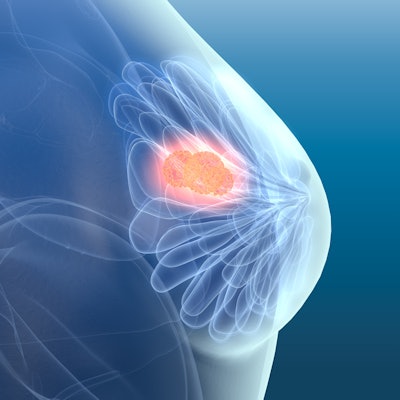
An artificial intelligence (AI) algorithm can accurately characterize breast density on mammography, according to a study published on 16 March in Radiology: Artificial Intelligence.
The findings suggest that AI could mitigate reader discrepancies regarding breast density, said study co-author Dr. Sergio Papa of Centro Diagnostico Italiano in Milan in a statement released by the RSNA.
"The particular value of this tool is the possibility to overcome the suboptimal reproducibility of visual human density classification that limits its practical usability," Papa said. "To have a robust tool that proposes the density assignment in a standardized fashion may help a lot in decision-making."
Dense breast tissue is considered a risk factor for breast cancer because it can reduce mammography's sensitivity by masking cancer lesions. It is typically evaluated using the four-point BI-RADS scale, noted a group led by Dr. Veronica Magni of the Università degli Studi di Milano. Women with dense tissue are typically referred for supplemental breast cancer screening via ultrasound or breast MRI.
However, use of the BI-RADS breast density scale can be susceptible to interobserver and intraobserver variability. So Magni and colleagues investigated whether an AI algorithm could diminish this variability.
"[The] aim of this study was two-fold: to develop and externally validate an AI-driven fully-automated software for breast density classification mimicking a consensus-based human visual assessment; and to assess its agreement and reliability in the clinical setting," the researchers explained.
The group developed and used an algorithm called TRACE4BDensity (DeepTrace Technologies, Milan, Italy) for breast density classification based on convolutional neural networks from mammograms obtained between 2017 and 2020. The algorithm was trained with 760 mediolateral oblique (MLO) images taken in 380 women that were interpreted by seven radiologists; three of these radiologists used a set of 384 MLO images in 197 women to validate the model.
The investigators found the following:
- The model showed an 89.3% accuracy in distinguishing BI-RADS A (almost entirely fatty tissue) or B (scattered areas of fibroglandular density), compared with C (dense tissue) or D (extremely dense tissue) categories.
- The model showed an agreement of 90.4% (178/197 validation mammograms) among the three validation readers.
- The model showed a kappa value for reliability of 0.81.
"Distinguishing dense from nondense breasts is indeed the most clinically relevant task and may drive the potential referral to supplemental screening," they concluded. "[AI] offers a robust way to overcome the variability of human visual assessment."