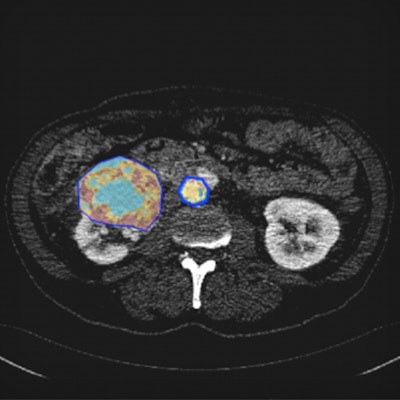
Being both data-driven and a vital element of discovery research, radiomics is poised to be a pivotal process for the development of new diagnostic imaging strategies and therapies, delegates learned at the French national radiology congress (Journées Francophones de Radiologie Diagnostique et Interventionnelle, JFR 2018), held in Paris from 12 to 15 October.
Rapid progress is being made, but as those in the field of radiomics seek to stratify which imaging parameters are useful for answering certain questions, they are facing a number of challenges, according to Dr. Laure Fournier, PhD, professor of radiology at Hôpital Européen Georges Pompidou in Paris.
"Traditionally, physicians were suspicious of data-driven research, but because of recent progress making its statistics sturdier, it is happening in all areas, including medicine, and imaging has opened up to it. There has been a shift in paradigm from mistrust to interest, particularly in its potential in discovery research," she told AuntMinnieEurope.com ahead of her JFR 2018 presentation on Saturday morning in the session AI for Idiots.
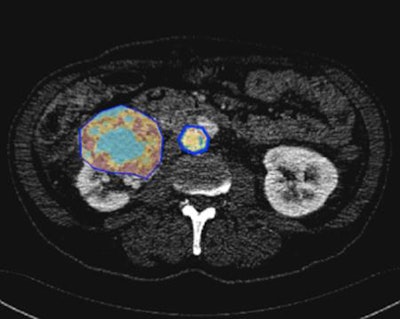
"Radiomics is increasing efficiency in research using high throughput techniques and that's why we should do it. But we must understand the physics of it," she noted.
In theory, radiomics uses big developments in data science technology and understanding to better sift through parameters to find the promising ones, and make research more efficient. For example, doctors interested in survival as an outcome, or in tumor response to specific therapies, need to understand which imaging parameters are associated with them.
Fournier explained, however, that looking at parameters often generates a black box effect, whereby radiologists don't understand what they are seeing, or the physical significance of it. The radiological image is merely a representation of a physical wavelength and its interaction with tissue, and there are a lot of technical elements during the processing of that image that will influence the imaging parameters.
She pointed to the texture parameters as a key example. These parameters reveal the heterogeneity of an image, yielding additional information that can't be seen with the naked eye. This quantification takes place at pixel level but radiologists need to understand what influences this quantification. Furthermore, the parameter might be used in a predictive manner, which helps doctors to make a diagnosis or select appropriate therapy. However, if the CT scanner or protocol changes, then will the parameter still be useful?
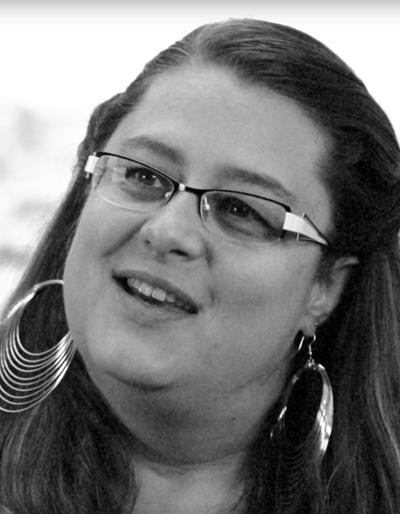
"You have to understand how a parameter is produced and what the measurement means. If not, this will lead to errors that will impact diagnosis and therapy," Fournier noted.
The solution is that radiomics researchers can't stop at parameter discovery. She illustrated the point through an example from her own radiology department. The group has discovered an interesting parameter (elongation) for the evaluation of HPV infection in head and neck cancers. Now the department's radiologists are working with the mathematicians and physicists to see what it means and to understand its sensitivity to different technical acquisition techniques. The team is also testing different calculation techniques to make the parameter more robust.
Like artificial intelligence (AI), radiomics is based on data-driven research but whereas AI is a tool, radiomics is a process that one can partly control, according to Fournier. If one can make mistakes in radiomics due to acquisition technique sensitivity, this is even more true in AI but in the latter, there seems to be less focus on this factor, she said.
"Another difficulty encountered in radiomics and AI is the problem of validation in data-driven research: There is not enough data and what exists is too similar. For example, when teaching algorithms you have to cover every single possibility or the algorithms might not recognize the exceptional situations and deal with them correctly when they crop up," Fournier pointed out. "No one knows how many patients it takes to train an algorithm whether for the purpose of radiomics or AI, but with radiomics one can vaguely perceive the issues of a lack of diversity, while in AI it is less apparent."
As well as improving research efficiency through traditional use, radiomics can open the door to new unsupervised discoveries. For example, instead of having a predetermined question, and looking for associated parameters, one can simply feed information using a radiomics program and explore if there are different groups of patients that emerge from the data.
This clustering can be represented visually by heatmaps that color-code the number values of a parameter to yield a synthetic view of massive data that is understandable to the naked eye.
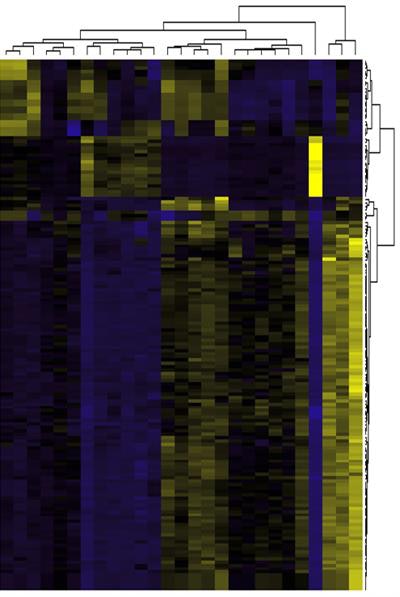
"This unsupervised use could lead us to understanding there are different patient profiles that previously have not been identified," Fournier said. "For example, if we notice that some patients don't respond to therapy and this imaging profile never responds, we can seek to understand the biological differences expressed in the image as to why they don't respond, and this helps to stratify different patient subgroups to different treatments."
Therefore radiomics acts as an important source of new medical discovery, she continued. Discerning new biological profiles will lead to the development of new therapies and drugs.
"Instead of imaging being downstream of drug development, it will be upstream," she said.
One challenge is this new field does not benefit yet from standardization. Each research group has their own software and parameters. Fournier pointed to one group of physicists that has made strides in this area with its Imaging Biomarker Standardization Initiative (IBSI); a pdf of standardized guidelines including recommendations and vocabulary so that results published in the field can be reproducible. IBSI is free and updated regularly, but is not yet peer reviewed.
Once issues of standardization are resolved, Fournier is positive about the future of radiomics' role in harnessing big imaging data and mining it in the coming years.
"Radiomics will allow new discoveries and understanding of images and what they can contribute to patient care," she said.