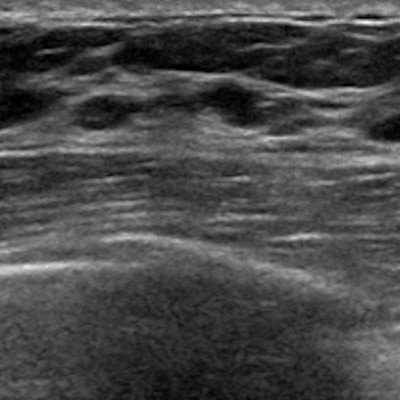
Artificial intelligence (AI) algorithms can yield a promising level of performance for differentiating between benign and malignant solid breast masses on B-mode ultrasound images, but further improvement is still needed, according to research published online on 5 August in European Radiology Experimental.
Researchers from Brazil trained and evaluated five different machine-learning algorithms to characterize breast ultrasound exams using 10 BI-RADS radiomics features. The highest-performing classifier yielded an area under the curve (AUC) of 0.840.
"[Machine learning] can aid the distinction between benign and malignant breast lesion on ultrasound images using quantified BI-RADS descriptors," wrote Dr. Eduardo Fleury, PhD, of the Instituto Brasileiro de Controle do Câncer (IBCC) in São Paulo and Karem Marcomini, PhD, of the Universidade de São Paulo (USP) São Carlos.
In the past, computer-aided diagnosis software provided results that many considered to be unsatisfactory. That's because the software was trained to classify lesions as humans do, but it lacked the abstract senses utilized by the human brain, according to the researchers.
"With the use of machine-learning systems, it is now possible to make an analogy of the subjective data used by humans with objective information used by computers," the authors wrote. "In this way, the computer can classify the lesions in an analogous way to the human beings."
Fleury and Marcomini sought to determine if BI-RADS computerized features -- quantified by "translating" descriptive terms from the BI-RADS lexicon into features that can be automatically computed by algorithms -- could be used to enhance the diagnosis of breast ultrasound exams. They assessed five machine-learning algorithms: multilayer perceptron, decision tree, linear discriminant analysis, random forest, and support vector machine (SVM).
The algorithms were trained and tested on a database of 206 consecutive cases with lesions: 144 benign and 62 malignant masses. A radiologist with two years of breast imaging experience acquired all diagnostic ultrasound exams using 5- to 10-MHz linear transducers on one of four systems: Nemio 30 (Canon Medical Systems), Aplio 400 (Canon), VFX 13-5 (Siemens Healthineers), and FV 10-5 (Siemens).
After the contours of the lesions were delineated on the grayscale images, 10 BI-RADS computerized features were extracted: area of difference with equivalent ellipse; lesion orientation; average of difference vector; number of peaks on the distance vector; average of the distance vector; area difference between the convex hull and tumor; echogenicity; entropy; shadow; and lesion size. Tenfold cross-validation was used for training and testing the machine-learning classifiers.
The SVM classifier produced the highest level of performance among the five machine-learning algorithms.
Best machine-learning algorithm performance for characterizing breast masses on US | |||
Sensitivity | Specificity | Area under the curve | |
SVM classifier | 71.4% | 76.9% | 0.840 |
"Machine-learning systems based on BI-RADS feature can help in malignant/benign differentiation but further improvement is needed," they wrote.
The researchers pointed out that a 2016 study in the literature reported that radiologists using descriptors in the fifth edition of BI-RADS produced an AUC of only 0.690. In other findings in the current study, the lesion margin and orientation were the optimal radiomics features for all of the machine-learning methods tested.
"As a future work, we intend to increase the number of samples from our image database to allow the use of other validation methods and ensure greater data reliability, especially by using an external dataset," they wrote. "Another perspective is to include new methods for selecting the best feature set. Finally, we intend to verify the classification performance through convolutional neural networks, eliminating the need for feature extraction and selection."