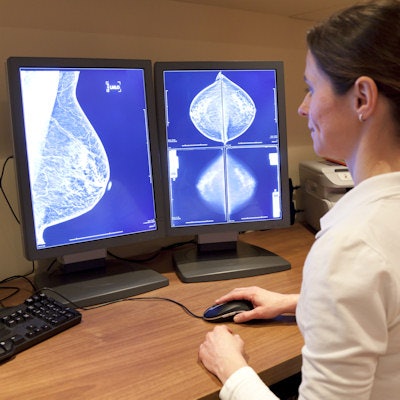
A breast cancer screening workflow that leverages the different strengths of radiologists and artificial intelligence (AI) software can improve overall accuracy and reduce workload, according to research published on 22 June in Lancet Digital Health.
In a study involving nearly 1.2 million digital mammograms produced at eight breast cancer screening sites in Germany, researchers from German AI software developer Vara, Memorial Sloan Kettering Cancer Center (MSKCC), and Essen University Hospital in Germany retrospectively assessed the performance of an AI software application, both on a standalone basis and when used in what they call a two-step "decision-referral" model. In this workflow, the AI software initially serves as a triage tool for highly likely normal cases and generates structured reports for these exams. For other cases, the software served as an as-needed interpretative aid for radiologists during their review.
Although the AI software didn't perform as well on a standalone basis when compared with the radiologists, the decision-referral approach led to significantly higher sensitivity and specificity than either the standalone AI results or the unaided radiologists. Workflow could also be safely reduced by as much as 70%, according to the researchers.
"This approach has potential to improve screening accuracy of radiologists, is adaptive to the (heterogeneous) requirements of screening and could allow for the reduction of workload through triaging normal studies, without discarding the final oversight of the radiologists," wrote the authors led by Christian Leibig, PhD, of Vara.
The researchers evaluated the AI algorithm and screening strategy on a dataset of 1,193,197 full-field digital mammograms performed between January 1, 2007, and December 31, 2020, at eight screening sites participating in the German national breast-cancer screening program. They then created an internal test dataset of 1,670 screening-detected cancers and 19,997 normal mammography exams from six sites, as well as an external test dataset from two other sites that included 2,793 screening-detected cancers and 80,058 normal exams.
Improved breast cancer screening performance on test set from combination of AI and radiologist review | |||
Standalone AI software (at exemplary configuration) | Average unaided radiologist | Decision-referral approach (AI triage of normal cases and assistance during radiologist review) | |
Sensitivity | 84.6% | 87.2% | 89.8% |
Specificity | 91.3% | 93.4% | 94.4% |
Sixty-three percent of the exams in the external dataset could have been triaged as normal based on the AI analysis. On the subset of studies assessed by AI, the algorithm produced an area under the curve of 0.982, exceeding the performance of the unaided radiologists.
Notably, the decision-referral approach also yielded significant increases in sensitivity for a number of clinically relevant subgroups, including subgroups of small lesion sizes and invasive carcinomas, according to the researchers.
"If the algorithm performs more accurately on one subset of studies and the radiologist is better on the other, each can perform predictions in which they excel," the authors wrote.
This workflow strategy would also enable screening programs to iteratively work towards automating more screening decisions within a safe work, rather than converting to a fully automated AI system without human oversight, according to the researchers.
"This study demonstrates and underlines that AI is not meant to replace human radiologists but can assist us to improve our diagnoses and in the long run, improve patient care," said Dr. Lale Umutlu of Essen University Hospital in a statement from Vara.
Co-author Dr. Katja Pinker-Domenig of MSKCC noted that two or more readers are always better when it comes to reading mammograms.
"It's both common and encouraged that radiologists seek counsel from their colleagues and the decision-referral approach is simply an extension of this," Pinker-Domenig said in the statement from Vara. "We are encouraged to see that this study helps to show this method can improve results."