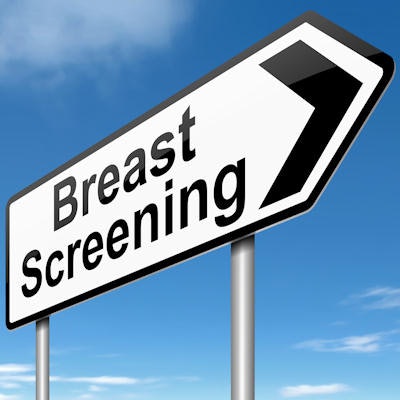
How does an artificial intelligence (AI) algorithm compare to radiologists when it comes to interpreting screening mammography exams? Their performances were fairly comparable, according to research presented at the recent RSNA 2017 meeting in Chicago.
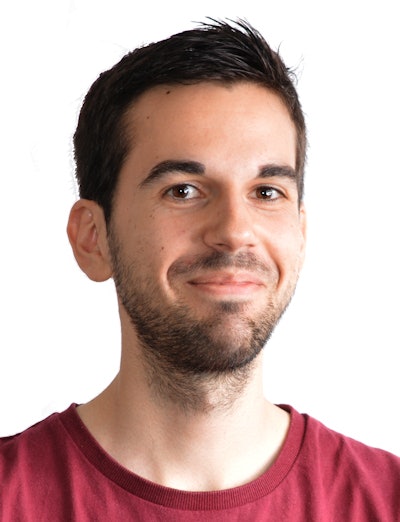
The study findings are good news, said presenter and doctoral candidate Alejandro Rodríguez-Ruiz of Radboud University Medical Center in Nijmegen, the Netherlands.
"Whether using the system as a decision-support aid or as an independent first or second reader, the implications on clinical practice could be enormous in terms of improved radiologist performance and cost-efficiency," Rodríguez-Ruiz told AuntMinnie.com via email.
In fact, like it or not, artificial intelligence in breast imaging is the hot new research frontier, Rodríguez-Ruiz said.
"We're on the verge of a new era of research in mammography, similar to how in the last decade, digital breast tomosynthesis gave rise to many studies to assess its full potential," he said.
Rodríguez-Ruiz and colleagues compared the performance of a commercial deep-learning computer detection system (Transpara version 1.2.0, ScreenPoint Medical) with that of radiologists in detecting breast cancer on digital mammography images. The study included 24 radiologists who retrospectively reviewed 1,435 two-view digital mammography exams, 336 of which were malignant (23%) and 430 of which were benign (30%). The radiologists ranked the exams on a scale of 0 to 10, with 0 indicating no lesion was present and 10 indicating a lesion that was "definitely malignant."
The group then applied a deep-learning algorithm to the mammogram dataset. The algorithm identifies soft-tissue and calcified lesions and combines the findings of all available views into a single cancer suspiciousness score (based on the same scale the readers used). Finally, Rodríguez-Ruiz and colleagues calculated the area under the receiver operating characteristic curve (AUC) for both the radiologist readers and the algorithm.
The AUC of the computer system was higher than that of 11 of the 24 readers, lower than 11 of them, and equal to two, Rodríguez-Ruiz said. These results were not statistically significant.
AI assistance
Should radiologists be worried that a machine performs so well for breast cancer detection? Absolutely not, Rodríguez-Ruiz told AuntMinnie.com.
"They should be excited, rather than concerned," he said. "There are many applications of AI for reading mammograms -- from decision support and screening-case triaging to second reading and resident training -- but all of them are oriented to improving radiologist performance rather than replacing them."
The bottom line is that AI could help busy radiology practices streamline their workflow, according to Rodríguez-Ruiz.
"We believe that our findings show, for the first time, that a new way of reading mammograms is possible," he said. "Once we are certain of the satisfactory standalone performance of this AI system, we can further investigate and assess its clinical applications and what is the actual benefit for radiologists and patients. Whether using the system as a decision-support aid or as an independent first or second reader, the implications on clinical practice could be enormous in terms of improved radiologist performance and cost-efficiency."