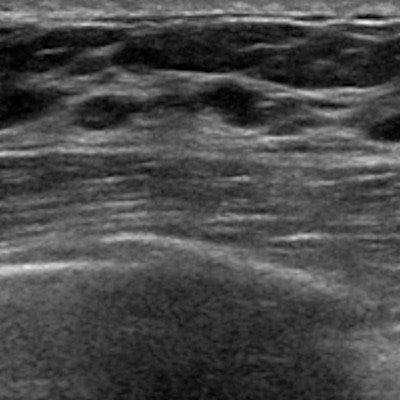
An artificial intelligence (AI) algorithm matched the performance of radiologist readers in detecting and classifying lesions on breast ultrasound exams, investigators from Switzerland reported in research published online on 29 March in European Radiology.
The study results suggest that their algorithm -- a deep convolutional neural network (CNN) -- could mitigate reader variability in breast ultrasound interpretation and reduce false positives and unnecessary biopsies, according to a team led by Dr. Alexander Ciritsis of University Hospital Zurich.
"The objective classification for breast lesions in ultrasound images suffers from a variability in inter- and intrareader reproducibility as well as from a high rate of false-positive findings, particularly in screening settings," the authors wrote. "Thus, a standardization of lesion classification based on ultrasound imaging could potentially decrease the number of recommended biopsies."
In ultrasound imaging, breast lesions are characterized according to the American College of Radiology's BI-RADS categories. But it can be difficult to classify breast lesions objectively in ultrasound images because of operator variability, Ciritsis and colleagues noted. Computer-aided diagnosis (CADx) systems have been used to support radiologists in their image interpretations, especially in the effort to distinguish between benign or suspicious lesions. But a deep-learning network could take this to another level.
"Deep-learning techniques could change the existing framework of CADx systems by relying on automatic feature extraction and thus alleviate and standardize the process of semiautomatic image segmentation and classification," noted the investigators, who sought to evaluate the performance of a deep CNN for detecting, highlighting, and classifying lesions on breast ultrasound.
The researchers used 1,019 breast ultrasound images from 582 patients to train the CNN. They then tested the neural network's accuracy on a dataset of 101 images that included 33 BI-RADS 2, 47 BI-RADS 3, and 21 BI-RADS 4-5 lesions; these images were also interpreted by two radiologist readers.
Ciritsis and colleagues used radiological reports, histopathological results, and follow-up exams as a reference, and they assessed the performance of the algorithm and the radiologists in terms of lesion classification accuracy and area under the receiver operating characteristic curve (AUC). They found that the neural network outperformed radiologist readers in a number of measures.
Radiologist reader performance vs. neural network | ||
Performance measure | Radiologists | Neural network |
Accuracy of differentiating BI-RADS 2 from BI-RADS 3-5 lesions | 79.2% | 87.1% |
Accuracy of differentiating BI-RADS 2-3 from BI-RADS 4-5 lesions | 91.6% | 93.1% |
AUC | 84.6 | 83.8 |
Specificity | 88.7% | 92.1% |
Sensitivity | 82% | 76% |
Because the study showed that the neural network's performance was at least comparable to -- if not better than -- that of experienced radiologist readers, it seems clear that the deep CNN shows promise as a tool for making breast ultrasound more accurate, according to the researchers.
"Our study demonstrated that deep convolutional neural networks may be used to mimic human decision-making in the evaluation of single ultrasound images of breast lesions according to the BI-RADS catalog," the group wrote.