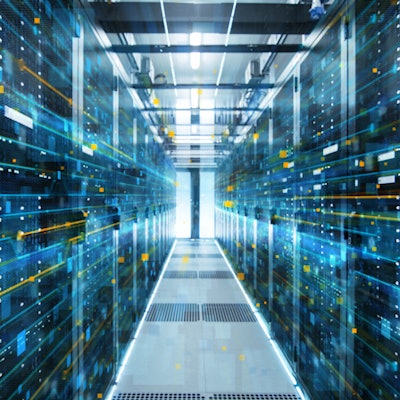
More than 150 independent software companies are developing machine-learning solutions for medical imaging, so sorting through the plethora of options to select vendors is a challenge. Here are 10 factors radiologists should consider -- and questions they should ask -- before partnering with vendors providing artificial intelligence (AI) products for medical imaging.
1. Clinical relevance
The foremost consideration for healthcare providers adopting AI into their clinical workflow is relevancy. Does the AI solution truly address the needs of the healthcare provider, regardless of the associated costs and inconveniences to implement such a solution?
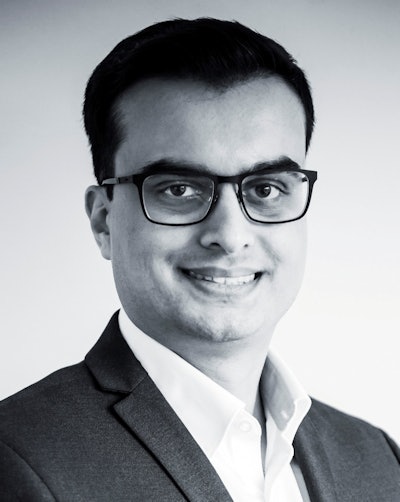
There are hundreds of potential use cases for AI in radiology, but the focus should be on those where AI can make the most impact, such as bottlenecks in the radiologist's workload. This includes repetitive tasks (e.g., manually taking measurements of imaging features) that can be automated, as well as providing additional information and decision support for more complex cases.
Radiologists should ask the following: Is the use case a problem worth solving using AI? (Use cases that are quick and easy to diagnose with limited involvement from the radiologist may not warrant AI.) What is the impact of using AI on the diagnosis and treatment decisions? Will it lead to improved patient care? What are the priorities for the radiologist to use AI and how would this be determined? Is the AI solution future-proof? Will it be relevant in the years to come?
2. Algorithm development
The resurgence of AI can be attributed to the shift in techniques from classic machine learning to the deep-learning approach. The challenge remains for AI vendors to reduce the "black box" phenomenon for the end user (radiologist), and the methodology used for algorithm development is key.
Radiologists should ask the following: How many images were used to develop the algorithm? Were the images obtained from a representative cross-section of population demographics and scanner manufacturers? Who annotated the images? Did the developers use experienced radiologists or residents? How many radiologists annotated each image (ideally two or more)? If two radiologists annotated the images, did the developers use a third radiologist for any discrepancies? Were the annotated images validated using clinical biomarkers or biopsy? Was the image dataset for training and validation of the algorithm different (and unseen) from the one used for testing the algorithm?
3. Clinical validation
Once developed, AI algorithms should ideally undergo clinical studies to test their robustness, accuracy, and reproducibility.
Radiologists should ask the following: What type of clinical study was used for the validation? Prospective studies using both case and noncase data outweigh the significance of retrospective studies. Did the clinical study involve single or multiple readers? Was it a single-center or multicenter clinical study? How does the performance of the AI algorithm compare to existing clinical practice? The AI solution should be equal to or better than the current standard of practice.
4. Product regulation
Only solutions with regulatory clearance may be used in clinical practice. At present, 57 vendors have received regulatory approval for 77 machine-learning algorithms for medical imaging from one of the four major regulatory bodies: Food and Drug Administration (FDA, U.S.), CE Mark (Europe), Pharmaceuticals and Medical Devices Agency (PMDA, Japan), and Ministry of Food and Drug Safety (MFDS, South Korea).
Radiologists should ask the following: Does the vendor have regulatory approval from the FDA? If so, what type of clearance has it received, i.e., 510(k), premarket approval, or de novo? Has the algorithm been cleared in full or only in part? If in part, which part is approved and why? Has this been clearly labeled for the end user? Is the solution regulated in any other market -- for example, in neighboring countries or other major markets (e.g., CE Mark)? Does the vendor have further regulatory applications in the pipeline?
5. Workflow integration
The results from AI solutions need to integrate seamlessly into the radiologist's preferred diagnostic viewer. Radiologists should be able to view and potentially interact with (edit/accept/reject) the findings without compromising productivity.
Radiologists should ask the following: Are the AI results integrated into the PACS interface, or does the algorithm require a separate user interface to view the results? Can the AI results be edited, accepted, or rejected based on the radiologist's clinical judgment? How are the results displayed? Are they presented as an overlay on the image or as a separate report? Is the AI solution PACS/modality vendor-agnostic?
6. Return on investment
Related to clinical relevancy, radiologists and healthcare providers expect to see a return on investment (financial and nonfinancial reasons) for AI solutions.
Radiologists should ask the following: Is the AI solution a productivity tool or a solution focused on quality improvement? Healthcare providers with a shortage of radiologists may value AI algorithms that increase productivity, but other providers may find value in algorithms that improve the quality of diagnoses and treatment decisions. Does the AI solution alter the diagnostic pathway, providing value to both the patient and healthcare provider? For example, does it reduce the need for invasive procedures and, in turn, the risk to the patient? Does the AI solution alter the treatment pathway, resulting in cost savings for the healthcare provider?
7. Deployment
Delivering an AI solution to the healthcare provider in a secure, fast, and cost-effective manner is key for its success.
Radiologists should ask the following: Is the AI solution installed locally onsite, or does it require access to a cloud server? If cloud-based, is the protected health information (PHI) removed before the image is analyzed by the AI algorithm? Does the AI algorithm require a dedicated virtual machine? Can it be "containerized" (e.g., Docker)?
8. Support and contracting
As radiologists implement AI solutions from multiple vendors, consideration should be given to the administrative overhead of handling individual contracting and invoicing. The level of technical support provided by algorithm developers is another important consideration, along with accountability, should the algorithm negatively impact the performance of the PACS.
Radiologists should ask the following: Who will provide technical support for the AI algorithms? Will it be the algorithm developers or their distribution partners? How will the solution be licensed? Will it be a one-time (perpetual) license, or will it be a recurring software-as-a-service (SaaS) license? What is the pricing model used by the developer? Does the developer set a fee on a pay-per-use basis (e.g., dollars per set number of scans), or does it charge one price (regardless of the algorithm usage)?
9. Capital funding
Many of the AI algorithm developers are startup companies that rely on external capital funding. The ability to raise capital through multiple funding rounds is reflective of a vendor's potential long-term success.
Radiologists should ask the following: How much capital funding has been raised to date, and who are the investors? How many funding rounds has the vendor secured? How long does its typical funding round last, and when was the most recent round completed?
10. Vendor leadership team
The credibility and experience of the leadership team (C-suite) and medical advisory board are key to a vendor's success in terms of product development and go-to-market strategy.
Radiologists should ask the following: Who is on the leadership team and medical advisory board? Does the leadership team have experience in taking healthcare technology products to market? Do members of the leadership team have previous similar experiences working for successful, revenue-generating companies?
Sanjay M. Parekh, PhD, is a senior market analyst at Signify Research, a health tech, market intelligence firm based in Cranfield, U.K. This article was originally published in the November 2019 issue of HealthCare Business News magazine.
The comments and observations expressed herein do not necessarily reflect the opinions of AuntMinnieEurope.com, nor should they be construed as an endorsement or admonishment of any particular vendor, analyst, industry consultant, or consulting group.