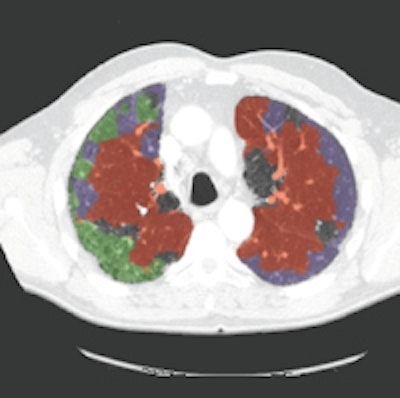
A new semiautomatic method for the classification of abnormal lung textures has been developed by researchers from the Netherlands. The interactive labeling protocol is more efficient to use than a fully automated alternative, and reduces the time taken to analyze a whole CT scan manually by over two-thirds.
With around two hundred different types of interstitial lung diseases, each of which requires a specific treatment plan, correct patient diagnosis is both a vital and complicated process. Diagnoses are made through a combination of pulmonary function testing, lung tissue analysis, and imaging, with CT chest scans playing a central role. In these scans, interstitial lung diseases typically present themselves in the form of abnormal lung textures -- which may include increases in lung density, patterns of nodules, and cysts whose walls form a honeycomb-like pattern.
Even for experienced radiologists, the classification of these textures in 3D lung CT scans is a time-consuming process, taking into account patient history, 3D anatomy, and individual scanner parameters. This is further complicated by the tendency of the imaging features of different lung diseases to overlap.
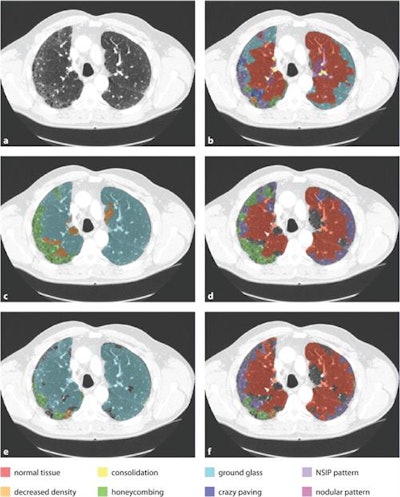
In their new study, Thessa Kockelkorn of the Images Science Institute at UMC Utrecht and colleagues developed one automatic and two interactive protocols for classification of abnormal lung textures. They tested these on scans of 10 patients who exhibited various types of lung disease and corresponding texture patterns. In each protocol, lung CT scans are segmented, and then subdivided into volumes-of-interest (VOIs) containing homogenous lung textures (Physics in Medicine and Biology, 20 July 2016, Vol. 61:16, pp. 5906-5924).
In the automatic method, the VOIs of one scan were labeled by an Extra-Trees classifier based on previously made annotations of the other scans. "In the interactive protocols," Kockelkorn explained, "an observer iteratively trains a classifier to distinguish different interstitial lung disease textures by correcting mistakes the classifier makes in a slice-by-slice manner." One interactive protocol started with training data from previous scans, the other from scratch.
The interactive protocols are considerably less time-consuming than manual labeling, Kockelkorn said, taking only 12 minutes for the user to label all the VOIs in a scan, compared with 45 minutes for manual classification.
In the automatic protocol, observers needed to relabel an average of 58% of the VOIs. In the interactive protocol without training data, the number of VOIs requiring relabeling decreased from 64% in the first slice to 13% by the second half of the scan, with 21% needing correction overall. With training data, the number of incorrectly labeled VOIs decreased from 56% to 13% in the second half, with only 20% requiring correction overall. Based on these results, Kockelkorn said: "The interactive approach we describe is a promising tool to make annotation of complete scans practically feasible."
With the development of new screening programs for lung disease, and improvements in scanner technologies leading to larger, higher-resolution datasets, workloads for radiologists interpreting lung CT scans will increase. Given this, "computer-assisted analysis of lung CT scans is inevitable," commented Joe Reinhardt -- a biomedical engineer at the University of Iowa who was not involved in this study. He added: "I believe [this] paradigm of the radiologist interacting with a computer system to 'annotate and correct' a complete lung CT volume is the best approach ... It will save time, improve efficiency, and reduce errors due to human fatigue."
With their initial study complete, the researchers are working to further improve the accuracy of their interactive classification protocol by using training data from previously annotated scans, as well as incorporating additional inputs from the protocol operator. At the same time, they are working to combine interactive texture analysis with interactive lung segmentation to help determine aerated lung volumes in patients who require mechanical ventilation.
© IOP Publishing Limited. Republished with permission from medicalphysicsweb, a community website covering fundamental research and emerging technologies in medical imaging and radiation therapy.