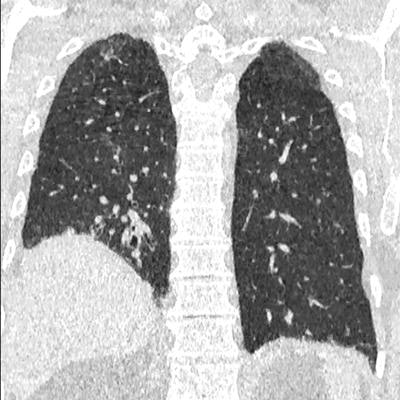
An MRI machine is a lumbering beast. Standing at well over 2 m tall, wide as a family car, weighing well over 1,000 kg, and constantly clunking and heaving away with an unnerving ticking "pssht pssht" sound, it is not exactly a patient-friendly piece of medical equipment.
It can also be deadly. Any ferromagnetic object within near reach will be sucked in toward the powerful magnetic field at accelerating speed, obliterating anything in its path. Add to this the claustrophobia-inducing tunnel you must lie in (about 60 cm to 70 cm wide, just enough clearance for your nose as you lie flat), the loud and staggeringly chaotic banging noise it makes once it's fired up, plus the need to lie absolutely motionless for the whole procedure (up to an hour long), and you can start to understand why patients are somewhat nervous about their MRI scans.
One patient described it to me as "a living coffin ... plenty of time for the mind to wander and reflect on life and the hereafter, while the grim reaper bangs away loudly from the other side."
So why do us doctors put our patients through this experience? Not to be cruel, but to be kind. MRI is a modern marvel of medical technology that allows clinicians to peek inside the human body in exquisite detail without any of the side effects or risks of radiation exposure that come with x-ray or CT.
The human anatomy is largely water-based (hydrogen and oxygen atoms), and MRI capitalizes on that biochemical makeup by using hugely powerful magnetic gradients to control the orientation of every single hydrogen atom in the body, lining them all up in one uniform direction before releasing them and letting them spin away at frequencies only detectable by fine-tuned receivers. The physics behind it is complex yet astounding, and the images that result are quite simply breathtaking.
The small price patients must pay for such excellence is a long time spent in motionless confinement surrounded by clattering noise.
Cutting the burden on patients
Before we look at improving MR acquisition time, it's important to highlight some recent research on lowering CT dose.
Artificial intelligence (AI) may be able to alleviate the burden of extended tube time for patients. What's more, it may also help enhance the diagnostic value of ultralow-dose CT.
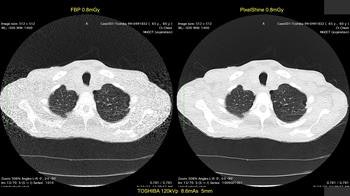
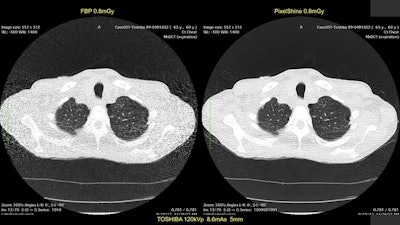
First image: Low-dose CT with filtered back projection (FBP) at 0.8 mGy appears grainy and noisy. Second image: Same CT postprocessed was rated diagnostic quality by independent radiologists. All images courtesy of Algomedica.
At the last annual Society for Imaging Informatics in Medicine (SIIM) conference in Pittsburgh, Pennsylvania, U.S., academics and physicians met to discuss the latest research in radiology. Hundreds of scientific posters and presentations were on display, and experts from all subfields of medical imaging poured over the latest theories and breakthroughs. The judges gave the first prize for research posters to a group from the University of Pennsylvania titled "Diagnostic Quality of Machine Learning Algorithm for Optimization of Low-Dose Computed Tomography Data."
This poster, simple and straightforward, described what could be a huge breakthrough in CT. The authors used a patented algorithm approved by the U.S. Food and Drug Administration (FDA) to assess the diagnostic quality of algorithmically enhanced low-dose CT images. In essence, CT scans were performed at ultralow radiation dose, almost equivalent to that of a simple chest x-ray, and compared with normal high-dose CT images. Lead author Dr. Nathan Cross found that 91% of AI-enhanced low-dose images were assessed by radiologists to be diagnostic, whereas only 28% of unenhanced images were diagnostic.
This is akin to those scenes in detective dramas where the characters are looking at a screen of grainy, closed-circuit TV footage or photos and someone shouts "Enhance!" at the IT guy, who magically taps away at the keyboard and instantly makes the images perfectly clear. This is for real, though, and it works on CT images. Even better, it works on CT images taken at radiation doses far lower than standard techniques, from any vendor.
The developers of the algorithm -- PixelShine, from a preseries B start-up called Algomedica, based in Sunnyvale, California, and for which I work as an adviser -- claim that the original noise-power spectrum (noise texture at all frequencies) is entirely maintained while improving diagnostic image quality by reducing noise magnitude. This is something that typical dose reduction techniques, such as iterative reconstruction, fail to do effectively. (In fact, the more reconstructive iterations you use, the worse image quality gets.)
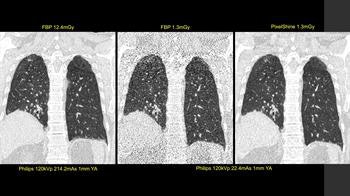
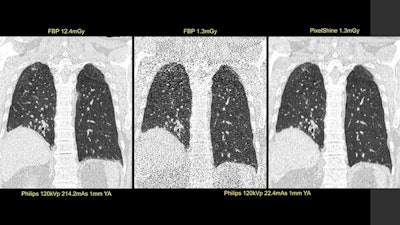
First image: Standard, high-dose CT at 12.4 mGy. Second image: Ultralow-dose CT at 1.3 mGy. Third image: AI-enhanced ultralow-dose CT at 1.3 mGy. Diagnostic image quality between the first and third images were rated as comparable by independent radiologists, despite a significant dose reduction of 11.1 mGy. The second image is noisy and nondiagnostic.
The potential implications for this kind of technology are game-changing. If proved to work robustly without losing clinically important detail, there is scope to reduce standard CT radiation exposure by several orders of magnitude.
Depending on the body part being scanned and other factors, current radiation doses are roughly equivalent to spending an hour at ground zero at Chernobyl. This is not enough to pose any significant risks, but having multiple scans will increase individual risk over time, such as tissue damage and inducing new cancers.
Vendors of CT scanners are under a sort of perverse incentive not to reduce dose too much, to maintain the image quality that radiologists expect, although companies do offer some dose reduction techniques. However, by performing ultralow-dose CT and then postprocessing the images using AI, these risks can be vastly mitigated, especially in children, bringing the radiation dose close to near negligible, e.g., the same amount of radiation as taking a long-haul transatlantic flight. In addition, this could theoretically bring down the cost of CT scanners by negating the need for expensive, high-powered components, but again something the big vendors may not like. Therein lies the true disruption.
There are guiding principles in radiation safety known as ALARP and ALARA (as low as reasonably practical/achievable), whereby the radiation exposure given for a medical procedure must be as low enough as possible while still maintaining diagnostic utility. The new potential for deep-learning algorithms in enhancing ultralow-dose CT may well redefine what we consider to be practical and achievable.
The cherry on the cake is these algorithms work on images produced by any CT vendor, at any dose, meaning scalability and adoption are low barriers to overcome. Eagerly awaited are the results of clinical trials set up to establish just how effective these algorithms can be.
So, how does this translate to a 10-minute MRI scan?
Research is underway into the use of deep-learning networks for the enhancement of undersampled MRI image data. Where CT has the downside of radiation, MRI has the downside of prolonged acquisition time. If algorithms can be developed to enhance noisy, grainy, undersampled MRI images produced in shortened time frames, then there is the potential to reduce time spent in the MRI scanner by up to two-thirds.
To put that in perspective, a standard study for an MRI of the lumbar spine takes about 30 minutes. Deep learning algorithms could reduce that to a mere 10 minutes. That's far less time spent listening to the grim reaper banging away at your temporary coffin!
Research in deep learning applications is relatively new in this field, with some activity going on at both Newcastle and King's College in the U.K., but published results relating to deep learning are in short supply.
Reducing MRI times using sparse image data acquisition is achievable and available, but doesn't produce the results that deep learning promises. Only just recently have researchers at Stanford University in California reported some results on PET with radiotracer dose reduction of up to 99%, for instance. This has particular relevance to the U.K., which is potentially leaving the European Atomic Energy Community (Euratom) as part of Brexit, and therefore won't have as plentiful access to the radioisotopes required for PET imaging.
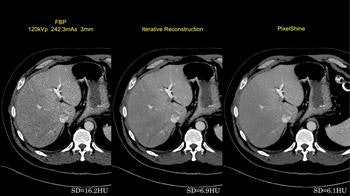
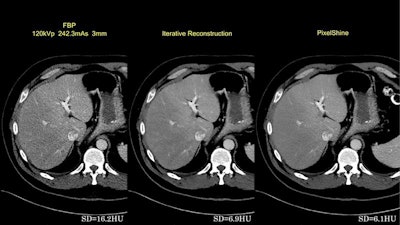
First image: Filtered back projection standard dose CT leaves a high standard deviation (SD) of pixel values. Second image: Iterative reconstruction (IR) improves SD. Third image: Postprocessed image improves on the SD of IR.
No doubt the big vendors of PET, MRI, and CT scanners will also be aware of this potential niche; they have, after all, introduced brand-specific dose reduction techniques, such as iterative reconstruction for CT. The race will soon be on to find out who can provide the best-quality MRI images in the shortest timeframes, and PET and CT at the lowest radiation doses.
This work, of course, needs to be supported by high-performance computing infrastructures, because MRI data are much larger than other imaging modalities. It will also need heavy input from radiologists to advise on acceptable levels of image quality to ensure diagnostic and clinical safety, as well as medical physicists to advise on adjusting the scanners' acquisition parameters, but these technical and collaborative challenges are worth overcoming if the ultimate goal is to be achieved.
An improvement in MRI throughput
Assuming the technology is proved to work, an increase in MRI patient throughput by up to threefold would certainly have a significant positive impact on waiting times for imaging studies, especially for musculoskeletal problems and cancers that are the mainstay of MRI. Similarly, reduced radiation exposure would enable increased and more liberal use of CT scanning.
Hospitals may even see a decline in plain-film, standard x-rays (which aren't very accurate anyway), as both MRI and CT become the go-to modalities. I can certainly envisage scenarios in which, for example, emergency room patients with suspected wrist fractures go straight to a fast MRI scan, and patients with shortness of breath avoid a chest x-ray and go straight to a low-dose CT chest.
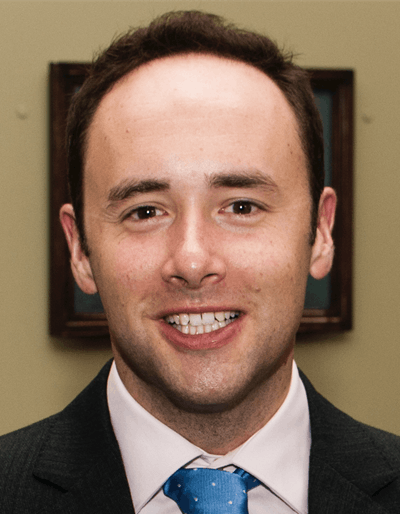
PET/CT is likely to become a hospital's first-line investigation of choice for cancer diagnosis, rather than the adjunct it is now. This trend might also increase the need for small, dedicated scanners for certain body parts; an ankle/knee MR machine doesn't need to be anywhere near as large as its whole-body cousin, and a brain CT scanner can now fit inside an ambulance, providing immediate access to acute imaging for life-threatening conditions such as stroke at a fraction of current radiation doses.
Of course, these developments and implementations must be supported by providing increased resources for the surrounding infrastructure. Money spent here will help lead to expected savings down the line. Increased staffing would be required both for operating the equipment at higher throughput (radiographers) and for reporting the increased volume of images (radiologists -- unless AI image perception algorithms take over, but that's a separate argument). Also, it is important to consider how to improve scheduling of appointments to accommodate the increased throughput; there may be a need for larger patient waiting areas and better facilities for getting patients changed into hospital gowns, and in and out of scanners.
None of this is beyond the realms of possibility, but it will take hard work to get there. While the algorithms in question are still in the clinical validation phase, the research certainly has great potential. More hospitals must engage at the early stages with this type of work, and more trials should be conducted.
Until then, it will take time before we can save time, save lives, and improve the standard of care.
Dr. Hugh Harvey is a consultant radiologist at Guy's and St. Thomas' Hospital in London. He trained at the Institute of Cancer Research in London, and was head of regulatory affairs at Babylon Health, gaining the CE Mark for an AI-supported triage service. He is a Royal College of Radiologists informatics committee member and adviser to AI start-up companies, including Algomedica and Kheiron Medical.
The comments and observations expressed herein do not necessarily reflect the opinions of AuntMinnieEurope.com, nor should they be construed as an endorsement or admonishment of any particular vendor, analyst, industry consultant, or consulting group.