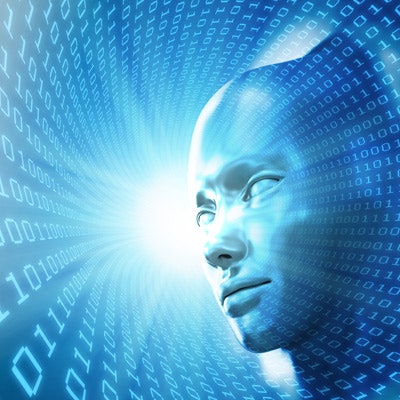
A type of artificial intelligence (AI) algorithm called a generative adversarial network (GAN) can learn to insert suspicious lesions into -- or remove them from -- mammograms, but the technology isn't yet capable of fooling radiologists, according to Swiss research posted online on 10 September in the European Journal of Radiology.
A group led by Dr. Anton Becker from University Hospital Zürich found that a GAN could learn on its own what image features are suspicious for cancer. Although radiologists could not distinguish between original and modified images on lower-resolution studies, they were able to do so easily on higher-resolution exams.
"With increasing memory and computing availability, GANs could potentially be used as a cyberweapon in the near future," they wrote.
Competing networks
GANs are a subclass of deep-learning algorithms and consist of two neural networks that compete against each other. A generator network manipulates sample images, while a discriminator network distinguishes between the real and manipulated samples. Essentially, the neural networks are competing to improve their own performance, according to the researchers. With enough time and resources, the generator network will theoretically be able to produce perfectly manipulated samples that can't be distinguished by the discriminator network.
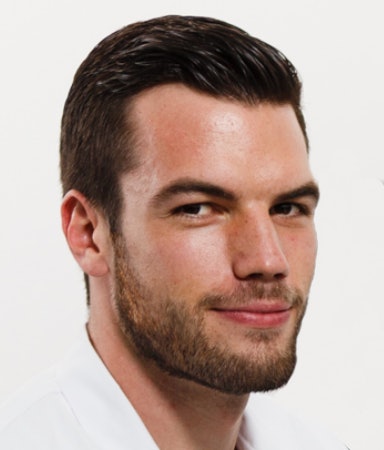
The researchers sought to determine if a pair of GANs could be trained to add or remove suspicious features from mammograms and to ascertain whether radiologists could detect these AI-mediated attacks. They trained a so-called cycle-consistent GAN model (CycleGAN), which is designed to translate images from one category (i.e., a normal mammogram) to another type (i.e., a mammogram with cancer) and vice versa.
The authors gathered 680 mammographic images from 334 patients included in the Breast Cancer Digital Repository (BCDR) and INbreast publicly available datasets. Of these 680 images, 318 showed potentially cancerous masses and 362 were negative control cases. They trained the model on a downscaled version (256 x 256 pixels) of the images. The GANs were then tested on an internal dataset consisting of 302 cancers and 590 controls.
Next, the researchers performed an experiment with three radiologists, including 30 modified images and 30 original images. There were 40 images in pairs, and 20 images of different patients. Suspicious features had been added to half of the modified images and removed in the other half. The radiologists read the images in random order and rated the presence of suspicious lesions on a scale of 1 to 5. They also ranked their likelihood of the image being manipulated.
Limited performance, for now
The experiment was performed first on lower-resolution images (256 x 256 pixels) and then repeated on higher-resolution images (512 x 408 pixels). The researchers found that overall radiologist performance wasn't affected by the GAN modifications on the lower-resolution images, although one radiologist did have a lower cancer detection rate that did not reach statistical significance. However, the radiologists could not distinguish between original and modified images (area under the curve [AUC] = 0.55, p = 0.45).
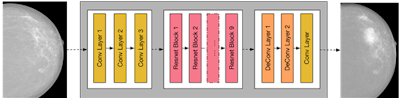
All radiologists showed a significantly lower detection rate (AUC = 0.37) on the modified high-resolution images than on the original images (AUC = 0.80). The difference was statistically significant (p < 0.001). They were also able to identify the modified images, as the artifacts were easier to see on the higher-resolution images, according to the researchers.
"Our results indicate that while GANs can learn the appearance of suspicious lesions, the modification of images is currently limited by the introduction of artifacts, and the size of the images is limited by technical memory constraints," they wrote.
Positive benefits
GANs also have the potential to contribute value in radiology, such as by discovering new features of a disease, for teaching purposes, and for detecting biases and confounders in training datasets.
"Furthermore, many datasets, especially in a screening setting, are highly unbalanced -- i.e., the cases of healthy individuals far outweigh the ones with cancer," the authors noted. "GANs could be used to create more balanced datasets and thus facilitate training of other [machine-learning] algorithms."
The proof-of-concept study showed that the CycleGAN can implicitly learn suspicious features and inject them into or remove them from mammography images, they concluded. However, the technology is currently limited, with a clear trade-off between the manipulation of images and the introduction of artifacts.
"Nevertheless, this matter deserves further study in order to shield future devices and software from AI-mediated attacks," Becker and colleagues wrote.