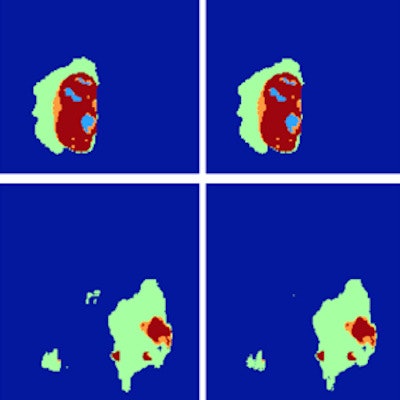
Artificial intelligence (AI) promises to transform radiological reading, and the imaging community must gear up for revolutionary diagnostic tools far removed from today's visual representations, a U.K. expert said at the European Society of Medical Imaging Informatics (EuSoMII) meeting in Rotterdam, the Netherlands.
"As machines are faster than humans for trawling through vast amounts of data, costs will be reduced. More importantly, for particular applications such as cancer screening, we will improve the error rates of human experts. This will directly impact the lives of millions of people," Ben Glocker, PhD, senior lecturer in medical image computing at Imperial College London, told AuntMinnieEurope.com ahead of the meeting. "The human expert also will be equipped with more advanced computational tools to analyze patient data to improve clinical decision-making."
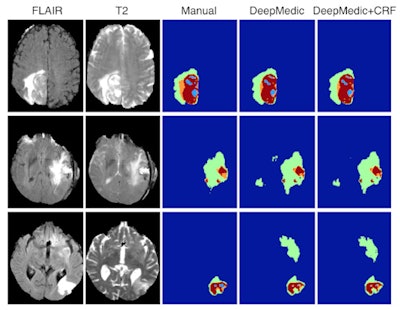
During his presentation on 18 November, Unlocking patterns in medical images with AI, he described how machine learning can make the diagnostic process more reliable.
Unlocking disease patterns
Machine learning is used in a wide range of projects at Imperial College and these are helping to reveal how the technology can unlock patterns and subtle signs of disease often difficult for humans to spot, due to the complex nature of the imaging data. Their machine-learning algorithms automatically detect and quantify brain lesions and can analyze the structure and function of the heart. Other projects include whole-body imaging, where algorithms are helping radiologists find abnormal and suspicious regions.
Glocker presented a number of example applications where his group successfully applied a form of machine learning called deep learning. He included results from brain, cardiac, and whole-body imaging, where the group's algorithms were close to the accuracy of a human expert.
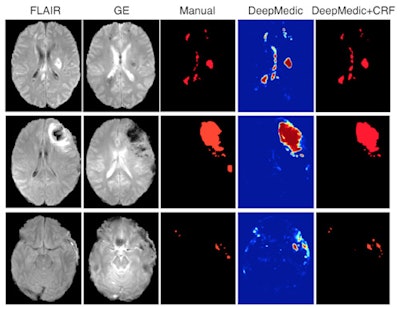
For the moment, the readiness of machine learning for clinical use is a mixed bag, he explained. While for some applications such as breast cancer screening, AI technology is very close to being available in routine practice, in other areas, research remains at an early stage. One start-up company, Kheiron Medical Technologies, is close to bringing a breast cancer screening tool to market, but wide-scale commercialization of other apps will take many more years, he added.
The key to routine clinical use lies in making AI systems resilient enough to handle the entire range of variation in clinical practice.
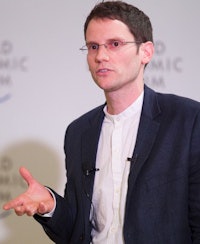
"The biggest challenge is to make deep-learning tools robust. Clinical data is very heterogeneous and current methods are not robust enough to cope with changes in the data," Glocker noted. "Another challenge is trustworthiness. Deep-learning tools behave like black boxes, and we need to make them more reliable and more interpretable, for gaining the trust of doctors, patients, and policymakers."
In the longer term, he suggests that humans will make use of AI to develop entirely new diagnostic tools.
"Currently, the main focus of research is on replicating what a human expert would do and then improving on top of that. But that might be a bad starting point," Glocker said. "Medical images are generated for visual inspection by humans, but that might not be the right representation of the underlying processes happening in the body."
For an algorithm to draw conclusions from the data and make the best possible predictions, the data representation might "look" very different from anything that humans are used to interpreting, he continued.
Hype control
Besides specific improvements in subspecialties, he envisages that AI's general impact on radiology will be significant, helping to overcome problems such as costs, staff shortages, and comparatively high error rates in some areas of image-based diagnosis.
However, he urged AI proponents to be careful not to overpromise and sensationalize what can be done with today's technology. Early diagnosis and detection of disease are important, but because outcome depends on many other factors, the right strategies for treatment and prevention are just as crucial.
"Breast cancer screening is certainly an area where if we can improve today's practice, it will have a huge impact on society," Glocker said, adding that in the short term such a leap may also be seen in cardiac imaging. "We must be careful about the hype surrounding deep learning, as many more years of research will be needed for other aspects."
He also stressed the human expert would need to remain in the loop.
"Domain knowledge is key for inclusion in deep-learning models. We won't solve the challenges in a purely data-driven way," he said.