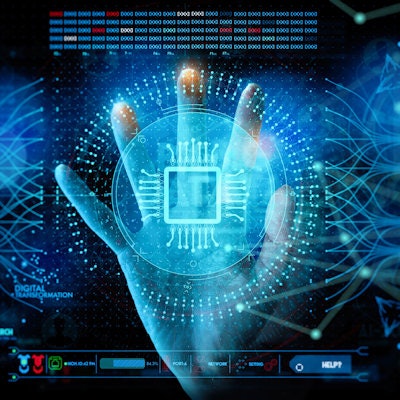
Artificial intelligence (AI) is poised to have an impact on the diagnosis of a number of chest imaging conditions, including chronic obstructive pulmonary disease (COPD), interstitial lung disease (ILD), and lung cancer, according to a session held on 3 March at ECR Overture.
In COPD, AI algorithms can automate repetitive image segmentation tasks and discover new biomarkers for COPD, according to Marleen de Bruijne, PhD, of Erasmus Medical Center in Rotterdam, the Netherlands, and the University of Copenhagen.
Machine-learning algorithms are available that can provide automated quantification of airways.
"So we do not yet segment every single branch that is visible in the images, but we do get quite complete detections and reconstructions of airway trees," de Bruijne said. "And once we have detected the airway trees, we are typically interested in airway diameters, lumen diameter, outer wall diameter, and wall thickness."
AI algorithms can also be useful for analyzing emphysema, which has most commonly been quantified using CT density values. This traditional approach of relying on CT density values can't discriminate between different types of emphysema, however, and is very sensitive to changes in inspiration level and to changes in scan protocol, according to de Bruijne. There's also a relatively low correlation between densitometry and visual assessment.
"But we can do better than this using AI," she noted.
AI can perform similarly to trained observers for detecting emphysema and differentiating severity, she said.
Imaging biomarkers
In addition, AI can be used to uncover new imaging biomarkers that might be important to describe emphysema or COPD, de Bruijne said. Machine-learning models can differentiate severity stages of COPD, as well as predict development of the disease better than densitometry.
"And we expect that the model would be even better at predicting future decrease in lung function if it was explicitly trained for that," she said.
AI can also be utilized to measure and quantify factors that may be important for COPD patients.
"For instance, we know that the pulmonary artery to aorta diameter ratio is an important predictor for COPD exacerbations and mortality in COPD patients," she said. "And this can be fully automatically quantified as well."
De Bruijne noted, though, that only a very small number of AI studies for pulmonology applications were published between 2005 and 2020, and most of these studies were not for COPD applications.
"But it is increasing and there are tools available," she said.
In clinical practice, AI can mostly contribute at the moment by providing anatomical measurements, according to de Bruijne.
"So [AI can be used to] perform a better quantitative assessment of the COPD patient and also improve the radiology workflow by incorporating such measures directly in the radiology report for radiologists to approve or adapt," she said.
Interstitial lung disease
AI can help in a number of applications for interstitial lung disease, including enabling better estimates of the extent of lung damage on CT exams, Dr. Joseph Jacob, a Wellcome Trust fellow at the University College London, said at the same ECR session.
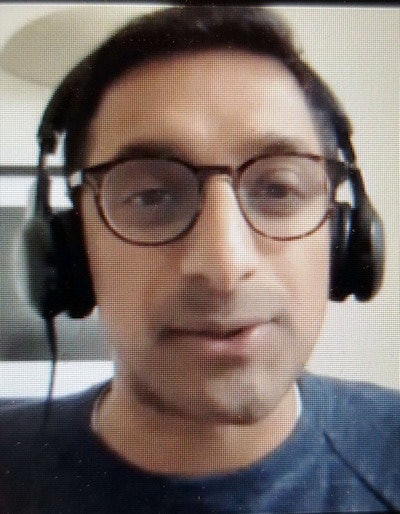
A number of software tools have been developed for quantitative analysis, including a software tool developed by the Mayo Clinic called Computer-Aided Lung Informatics for Pathology Evaluation and Rating (CALIPER).
Jacob also highlighted work at his institution in quantification of pleuroparenchymal fibroelastosis (PPFE) in idiopathic pulmonary fibrosis (IPF). His group found that their algorithm yielded higher sensitivity in detecting PPFE that indicated a poor prognosis in these patients.
"And interestingly, the PPFE that we identified does not correlate with other measures of lung fibrosis like [diffusing capacity of the lungs for carbon monoxide] extent or ILD extent, suggesting that this is a separate process complicating IPF patients that the computer has really been able to show us that we weren't able to see so clearly visually," he said.
In the future, earlier identification of ILD could be facilitated by lung cancer screening exams, according to Jacobs. Only 3% of lung cancer screening participants have lung cancer and 3% have established fibrosis, but 11% have features on their scan that may represent fibrosis that may develop in the future, he said.
"So by studying their progression, maybe we can identify those imaging patterns that are suggestive of progressive disease," he said. "And that might then allow us to develop a new radiology vocabulary to describe these interstitial lung abnormalities [that are] the precursor of lung fibrosis, instead of relying on reticulation, ground glass, and traction bronchiectasis as we have for the past 25 years."
Lung cancer
AI can be used in lung cancer imaging applications such as detection of nodules in a screening setting; diagnosis of cancer; classifying cancer subtype; and even predicting treatment response and survival, said Dr. Joon Beom Seo of the University of Ulsan College of Medicine. It can also be utilized to improve radiomics.
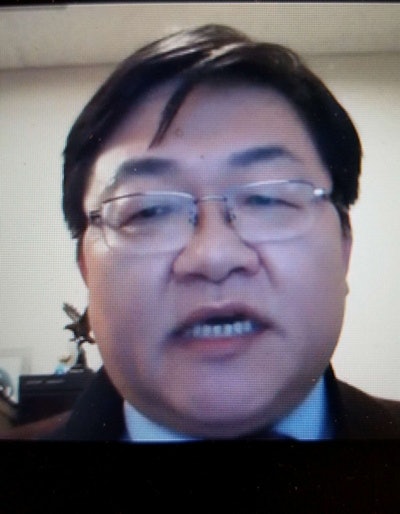
In the past, computer-aided detection (CAD) and computer-aided diagnosis (CADx) software was developed for detection and diagnosis of cancer, but clinical usage has been limited by a number of shortcomings, including an increased number of false-positive results, Seo said.
However, deep-learning algorithms have been developed that have been shown in testing to perform well in detecting nodules on low-dose CT lung cancer screening exams, according to Seo. In addition, his group has developed an algorithm for detecting subsolid nodules.
As of March 1, 17 commercial software applications have received either the CE Mark or U.S. Food and Drug Administration (FDA) clearance, according to Seo.
"But the real performance of each software in clinical settings is not determined," he said.
As only 3.6% of nodules are actually lung cancer, it's important to determine the risk of malignancy of all nodules detected on CT, Seo noted. A number of researchers have developed AI algorithms to classify nodules by subtype. Another study by Seo's group found that deep-learning software can accurately measure the solid portion of lung cancer.
Furthermore, AI technology can improve inter-reader agreement for LUNG-RADS categorization of nodules, as well as assist in estimating nodule malignancy risk, he said. Other applications include predicting disease-free survival from preoperative CT, assessing prognosis of resectable lung adenocarcinoma, differentiating invasive adenocarcinoma from preliminary invasive lesions, combining a machine-learning algorithm with radiomics to predict immunotherapy response, and detecting important mutations in lung adenocarcinoma. AI can also improve the reproducibility of radiomics, he said.
"AI can be used in almost every step of imaging practice in lung cancer imaging, and although the commercially available software are limited only to the detection and characterization of nodules, I'm quite sure that this [other] research work will be implemented to the clinical practice to be used," Seo said. "So surely AI can enhance our clinical workflow for cancer management in the future."
The future
Looking ahead 10 years, session chairperson Dr. Jürgen Biederer of Heidelberg University expects that thoracic imaging will deliver microscopic-detail resolution and provide morpho-functional imaging.
In addition, "biomarkers will replace 'findings' and radiomics will increase our diagnostic scope," Biederer said. "And AI is expected to help us at all levels."
But will AI replace radiologists?
"Not at all," he said. "Prepare for an exciting journey and just make sure you don't miss the boat."