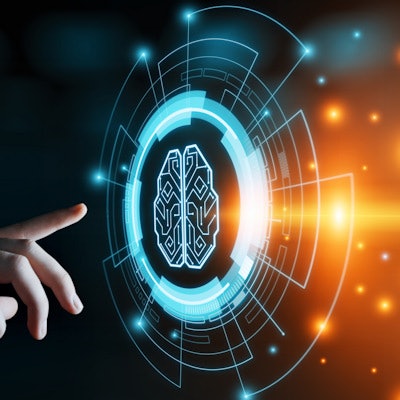
Integrating radiology artificial intelligence (AI) technology into clinical practice changes the workflow, and successful implementation requires a thoughtful plan and the involvement of many stakeholders, according to a recent webinar held by the European Society of Medical Imaging Informatics (EuSoMII).
In a wide-ranging talk, Dr. Erik Ranschaert, PhD, the president of EuSoMII, reviewed the key workflow issues associated with radiology AI and offered recommendations for how healthcare institutions can adopt radiology AI tools.
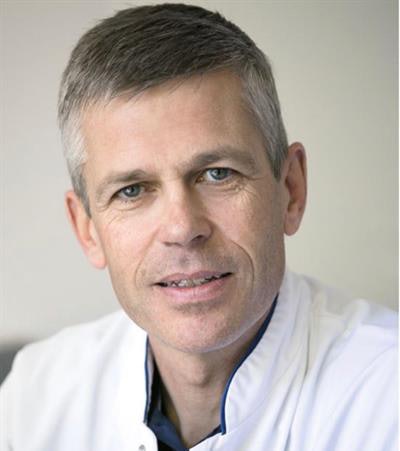
It's important first to decide what you want to accomplish with radiology AI. Currently, most attention is being paid to automated image analysis tasks. However, there are many other possible applications for AI that can yield improved patient care and safety, Ranschaert said.
"It's essential to start with defining the most relevant clinical use cases for the implementation of AI in your workflow of daily practice," he explained.
After determining what type of AI application to pursue, a road map should be created. This process should include identifying the decision-makers in purchasing AI and identifying and engaging with all stakeholders. A local champion should also be found who strongly believes in the project and can spend time implementing it, said Ranschaert, who is a radiologist at Elisabeth-TweeSteden Hospital (ETZ) in Tilburg, the Netherlands.
Next, learn about the existing policies and regulations and define the IT infrastructure that you have or need, depending on the targets.
"For example, do you want to implement existing commercial tools from vendors, or do you want to develop AI tools yourself?" he said. "This has an impact on how you build your infrastructure. As you know, everything needs to be seamlessly integrated, otherwise nobody will use it."
Then find out about the current priorities of the information and communications technology department to see when they would be available to help with the project, Ranschaert said.
"You have to talk with them and find the plan to implement this, because you will absolutely need them," he noted. "So allow plenty of time."
Road-map elements
It's a good idea to have a stakeholder advisory committee or some type of governance structure, he said. There should be regular communication with all participants.
Also, identify key performance indicators for what you want to achieve with the AI application. A multistage review of the AI tool before, during, and after clinical implementation would be helpful. After each review stage, adaptations and improvements can be made based on feedback, Ranschaert added.
Budgetary issues are also a factor, as calculating a return on investment can be difficult. To justify the cost of the technology, it's perhaps best to focus on benefits relating to faster turnaround time; improved efficiency, accuracy, and patient safety; strategic positioning of the hospital; satisfaction of referring physicians and patients; and higher capacity in the radiology department.
"There are many factors that you have to think about, and the more you have the better it will be to convince the stakeholders to make this investment," he said.
AI can be implemented by purchasing a vendor offering, developing the technology in-house, pursuing a partnership with academic institutions to co-develop algorithms, or a combination of these options, according to Ranschaert.
Integrating AI
As most AI use cases will help the radiologist analyze images and add relevant findings to the report, it's important to determine at what stage you want the AI tool to become active. It's possible that the AI application could even bypass the radiologist or resident to produce a preliminary report, such as in cases of normal chest x-rays or providing a stroke report directly to the neurologist or interventional radiologist via a mobile device.
There are three possible scenarios for how AI can be utilized: AI on demand, automated image analysis, or discrepancy management, according to Ranschaert.
In the AI-on-demand model, the radiologist decides when to ask for AI interpretations of a single image or a series of images. These images are then sent from the PACS to the AI server, which can be in the cloud. The AI results are then sent back to the RIS and the electronic health record (EHR). The big advantage is that radiologists retain full control of asking for AI interpretations, but it requires a manual step and is more time-consuming and could result in delays in the workflow, he noted.
The second approach -- automated AI image analysis -- could be the most preferred option, Ranschaert said. Before exams are read by radiologists, they are automatically sent in the background from the imaging modality to the AI server, and the AI results are then sent on to the PACS. This method can help prioritize the reading order, therefore reducing turnaround time. If they wish to, radiologists can view the AI findings during interpretation and ensure accuracy and revise the AI report, if necessary, before it's sent to the EHR.
The third scenario -- the discrepancy management option -- follows a similar workflow as the automated image analysis model; however, instead, it automatically routes AI results to the RIS/EHR. These results could be considered a preliminary report, which can then be reviewed by the radiologist to make a final report.
Of course, this method would require an extremely accurate AI algorithm that's highly sensitive and specific. It would offer the fastest turnaround time but also the potential risk of errors, which can be noticed by the referring physician before the radiologist, according to Ranschaert.
"It might also increase calls to the radiology reading room regarding the interpretation and might possibly delay the workflow," he said. "It might also have medicolegal consequences."
5 recommendations
Ranschaert offered five key recommendations for integrating AI into your workflow:
- Have a local champion
- Find a relevant use case
- Make a road map
- Include all relevant stakeholders in the planning, testing, and implementation phase
- Define methods and metrics to evaluate the AI application
Ranschaert noted that the technology must perform at least as well as the existing solution, and it must deliver measurable improvements in diagnostics, workflow, and costs.
"AI is a way to reach something; it's not a goal by itself," he said.