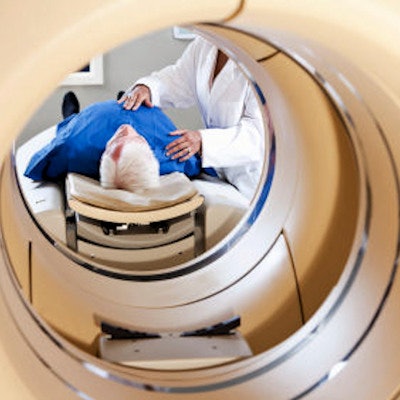
Radiomic factors -- that is, image-based tumor phenotypes -- gleaned from F-18 FDG PET exams can help clinicians personalize care and better predict treatment outcomes for patients with head and neck squamous cell carcinoma (HNSCC), according to a study published on 7 September in EJNMMI Research.
The study findings could translate to better treatment decisions and outcomes for HNSCC patients, wrote a team led by Dr. Roland Martens of Amsterdam University Medical Center in the Netherlands.
"More efficient pretreatment response prediction may result in patient-tailored escalation or toxicity-reducing de-escalation ... of (chemo)radiotherapy or a switch to different treatment options (e.g., surgery)," the group wrote.
Patients with head and neck squamous cell carcinoma often undergo radiotherapy -- with or without chemotherapy. But this treatment fails in half of cases, with cancer recurring in 90% of patients within the first two years after treatment, Martens and colleagues noted.
The researchers hypothesized that developing a list of quantitative F-18 FDG PET radiomic features in patients with HNSCC could help predict recurrence, metastasis, and overall survival who had undergone chemotherapy and radiation therapy treatment.
The study included 71 patients who had F-18 FDG PET/CT imaging between 2012 and 2018. From these exams, the researchers extracted 434 radiomic features that they then assessed for their predictive value, tracking correlation with clinical factors (including human papillomavirus infection [HPV] status, which can affect a person's vulnerability to radiation) and PET parameters such as mean standardized uptake values (SUVmean).
Of the 434 radiomic features, the group ended with 124, which researchers then consigned to eight categories. These included the following:
- Factor 1: Least axis length, nonuniformity, high dependence of high grey levels
- Factor 2: Histogram range and contrast, dissimilarity, cluster prominence
- Factor 3: Histogram gradient and long run low gray-level emphasis
- Factor 4: Volume-difference, coarseness, and gray-level nonuniformity
- Factor 5: Aspherity, major axis length, inversed compactness, and inversed flatness
- Factor 6: Histogram variation coefficient
- Factor 7: Inversed small-zone low gray-level emphasis
- Factor 8: Inversed difference features
Martens and colleagues evaluated the prognostic performance of the radiomics-based prediction models using area under the receiver operating curves (ROC) and concordance indexes. They found that the following clinical/radiomic combinations offered the best predictive value for HNSCC:
Radiomic and clinical factors that predict HNSCC outcomes | |||||
Measure | Factors | Training set ROC | Validation set ROC | Training set concordance | Validation set concordance |
Distant metastasis prediction | Metabolic active tumor volume | 0.625 | 0.627 | 0.657 | 0.627 |
Overall survival | HPV status
SUVmean SUVmax Factor 1 Factor 5 |
0.763 | 0.764 | 0.751 | 0.764 |
Recurrence prediction | HPV status
SUVmean SUVpeak Factor 3 Factor 4 Factor 6 |
0.801 | 0.644 | 0.779 | 0.645 |
The findings are promising, but more research is needed, according to the investigators.
"This study was hypothesis generating and the feasibility was tested," they concluded. "However, in the next step to clinical translation, more extensive validation and refinement on larger and external datasets as well as evaluation of the clinical applicable calculators is needed ... Our study suggests that adding radiomics to the [F-18 FDG] PET image analysis can improve prognostication as a step toward personalized treatment of HNSCC patients."