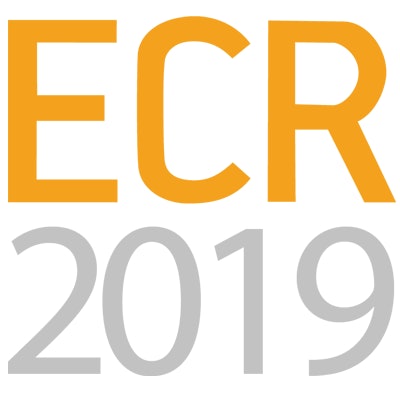
VIENNA - An artificial intelligence (AI) algorithm can provide real-time, patient-specific radiation dose estimates on single- or dual-source CT studies at an accuracy approaching the level of Monte Carlo simulations, according to a presentation on Wednesday at ECR 2019.
Researchers from Germany trained a deep-learning algorithm to provide radiation dose estimates based on a CT volume and a first-order dose estimate volume. In testing, their method -- called deep dose estimation -- yielded estimates that deviated by an average of less than 5% from the current gold standard: Monte Carlo simulation. It also took less than a second to perform on a 256 x 256 x 48 voxel volume and would require five seconds to process a whole-body CT exam, according to presenter and senior author Dr. Marc Kachelriess, PhD, of the German Cancer Research Center (DKFZ) and Heidelberg University in Heidelberg, Germany.
"So you could actually use the deep dose estimation for a real-time dose estimation for the patient," Kachelriess said. "Combined with the segmentation of the organs, you could give organ-specific doses and effective doses if you like."
He presented the research on behalf of first author Dr. Joscha Maier.
Dose assessment methods
Dose assessment is an important issue in CT imaging for optimizing imaging or for calculating patient dose. However, common metrics such as CT dose index weighted (CTDIw), CT dose index volume (CTDIvol), dose-length product (DLP), k-factor, and size-specific dose estimates have limitations for providing dose information on an organ- or patient-specific basis. Both Monte Carlo simulation of the CT acquisition and another method -- an analytic linear Boltzmann transport equation solver -- are accurate but computationally expensive and not suitable for real-time use, Kachelriess said.
On the other hand, other dose-calculation alternatives -- such as applying patient-specific conversion factors to the DLP, applying lookup tables using Monte Carlo simulation of phantoms, or performing analytic approximation of CT dose deposition -- are faster but less accurate than the Monte Carlo estimates, Kachelriess said.
Deep learning
Therefore, the German researchers sought to make use of a deep convolutional neural network to achieve both fast and accurate CT dose estimation. They trained the network to reproduce Monte Carlo dose estimation by providing it with a CT volume and a first-order dose estimate volume. This dose estimate, which encodes the tube current, tube voltage, shaped filters, etc., is calculated analytically using back projection to sum up the contributions of the photo effect, Compton scattering, and energy deposition in each voxel of the image, according to the researchers.
As a result, the first-order estimate provides an image displaying the main characteristics of the dose distribution, Kachelriess said.
For training and validating the deep-learning model, the researchers simulated 1,440 circular dual-source CT scans of the thorax, abdomen, and pelvis using 12 different patients. Simulation was performed with and without a bowtie. Image reconstruction was performed on a 512 x 512 x 96 grid with 1-mm voxel size, followed by 2 x 2 x 2 binning to reduce the number of voxels for dose estimation.
Of the 12 patients, nine were used for training and three were used for testing. The network was trained for 300 epochs, and the same weights and biases were used for all cases, Kachelriess said.
The method approached similar accuracy in deriving dose estimates -- with an average deviation of 4.6% -- when compared with the Monte Carlo dose estimates, the researchers found. The model was also much faster.
Processing time for estimating patient dose | ||
Monte Carlo simulation | Deep-learning model | |
48 slices | 1 hour | 0.25 seconds |
Whole-body CT exam | 20 hours | 5 seconds |
Kachelriess said their model generalizes to different anatomical regions and is able to handle different fluence fields. He also noted that the study was restricted to training data with a CT scan volume of about 10 cm.
"So we did not train [with] the full-body CT scan, which is basically just some additional work," he said. "But we are pretty confident that it will show the same results."