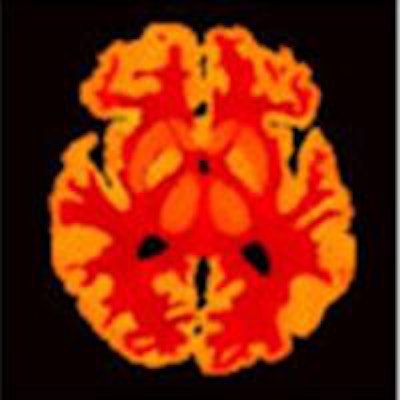
Quantification of PET images is limited by the relatively poor spatial resolution of the acquired data and the associated partial volume effects, as well as the inherently low signal-to-noise ratio of PET. Smoothing performed after image reconstruction can improve the signal-to-noise ratio. However, this process is typically achieved using Gaussian filtering, which also reduces the spatial resolution and deteriorates the quantification accuracy of small structures.
The introduction of hybrid imaging systems such as PET/MR scanners provides another option: incorporating information from high-definition structural images into reconstruction or postreconstruction processes to improve PET image quality. Researchers from the A*STAR-NUS Clinical Imaging Research Center (CIRC) in Singapore have demonstrated this approach using MRI for PET image filtering and partial volume correction in the framework of postreconstruction processing.
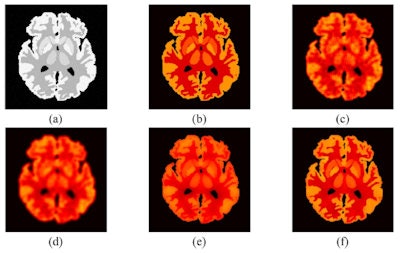
"We aimed to develop fast voxel-wise filtering and partial volume correction techniques to improve the signal-to-noise ratio and spatial resolution of brain PET images with the help of high-definition structural MR images," said Jianhua Yan, from CIRC.
Yan and colleagues adapted a recently proposed guided filtering method to perform PET image filtering by introducing an anatomical MR image as a prior term. This approach (called MRI-guided filtering) assumes a local linear model between the guidance image -- in this case, a coregistered high-resolution MR image -- and the filtered output.
Using the point spread function (PSF) of the PET system to account for the resolution mismatch between MRI and PET, they also proposed a cost function for PET partial volume correction (MRI-guided PVC). Yan notes that it is not necessary to perform both filtering and partial volume correction. "If the PSF information is available, we can just use MRI-guided PVC to improve the resolution and signal-to-noise ratio," he explained.
Key comparisons
The researchers compared their proposed MRI-guided filtering and MRI-guided PVC methods with Gaussian filtering. They first assessed the performance of the three methods using simulated dynamic 2D PET brain data. For the simulation, they used a high-resolution Zubal head phantom with 128 slices containing 256 x 256 pixels of 1.5 x 1.5 mm. They selected slice 64 for this study and simulated four groups of structures within this slice. To determine the PSF, they simulated a point source in the center-of-view and fitted the reconstructed profile with a Gaussian function (Physics in Medicine and Biology, 9 January 2015, Vol. 60:3, pp. 961-976).
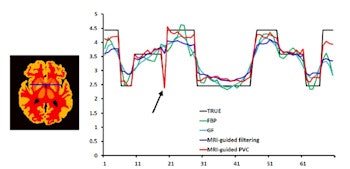
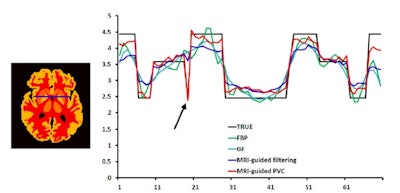
Signal profiles through the blue line (in the left image) for true PET, FBP (uncorrected), Gaussian filtering (GF), MRI-guided filtering and MRI-guided PVC.
A typical PET image reconstructed by filtered back projection (FBP) was seen to be highly noisy. Gaussian filtering effectively smoothed the noise, but also blurred the edges within the image. Images produced by MRI-guided filtering and MRI-guided PVC exhibited dramatically reduced noise. In addition, MRI-guided PVC further improved the resolution recovery, as seen in thin structures such as the occipital cortex.
The team also evaluated the methods using three common metrics: mean squared error (MSE); peak signal-to-noise ratio (PSNR); and structural similarity index (SSIM). Gaussian filtering led to higher MSE compared with the unfiltered reconstructed image, while MRI-guided filtering reduced MSE, and MRI-guided PVC decreased it further. In terms of PSNR, Gaussian filtering provided limited improvement, while MRI-guided filtering and PVC yielded increases of about 10% and 50%, respectively. MRI-guided filtering and PVC were also superior to Gaussian filtering with regard to SSIM, with MRI-guided PVC exhibiting the highest SSIM value.
A profile through the PET image was used to quantitatively compare PET intensity recovery from the images. As expected, loss of edge structures was most obvious in the Gaussian filtered image. Overall, MRI-guided filtering and PVC exhibited optimal performance, with MRI-guided PVC best at recovering intensity and resolving edge structures.
Clinical data
Yan and colleagues also applied their methods to F-18-FDG brain data acquired from a cervical cancer patient using an integrated PET/MR scanner (Siemens Healthcare's Biograph mMR).
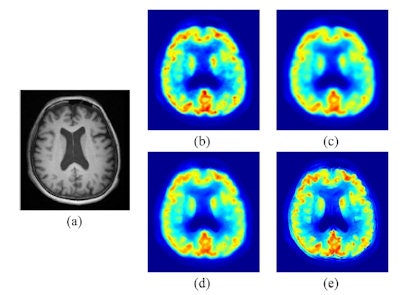
Four representative axial PET images (reconstructed without smoothing; Gaussian filtered; MRI-guided filtered; and MRI-guided PVC) showed that, again, MRI-guided PVC provided the best structure delineation while keeping noise under control. Profiles taken through the image also supported the finding that MRI-guided PVC can effectively recover intensity and improve contrast.
The authors note that most existing PVC methods for PET require segmentation of the anatomical information, which is challenging and time-consuming for brain images. The new filtering and PVC approaches, on the other hand, are performed on the voxel level and avoid the MRI segmentation task.
"We are now working on evaluating the proposed filtering and PVC methods for other anatomical regions outside the brain," Yan toldmedicalphysicsweb. "In addition, the effect of the proposed methods on kinetic parameter estimates for dynamic brain PET imaging is also being investigated."
© IOP Publishing Limited. Republished with permission from medicalphysicsweb, a community website covering fundamental research and emerging technologies in medical imaging and radiation therapy.