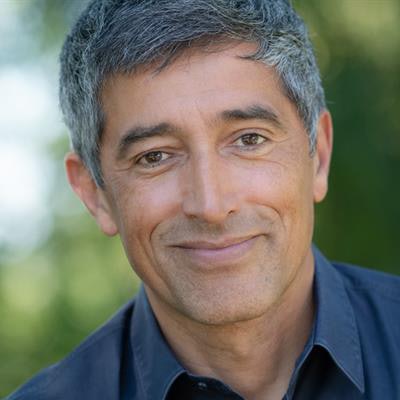
In June, Ranga Yogeshwar, a physicist from Luxembourg who is also well known in Germany as a science journalist and TV presenter, was interviewed for the June 2019 newsletter of the German Röntgen Society (Deutsche Röntgengesellschaft, or DRG). His comments on artificial intelligence (AI) were knowledgeable, competent, critical, and to the point. He also said the following:
The question [is] of how we validate data, also in science. Where do training data come from? Are they certified? How certain can we be that training data may not contain an a priori error? I still miss a differentiated discussion. It is sometimes frightening on what basis data are collected and trained, even in powerful AI systems. ... For me, it is a question of reflected progress, in which data is validated on the one hand and data flows and access rights are clearly regulated on the other.1
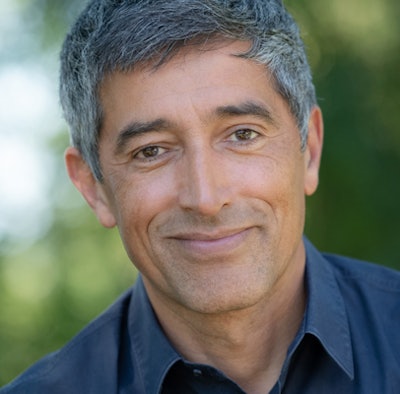
Validation is a neglected, or simply ignored, factor. In many cases, the data quality of the input is bad and the necessary trustworthy infrastructure does not exist or requires a much greater technical effort than expected. In many instances, the complexity of the problem to be solved is taken into account neither by the promoters of the application nor by its users because they don't understand the first thing about it.
Often complex software and hardware used are impossible to link -- it's not only one program but also many components that have to connect and grasp the incoming data to process them in the expected way. In newspeak, this is politely called "lack of maturity."
Hurdles to overcome
The difficulty of implementing validation -- for instance, for contouring tools and applying them in AI studies -- is demonstrated in a recent paper by Zheng Chang:
Before the AI contouring tool is fully adopted into clinical use as a part of standard practice, it needs validation in more independent multicenter studies with larger patient cohorts. Although the AI contouring tool shows promising results for [nasopharyngeal carcinoma (NPC)] primary tumor delineation in this study, section-by-section verification of tumor contour by radiation oncologists should never be omitted.2
In the 1980s and 1990s, I led an image processing group in the radiology department I headed. A number of important innovations in the field of image processing, image visualization, data collection, and early applications of very specific AI were developed during this time and became basic and expert knowledge, including the knowledge of pitfalls and setbacks.3
Validation is among them; it seems nearly impossible because the parameters of most digital radiological examinations are not exactly reproducible. However, extremely thorough validation must take place before AI algorithms are clinically feasible.
A Korean paper on AI highlighted that in the first half of 2018, "of 516 eligible published studies, only 6% (31 studies) performed external validation." In addition:
None of the 31 studies adopted all three design features: diagnostic cohort design, the inclusion of multiple institutions, and prospective data collection for external validation. ... Nearly all of the studies published in the study period that evaluated the performance of AI algorithms for diagnostic analysis of medical images were designed as proof-of-concept technical feasibility studies and did not have the design features that are recommended for robust validation of the real-world clinical performance of AI algorithms.4
Radiology's involvement in AI
At the May 2019 conference titled Standing at the Crossroads: 40 Years of MR Contrast Agents, there was an intriguing contribution from researchers in the "hard" sciences that radiologists will be involved in patient studies with common techniques and contrast agents but not in dedicated MR studies with techniques using novel diagnostic, therapeutic, and theragnostic compounds.
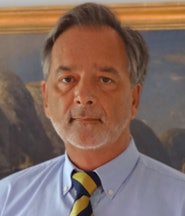
The reason given is the radiologists' lack of background in dedicated MR techniques and biochemical interactions of targeted compounds and tracers. Such examinations or interventions would become the domain of other disciplines, e.g., oncologists and neuroscientists, and perhaps also specialists in nuclear medicine.
The scientists' comments were supported by a cardiologist who stressed that he thinks radiology is experiencing a major change -- most likely a decline. He described how he and his colleagues have undergone training and are now completely independent of any radiology input. He believes there is a similar trend in neurology and orthopedics.
This controversy complicates the potential validation of AI data collection even more.
Another issue is the question of whether radiologists really understand what is happening with and in their equipment and the optimization of examinations. Basic T2- or T2*-weighted sequences, for instance, have been superseded by all sorts of concealed manipulations to improve speed -- tricks that are hidden and of which users are largely unaware.
Similarly, with CT, the impact of energy, contrast agent volume, and timing means most radiologists are completely dependent on built-in protocols. Thus, general radiologists or other imaging professionals tend to be excluded from making any changes to patient studies.
It is interesting how radiology is seen by some of the scientists developing the tools for radiology. This opinion will not find many friends in the radiological community, but the involvement of nonradiologists has already been noticeable for some time. More so, simple applications of artificial intelligence will shift routine image assessment away from radiologists.
Human laziness will rely on AI. There is more to this laziness than commonly thought. The human brain delegates tasks to the background that it doesn't consider relevant. Relying on the "responsible" performance of hardware and software will allow vigilance to easily fade.
A good example is the shift of our cognitive systems from the task of supervising a fully autonomous device to a less relevant device, e.g., away from the performance of a radiological AI system. It was shown in a group of people that driving cars with manual transmission was associated with better attention and less failure than driving cars with automatic transmission.5 To my knowledge, nobody has ever looked into such issues possibly also to be found with AI, but it's important to be aware of them.6
References
- Yogeshwar R. Die daten sind das programm. Deutsche Röntgengesellschaft website. https://www.drg.de/de-DE/5327/kuenstliche-intelligenz/. June 2019. Accessed 23 July 2019.
- Chang Z. Will AI improve tumor delineation accuracy for radiation therapy? Radiology. 2019;291(3):687-688. doi:10.1148/radiol.2019190385.
- Rinck PA. Chapter 15: Image processing and visualization/Chapter 16: Dynamic imaging. In: Rinck PA. Magnetic Resonance in Medicine. A Critical Introduction. 12th ed. Norderstedt, Germany: BoD; 2018.
- Kim DW, Jang HY, Kim KW, Shin Y, Park SH. Design characteristics of studies reporting the performance of artificial intelligence algorithms for diagnostic analysis of medical images: results from recently published papers. Korean J Radiol. 2019;20(3):405-410. doi:10.3348/kjr.2019.0025.
- Cox DJ, Punja M, Powers K, et al. Manual transmission enhances attention and driving performance of ADHD adolescent males: pilot study. J Atten Disord. 2006;10(2):212-216.
- Rinck PA. Total reliance on autopilot is a risk to life. Rinckside website. http://www.rinckside.org/Rinckside%20Columns/2012%2010%20Autopilot.htm. October 2012. Accessed 23 July 2019.
Dr. Peter Rinck, PhD, is a professor of radiology and magnetic resonance and has a doctorate in medical history. He is the president of the Council of the Round Table Foundation (TRTF) and the chairman of the board of the Pro Academia Prize.
The comments and observations expressed herein do not necessarily reflect the opinions of AuntMinnieEurope.com, nor should they be construed as an endorsement or admonishment of any particular vendor, analyst, industry consultant, or consulting group.