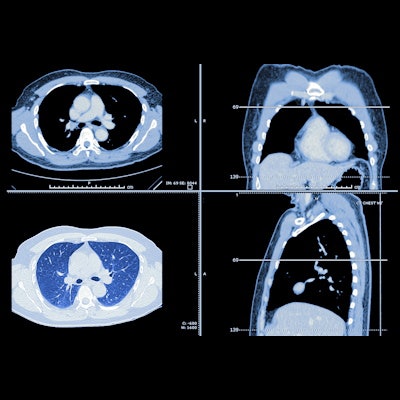
VIENNA - Artificial intelligence (AI)-based software can help radiologists detect more lung nodules on CT, regardless of whether they use the software concurrently during interpretation or afterward as a second reader, according to research presented 15 July at ECR 2022.
Researchers from Siemens Healthineers, the Cleveland Clinic, and the University of California, Los Angeles performed a multireader, multicenter study to assess the effect of lung nodule computer-aided detection (CAD) software on radiologists' diagnostic accuracy when used in two different workflows. They found that both methods yielded beneficial performance improvements.
"Using AI-based CAD as a concurrent first reader and as a second reader both significantly improve the readers' sensitivity and accuracy in detecting all kinds of nodules and only with a small price, which is the small increase in false positives per case," said presenter Yiyuan Zhao, PhD, of Siemens Medical Solutions USA.
In their study, the researchers recruited 20 radiologists to independently read 232 chest CTs collected from multiple institutions. Of these cases, 143 were positive and 89 were negative for lung nodules.
All cases were read twice by each of the radiologists after a one-month period, randomly reading the cases with AI as either a concurrent reader or as a second reader after their initial interpretation. The radiologists were asked to identify solid, part-solid, and ground-glass nodules.
Later, these consolidated image marks were reviewed independently by five expert thoracic radiologists. These assessments were then adjudicated by another expert thoracic radiologist to determine the reference standard for the study.
The software (Siemens) was based on two AI networks: a cascaded-faster residual convolutional neural network for identifying suspicious regions on the images and a ResNet neural network for performing further classification of these regions, according to Zhao. After the radiologists finished reading the cases twice, the researchers assessed the impact of AI on detection of solid (3-30 mm), part-solid (5-30 mm), and ground glass nodules (5-30 mm).
Improved average radiologist performance for lung nodule detection from AI | |||||
Unaided radiologists | Radiologists with concurrent use of AI | Radiologists with AI as a second reader | |||
Area under the curve (AUC) (lobe level) | 0.76 | 0.809 | 0.811 | ||
Sensitivity (nodule level) | 43% | 92.2% | 91.5% | ||
Specificity (lobe level) | 94.2% | 51.9% | 53.7% | ||
False positives per case | 0.44 | 0.55 | 0.65 |
The improvements in AUC and sensitivity were statistically significant (p < 0.001) for both reading paradigms.
When reviewing the performance of each radiologist, the researchers found that concurrent use of CAD improved nodule-level sensitivity by as high as 20% with only an average 1.6% decrease in lobe-level specificity. Similarly, using CAD as a second reader increased nodule-level sensitivity up by 20.5% with only an average drop in lobe-level specificity of 2.3%.
"Using CAD can give benefits to radiologists during reviewing CTs for nodule detection, and this increase can be greatly helpful in early cancer detection due to increased number of nodules being detected and of course the reduction in chances for missing malignant nodules," Zhao said.
He noted that the use of AI as a concurrent reader will not increase radiologist reading time, and could in fact, improve their efficiency as the software points out suspicious nodule areas for review.