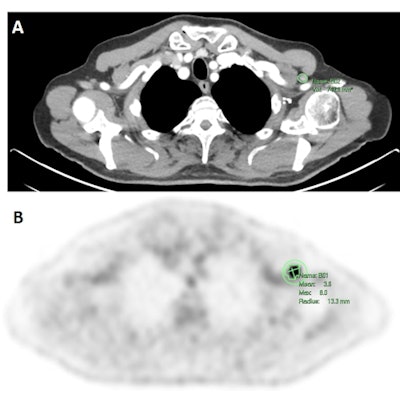
A machine-learning model based on PET/CT radiomics can differentiate between metastatic breast cancer and swollen lymph nodes due to COVID-19 vaccination, according to a study published on 6 April by European Radiology.
An Israeli group investigated whether an artificial intelligence algorithm trained on PET/CT radiomics features could differentiate between metastatic axillary lymphadenopathy in breast cancer patients and FDG-avid reactive axillary lymph nodes in people who received COVID-19 vaccines (Comirnaty, Pfizer-BioNTech).
The model achieved a high accuracy and provides an approach for a conundrum that so far has proven very difficult, wrote Dr. Michal Eifer, a diagnostic imaging resident at Chaim Sheba Medical Center and Tel Aviv University, and colleagues.
"Discrimination between COVID-19 mRNA vaccine-associated FDG-avid lymph nodes and malignant lymph nodes using qualitative and quantitative variables may be impossible," they noted.
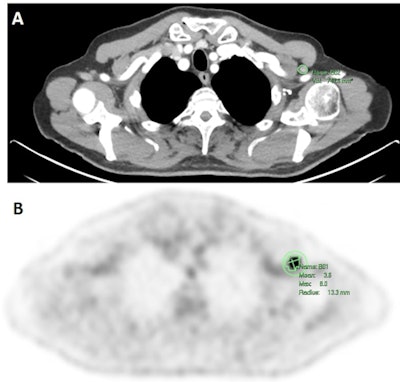
The presence of ipsilateral FDG-avid axillary lymph nodes after COVID-19 mRNA vaccinations is a frequent and challenging finding on imaging, specifically in malignancies with a tendency to involve the axilla, such as breast cancer.
Since the initiation of the worldwide COVID-19 vaccination rollout, Eifer and colleagues have reported on the incidence and intensity of FDG-avid axillary lymph nodes ipsilateral to injection sites and described associations between FDG avidity and variables such as age and immune status.
Study logistics
In this pilot study, the researchers investigated whether radiomics with machine learning could distinguish between potentially deadly metastatic nodes and nodes that mimic cancer after vaccine shots in F-18 FDG-PET/CT scans.
The group retrospectively analyzed FDG-positive, pathology-proven, metastatic axillary lymph nodes in 53 breast cancer patients who had PET/CT for follow-up or staging, and FDG-positive axillary lymph nodes in 46 patients who were vaccinated with the COVID-19 mRNA vaccine. A total of 165 axillary lymph nodes were included in the analysis. Eighty-five were pathology-proven breast cancer lymph nodes and 80 were post-COVID-19 mRNA vaccine axillary lymph nodes.
To train the machine-learning models, the researchers extracted 110 radiomics features from PET, CT, and combined PET/CT scans for all segmented lymph nodes. Data analysis was performed using K-nearest neighbors (KNN) and random forest (RF) supervised machine-learning classification models. The models' performance was evaluated using area under the receiver-operator characteristic curve (AUC) score.
Key findings
The machine learning analysis of the three input data sets -- CT, PET, and combined PET/CT -- using the RF and KNN models showed that the combined PET/CT input had the highest AUC values, followed by the CT and PET inputs.
The KNN model showed the best performance score for combined PET/CT, with a validation AUCs of 0.98 and a validation accuracy of 0.88%. The RF model showed the best result for CT input, with a validation AUC of 0.96 and validation accuracy of 90%, according to the findings.
"Our analysis demonstrates that FDG PET/CT radiomics features can distinguish between breast cancer and reactive COVID-19 mRNA vaccine lymphadenopathy," the authors wrote.
First-order variables, specifically those reflecting tumor homogeneity, such as entropy and uniformity, were among the most statistically significant variables for distinguishing metastatic from post-COVID-19 vaccine-associated lymph nodes, they added.
While the use of radiomics with CT and MRI has become increasingly popular, the approach has only recently begun to be applied to PET imaging, the authors noted.
"Further larger studies are needed to validate these results and to evaluate if this method can also be applied to differentiate benign lymphadenopathy from a malignant one in other conditions where the clinical assessment is not so helpful," Eifer and colleagues concluded.