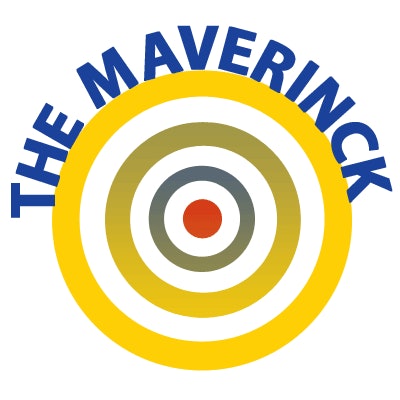
The point of artificial intelligence (AI) is that it "learns" on its own and becomes an expert -- or possibly even the one and only expert. However, AI is not as simple an approach as it's being sold today.
AI, or expert systems, is not a new idea. It has come and gone since the 1940s, or even since the 18th century, with Maelzel's chess-playing machine, The Turk. The reliance on advanced scientific theories, modes of reasoning, and the utilization of scientific methodology, specifically observation, can easily lead to tunnel vision, wrong conclusions, or as it's known from the 19th century, "ratiocination."
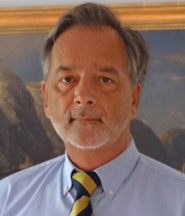
The famous first medical application of AI was MYCIN, a program developed in the 1970s at Stanford University in California.1
MYCIN, as Bruce G. Buchanan and Edward H. Shortliffe described it in a recapitulation of the project, was software that embodied some intelligence and provided data on the extent to which intelligent behavior could be programmed. The intention was to identify bacteria causing severe infections, such as bacteremia and meningitis, and to recommend antibiotics at the right dosage for a patient. As with other AI programs, its development was slow and not always in a forward direction.
It worked, but it also didn't, and was never used in practice -- not only because computing power was insufficient, but rather for an inherent problem of AI: the knowledge of a human expert cannot be translated into digitizable rule bases.
Additionally, AI is not immune to human prejudice that always exists -- wittingly or unwittingly. Such preconceptions cannot be filtered out because of AI's lack of a critical mind. Buchanan described this problem in a conclusion:
There are many "soft" or ill-structured domains, including medical diagnosis, in which formal algorithmic methods do not exist. In diagnostic tasks there are several sources of uncertainty besides the heuristic rules themselves. There are so-called clinical algorithms in medicine, but they do not carry the guarantees of correctness that characterize mathematical or computational algorithms. They are decision flow charts in which heuristics have been built into a branching logic.2
The flaws
AI is mindless. This is a fundamental flaw. Although its developers meant it to be a "science," AI is not a real science; it's closer to computer gambling and tinkering than to creating a fundamentally reliable support system for highly specific tasks.
Neural AI networks are good at (crudely) classifying pictures not only in radiology; meanwhile they encompass the entire spectrum of medical imaging, including, for example, nuclear medicine, dermatology, and microscopy. They were known for years as CAD, or computer-assisted diagnosis.
A typical example is a May 2018 article by a dermatology group at Heidelberg University in Germany. They used deep-learning neural networks for the detection of melanomas. The U.K newspaper The Guardian summarized the press release from Heidelberg with the headline: "Computer learns to detect skin cancer more accurately than doctors."
The authors of the research paper in Annals of Oncology concluded: "Most dermatologists were outperformed by the neural networks. Irrespective of any physicians' experience, they may benefit from assistance by a neural networks' image classification."3
In an editorial accompanying the dermatology article, the commentators were more careful and raised some additional concrete questions.
"This is the catch; for challenging lesions where machine-assisted diagnosis would be most useful, the reliability is lowest," they wrote. "Whilst dermatology is a visual specialty, it is also a tactile one. Subtle melanomas may become more apparent with touch as they feel firm or look shiny when stretched."4
Legal responsibility
Another main problem of AI is the overwhelming majority of its users do not understand and cannot follow its black box judgments and its reasoning to reach certain choices. Interestingly, there also are a number of reports that developers of AI software did not understand why their algorithms reach certain results and decisions; the algorithms are impenetrable.
Thus, the well-meant "right to an explanation" of decisions made by an AI expert system concerning a person, passed as a European law in the General Data Protection Regulation (GDPR), can hardly be fulfilled. Even some creators are unable to find inherent flaws in their source code, so they won't be able to explain it to their "victims." I wonder what the legal consequences will be.
It is a principle of information technology that convenience and security are generally mutually exclusive. Once again the question arises whether the limits of what is ethically permissible are being shifted because something is technically possible. However, financial and career interest often override established values of the medical profession. More so, there are other interests in forcing the introduction of AI by groups and institutions owing no allegiance and acknowledging no responsibility to patients, doctors, or people in general.
At this point we are faced with another question -- who is really responsible and accountable for the quality of the results? The radiologist, the hospital's administrator, the software engineer who wrote the source code, the company that sold the software?
The companies will reject any responsibility, stating the AI software was delivered free of defects. Even if the customer will get access to the source code, nobody will ever be able to prove the algorithm has a flaw. You may have bought a pig in a poke -- and are stuck with it.
Understanding AI
There are other problems. In a recent overview of AI in AuntMinnieEurope.com, Dr. Neelam Dugar stated:
The accuracy of these algorithms is dependent on two important factors: the type of algorithms used and also the acquisition parameters applied by the modality. If the algorithm is to be accurate, it is really important the acquisition parameters are standardized prior to application of the algorithm.5
This is a major dilemma of AI and deep learning. In many instances, the calculated parameter data are incorrect, as we have seen in "MR fingerprinting" and related methodologies. These values cannot be reliably reproduced, thus they shouldn't be used in a neural network.6 Deep learning can lead to the description of complex relationships that might only exist because they are based on artifacts or wrong presumptions.
Simple tasks are easily solved by AI, but multilayered tasks are far more complicated to work out. During the last 10 years, neural networks have shown promises. Still, AI doesn't mean an understanding, thinking, and comprehending computer, but programmed "if-then" ordered decisions. At the present stage, AI is more real incompetence that easily can run wild and lose control than helpful support in diagnosis.
AI is also claimed to be objective. But there is no objectivity or neutrality in AI, its decisions are not necessarily knowledge based, but biased. More so, quantifying algorithms freeze a state of the past because they use old data.
Artificial imaging programs are useless if applied randomly without a well-defined and sharply delineated aim. Many approaches to explain results of AI are based on hypotheses that are still to be proved, and much research in this field is empirical and heuristic.
Still, AI will come on to the market; its business value is enormous. By the way: If AI should work, even limping and stuttering, other disciplines will take over radiology in those fields they find attractive -- because with fast AI results, it's easy and makes money. Anyone can use it, from technologists to physicians in clinical disciplines. Radiologists are not needed for this.
Dr. Peter Rinck, PhD, is a professor of radiology and magnetic resonance and has a doctorate in medical history. He is the president of the Council of the Round Table Foundation (TRTF) and the chairman of the board of the Pro Academia Prize.
References
- Buchanan BG. A (very) brief history of artificial intelligence. AI Magazine. 2005;26(4): 53-60.
- Buchanan BG, Shortliffe EH (eds). Rule-based expert systems: The MYCIN experiments of the Stanford Heuristic Programming Project. Reading, MA: Addison Wesley; 1984:683.
- Haenssle HA, Fink C, Schneiderbauer R, et al. Reader study level-I and level-II Groups. Man against machine: diagnostic performance of a deep learning convolutional neural network for dermoscopic melanoma recognition in comparison to 58 dermatologists. Annals of Oncology. 2018 Aug 1;29(8):1836-1842.
- Mar VJ, Soyer HP. Artificial intelligence for melanoma diagnosis: How can we de-liver on the promise? Annals of Oncology. 22 May 2018.
- Rinck PA. Relaxation time measurements in medical diagnostics. In: Magnetic resonance in medicine. A critical introduction. 12th ed. BoD, Norderstedt, Germany; 2018:pp. 87-92.
- Dugar N. AI algorithms begin to loom large in radiology. AuntMinnieEurope.com, 27 June 2018. https://www.auntminnieeurope.com/index.aspx?sec=sup&sub=pac&pag=dis&ItemID=616072. Accessed 25 September 2018.
The comments and observations expressed herein do not necessarily reflect the opinions of AuntMinnieEurope.com, nor should they be construed as an endorsement or admonishment of any particular vendor, analyst, industry consultant, or consulting group.