whether patients who have experienced stroke will have peripheral bleeding after they've undergone thrombectomy? Perhaps, Italian investigators say.
The ability to predict peripheral bleeding (i.e., hemorrhagic transformation) after endovascular thrombectomy is crucial, as the complication can be deadly, wrote a group led by Dr. Valerio Da Ros of the University Hospital of Rome in a study published on 25 October in Neuroradiology.
"Hemorrhagic transformation represents the most severe and dreaded complication of acute ischemic stroke and is an independent predictor of unfavorable outcome," the team noted.
Stroke patients typically undergo a CBCT exam immediately after thrombectomy, then have a noncontrast CT 24 hours later to detect any additional bleeding, guide an anticlotting regimen, and/or assess the final infarct volume.
The ability to predict with CBCT whether a patient will have peripheral bleeding after the procedure would improve patient outcomes, but previous research on this has been focused on evaluating the efficacy of visual assessment of the CBCT images -- which, since reader interpretation is subject to variability, could affect its predictive performance. That's where machine learning could help.
"The diagnostic challenge remains to differentiate abnormal post-EVT contrast staining (mimicking subarachnoid, intraventricular, or intracerebral hemorrhage) from actual intracranial hematomas," the group explained.
Da Ros and colleagues sought to explore whether machine learning with CBCT could help predict future bleeding via a study that included 43 stroke patients who underwent endovascular thrombectomy, then had an immediate postprocedural CBCT, and then, 24 hours after the thrombectomy, underwent a noncontrast CT.
Three radiologists analyzed both CBCT and noncontrast CT images, marking any regions of interest and categorizing them as hemorrhagic or nonhemorrhagic. The investigators then evaluated a machine-learning model (Bernoulli Naïve Bayes Classifier with MinMaxScaler) for its ability to improve CBCT's performance.
Of the 43 patients, 172 regions of interest were noted and were categorized as the following:
- 92 as "unremarkable"
- 5 as parenchymal contrast staining
- 29 as ischemia
- 7 as subarachnoid hemorrhages
- 39 as hemorrhagic transformation
The team found that using a machine-learning model with CBCT had a specificity of 75% for predicting risk of hemorrhagic transformation 24 hours after the procedure. What the team called the model's precision was lower, however, at 60%.
"Our study showed a roughly 60% precision for [hemorrhagic transformation] prediction," it noted. "Although our model was trained by operator-defined ROI, this performance significantly differs from the sensitivity, specificity, and accuracy rates (often over 90%) of visual CBCT assessment reported in the literature so far."
The study results suggest that more work needs to be done to refine the machine-learning model. But they also underscore its potential, according to the authors.
"Despite our preliminary data with machine learning being suboptimal [in its precision], this tool could be adequately implemented, and this novel standardized quantitative CBCT imaging analysis is warranted to overcome the inter-operator variability of interpretation, representing a further step towards a more precise and personalized medicine for our patients," they wrote.