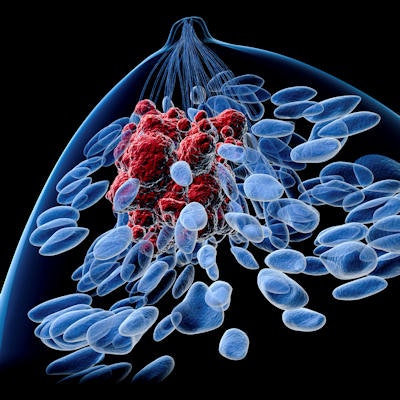
Researchers from Austria have developed a new computer-aided segmentation and diagnostic (CAD) algorithm to distinguish between cancerous and benign breast lesions using PET and MRI data. Their study was published online on 27 April in European Radiology Experimental.
The automated data-driven CAD system detected all malignant lesions and missed only two benign lesions in the study sample. The goal now is to have this CAD approach become a reliable tool for exploring different modalities and their related data to improve the detection and classification of breast lesions.
"Our results showed that automatic lesion segmentation was accurate and improved with information from all modalities, but even a small number of features were sufficient to achieve the reported maximum accuracy," noted the group led by co-first authors Wolf-Dieter Vogl, PhD, and Dr. Katja Pinker, PhD, from the Medical University of Vienna.
In recent years, clinicians have used imaging tools and protocols such as dynamic contrast-enhanced (DCE) MRI, diffusion-weighted MRI (DWI-MRI), and FDG-PET to gather information on tumor metabolism, microstructure, and morphology to better differentiate between benign and malignant breast lesions. Hence, there is a need to create CAD methods to target the most relevant data.
"As yet, the information provided by individual imaging techniques as part of multiparametric imaging remains poorly understood," Vogl, Pinker, and colleagues wrote. "To identify the diagnostically relevant parameters captured across DCE-MRI, DWI, and F-18 FDG PET, we propose a novel automated data-driven approach: a combined breast lesion segmentation and classification system for multiparametric imaging data where the system automatically identifies the information in the imaging data that contribute to an accurate segmentation and classification."
To create the CAD algorithm, the researchers first aligned breast imaging data from DCE-MRI, DWI, and FDG-PET and segmented the breast. Lesion segmentation was performed using a machine-learning algorithm that assigned 1 for a lesion or 0 for no lesion to each voxel. From there, they extracted local textural, kinetic, and intensity-based image features and were able to classify lesions as malignant or benign.
To test and validate the CAD system, the researchers retrospectively looked at 34 patients who had undergone a 3-tesla MRI scan (Magnetom Tim Trio, Siemens Healthineers) in the prone position with a four-channel breast coil and a whole-body PET/CT scan (Biograph 64 TruePoint, Siemens) in the prone position. The patients collectively had 22 malignant lesions and 12 benign lesions; two patients had multifocal or multicentric cancer.
When applied to the subjects, the CAD approach identified all 22 (100%) malignant tumors and 10 (83%) of 12 benign lesions. The researchers attributed the two omissions to low tracer uptake. Based on an area under the receiver operating characteristic curve (AUC) of 98%, the CAD system reached sensitivity of 94% and specificity of 93%.
The researchers concluded that their CAD system provides a "means for in-depth understanding of the multivariate information, where redundancies and relationships between imaging data are not obvious. This is essential for further clinical exploitation of imaging parameters."