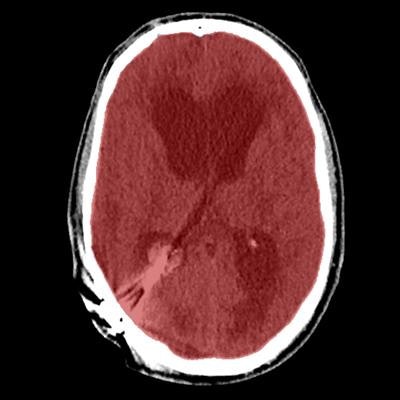
Using automated software to interpret head CT scans could someday slash the time emergency doctors need to start clot-busting treatments in cases of suspected ischemic stroke, according to Dutch researchers.
In their study, the researchers developed a way to use software to automatically segment the cranial cavity based on anatomy borrowed from multiple image atlases, then refined the segmentation based on the patient's head CT data. The results closely correlated with manual segmentation of the cranial cavity in CT studies, validating the approach in a database of almost 600 patients.
"Our study will thus serve as a first step towards automated evaluation and interpretation of cranial CT exams," wrote Ajay Patel, a PhD candidate, and Rashindra Manniesing, PhD, from the diagnostic image analysis group of Radboud University Medical Center (SPIE Newsroom, September 22, 2016).
The results "highlight what we can achieve with respect to automated segmentation in cranial CT images," Patel added in an email to AuntMinnie.com.
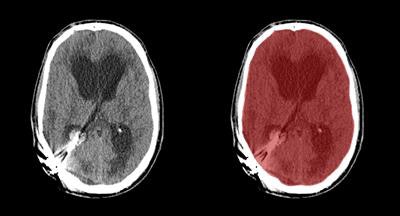
One size doesn't fit all
Cranial CT exams in everyday clinical practice contain large variations in anatomy and pathology, while the same features across different samples tend to look alike. This makes the cerebral cavity a good target for automated segmentation, especially in stroke care where "time is brain," and manual methods are slow and tedious.
Most acute strokes are ischemic in origin, indicating disruption to cerebral blood flow, while others are hemorrhagic, caused by vessel or aneurysm rupture.
Obviously, the conditions require very different approaches. Ischemic stroke treatment consists of administering thrombolytic drugs to prevent soft-tissue damage and loss of brain function within 4.5 hours after symptom onset -- but thrombolysis can have disastrous consequences if applied to patients with hemorrhagic strokes.
"A fast neuroimaging-based patient triage is therefore required for appropriate treatment selection," Patel and Manniesing wrote.
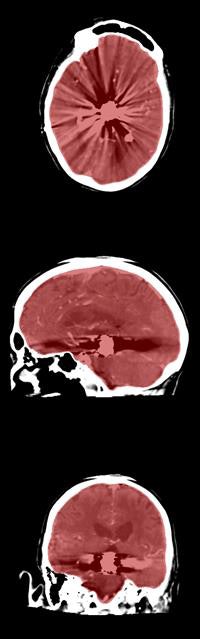
In the time-critical environment of stroke care, CT is favored over MRI owing to its wide availability, comparatively low cost, and high speed, according to the authors. In 4D CT perfusion, a contrast agent is used to facilitate the visualization of cerebral hemodynamics over time. CT perfusion yields several parameters for stroke assessment, including cerebral blood flow and volume, which offer information about the extent and severity of infarcted tissue.
Blood flow and volume measurements can also distinguish between permanently damaged tissue and tissue that may be salvageable by reperfusion therapy. To date, however, there has been little progress in the development of algorithms for the automated quantification and analysis of cranial CT exams in acute stroke, they wrote.
Atlases of priors
The researchers sought to encompass the wide variety of cranial anatomy observed in clinical practice through the creation of an atlas image database. Based on anatomical similarity to the patient, the most appropriate atlas images are selected and used by the algorithm to obtain a segmentation of the cranial cavity.
The data in the atlas define the anatomy in the cranial cavity, thereby enabling an early approximation of the patient's cranial cavity via image registration, the authors explained. After initial segmentation, the details are refined using the sharp natural gradient between the intracranial tissue and the skull.
Prior images in the atlas are registered to the patient image using a two-staged approach, Patel said. The first is an affine registration for a coarse alignment of the images, followed by a second, nonlinear registration to deform the atlas labels to fit the patient image. The method described is used to obtain a segmentation of the entire cranial cavity and does not differentiate between healthy or ischemic regions within the brain parenchyma. The segmentation serves as an initial step in the development of algorithms to detect and quantify anatomical regions and pathology within the cavity.
To test the algorithm, the researchers used a set of 573 acute stroke and trauma patients who had undergone CT or CT perfusion at the hospital. Exclusion criteria were limited so the data would conform to everyday practice as much as possible. For example, images that revealed metal artifacts and gross pathology such as tumors or large hemorrhages were not excluded from analysis.
The atlas images in the database were all registered to a CT template image beforehand, a process that aligns all of the images in a common space, Patel told AuntMinnie.com.
"An incoming patient image is quickly aligned to the same space," he said. "This allows us to compare the patient to all atlas images in our database without having to register them to each other one by one," he explained.
Labels for the registered atlas are then fused to create an initial approximation of the patient's cranial cavity, which is used to create a multistage geodesic active contour levelset method, where levelset represents an evolving surface steered by image information.
The gradient at the inner edge of the skull served as a boundary to stop propagation of the segmentation process. The first stage of refinement enables correction of errors that might be introduced during image registration while also allowing fast propagation of the initial segmentation. The second stage refines segmentation along the inner boundary of the skull.
The automated results were compared with the manual annotations of trained observers, which were used as a reference standard.
Fast, equivalent to manual segmentation
The multiatlas registration results showed a high degree of overlap with the reference standard, yielding a mean Dice similarity coefficient of 0.973 ± 0.035, and adding levelset refinement increased the value to 0.984 ± 0.026.
The results encompass a large spectrum of anatomical and pathological variations seen in everyday clinical practice, and they closely approach the high performance of expert manual annotation, the authors wrote.
"Segmentation of the cranial cavity in CT images may be overlooked as a problem in medical image analysis or seen as a trivial task," Patel said. "However, it is a fundamental step in the development of algorithms for automated evaluation and analysis of cranial CT exams. We demonstrate a robust and fully automated method that has been extensively evaluated and achieves an accuracy close to that of a human observer."
Development of the segmentation algorithm "forms the crucial basis for the development of future algorithms to discern ischemic from hemorrhagic stroke," Patel continued in his email. "I firmly believe we will be able to do so in the coming years."
The next step in the research will be to use the segmented data to distinguish ischemic from hemorrhagic stroke to determine the course of care.
Cranial cavity segmentation "facilitates the development of computer-aided detection systems for intracerebral hemorrhages -- and their presence is an important contraindication for thrombolytic treatment in acute stroke," Patel said. "Our current results show ... an accuracy close to that of time-consuming human annotation."
More detailed results will be published soon, he said.