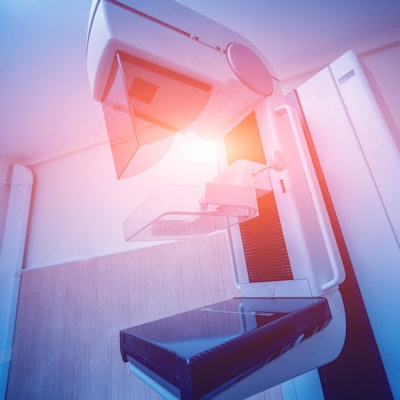
An AI model predicts breast cancer risk after a negative screening, according to new research published on 13 June in Radiology.
A team led by Celeste Damiani, PhD, from the Italian Institute of Technology in Genoa found that the model predicted breast cancer risk with higher performance than breast density measures. The group also found that the model had similar performance for invasive cancer and ductal carcinoma in situ (DCIS), as well as higher performance for advanced cancer risk and screen-detected estrogen receptor-positive cancer.
"These results suggest the model might have a role in the development of risk-based screening algorithms," Damiani and colleagues wrote.
Mammographic breast density is one of the largest predictors of future breast cancer risk, as dense tissue can create a masking effect on conventional mammography. Damiani and colleagues hypothesized that additional mammographic information beyond breast density -- computer-aided detection (CAD) suspicion scores, for instance -- could help better assess breast cancer risk.
An AI model developed by researchers at the Massachusetts Institute of Technology called Mirai has previously shown to be successful in predicting breast cancer risk over a five-year period. The team investigated Mirai's performance for assessing risk for future breast cancer based on digital mammograms and explored the possible heterogeneity of performance for interval versus screen-detected cancers by accounting for age group and cancer type.
The study consisted of data from the U.K.'s National Health Service (NHS) Breast Screening Program which included 2,044 women with screen-detected cancer (1,528 had invasive cancer and 503 had DCIS). The investigators also included interval breast cancer data from 696 women (636 with invasive cancer, 54 with DCIS).
They found that the AI model had a higher likelihood of predicting a future breast cancer than one that used breast density data alone, with an area under the curve (AUC) of 0.67 for the model compared with 0.56 for breast density (p < 0.001). While breast density improved the model's performance for interval cancer prediction from an AUC of 0.69 to 0.71 (p < 0.001), this was not the case for screen-detected cancers.
The AI model had an overall AUC of 0.68. There was no significant difference between interval (AUC, 0.69) and screen-detected cancer (AUC, 0.67; p = 0.085). The team also found similar performances from the model for detecting invasive cancer versus DCIS, with AUCs of 0.68 and 0.66, respectively (p = 0.057).
Despite their results, the study authors urged future studies to explain the model's internal workings and how mammographic features contribute to its performance. Further testing is needed in retrospective and prospective studies to compare the model with other tools, particularly those for digital breast tomosynthesis (DBT), they wrote.
In an accompanying editorial, Ritse Mann, MD, PhD, from Radboud University Medical Center in the Netherlands and Ioannis Sechopoulos, PhD, from the University of Twente in the Netherlands wrote that this kind of image-based risk modeling may act as a precursor to determining optimal screening pathways.
"It is probable that the detection of early signs of breast cancer explains why image-based risk estimation performs so much better than the classic risk estimators that only focus on factors outside the breasts," they wrote.