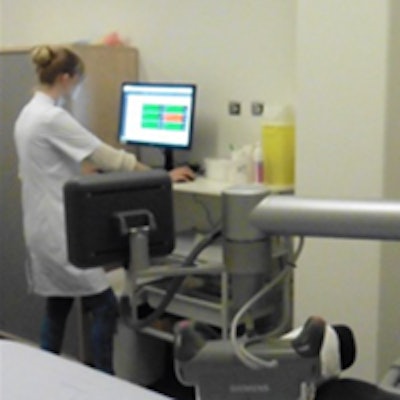
A new technique detects artifacts in automated 3D breast ultrasound at the time of acquisition, enabling sonographers to ensure that images have been acquired adequately before sending patients home, according to a study in the Journal of Medical Imaging.
Researchers from Germany, the Netherlands, Belgium, and Japan were involved in the project, which improves the reliability of 3D breast ultrasound, a tool that has become indispensable in the care of many women with dense breasts. The team developed an image quality assessment system for automated breast ultrasound (ABUS) that looked for three common artifacts. Depending on the artifact, the tool revealed excellent sensitivity for the detection of image shadowing and other common artifacts.
"To our knowledge, this was the first time that the presence and importance of different specific imaging artifacts in ABUS images was analyzed in a reader study," wrote lead author Julia Schwaab from software developer Mediri of Heidelburg, Germany in an email. "We showed that it is possible to reproduce the human perception of image quality in 3D breast ultrasound images by using image processing and machine learning algorithms."
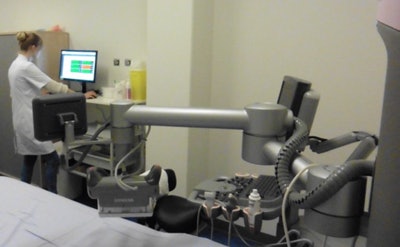
The algorithm can be applied to MRI images as well, she added. The proposed algorithms work quickly and reliably, enabling the system to evaluate image quality during a patient appointment, wrote study authors Julia Schwaab, Dr. Albert Gubern-Mérida and colleagues (Journal of Medical Imaging, 25 April 2016).
Gaining ground
Three-dimensional ABUS is gaining ground in breast cancer screening as an adjunct to x-ray-based mammography, thanks to its prowess in detecting small invasive cancers that can't be seen on mammography in women with dense breasts. Ultrasound is inexpensive and radiation-free, though highly operator-dependent, Schwaab et al wrote.
"Bad skin contact or slight misplacement of the transducer during ABUS acquisition produces imaging artifacts, which may obstruct a complete diagnostic evaluation," the study team wrote. "This may lead to a recall of the woman for subsequent additional imaging, which increases screening costs."
Recall rates of up to 19% have led to considerable extra time and expense performing breast ultrasound. Technologists need sufficient training time before they are able to produce artifact-free images because correct positioning of the transducer frame is essential, the authors wrote.
Automated image quality assessment could support technologists in recognizing image artifacts during or right after exams, allowing scans to be repeated while patients are still in the exam room. In this way, correct artifact detection would help anticipate and potentially avoid recalls. Once the patient leaves, a recall can take several days.
Yet despite active research in MRI and handheld-ultrasound, little has been done to ensure that ABUS images are of diagnostic quality. In a rare prior study, the current authors looked at artifacts considered most disruptive to the diagnostic process that could yield the greatest improvement by rescanning. The three most disruptive artifacts included shadowing caused by the nipple, the position of the nipple relative to the rest of the breast, and the shape of the breast contour.
"If we manage to achieve high specificity in artifact detection, avoiding unnecessary rescans, such a tool could not only lower the number of recalls that cost time and money but also help to train the technicians," Schwaab et al wrote.
Three problems
The group developed an automated image quality assessment system they called AQUA to detect three key ABUS artifacts during acquisition. The algorithm combines image processing and machine learning to detect these artifacts based on 368 clinical ABUS images that were previously examined by two experienced clinicians. AQUA addresses each of the three main artifact problems in ABUS:
- With the nipple positioning problem, the nipple can be pushed very close to the edge of the breast in the coronal view, causing posterior acoustic shadows and obscuring anatomic structures behind the nipple. Rescanning generally solves the issue. In the study, several processing steps were performed to extract the breast mask, focusing on the breast contour.
- The second problem, nipple shadowing, results from imperfect contact between transducer and skin, leaving an acoustic shadow behind the nipple. Applying more contact gel can fix the problem. The algorithm obtains the nipple position from the DICOM header. The size of the dark cylindrical region around the nipple position was estimated by counting cylinder segments with low pixel intensity.
- The third problem involves transducer positioning when the breast is insufficiently supported by cushions, leaving a lack of contact and inability to image other breast regions. The nipple position relative to the rest of the breast is important because it relates to acoustic shadows that hamper interpretation. The technologist provided the absolute nipple position during acquisition, and the information was combined with breast contour data.
The technologists marked the nipple position manually after each measurement and stored the data in the DICOM header of the corresponding file for use in further image processing. The group first merged each of the three image quality aspects individually, then merged features with insufficient image quality as a fourth classifier because there were so many of them. Image processing and machine learning algorithms were combined to detect these artifacts based on 368 clinical automated breast ultrasound images that were previously rated manually by two experienced clinicians.
The study included 815 ABUS volumes from 114 women divided into two datasets. All images were acquired using one of two ultrasound scanners, Somo-v automated 3-D breast ultrasound system (U-Systems) or the Acuson s2000 ABVS (Siemens Healthcare). Image analysis combined image processing and machine-learning algorithms to reproduce the perception of 3D image quality in breast ultrasound, the authors wrote.
High detection rate
Specificity was 99%, as 55% of the images that were rated as low quality were detected by the algorithms. The areas under the ROC curves of the single classifiers were 0.99 for the nipple position, 0.84 for the nipple shadow, and 0.89 for the breast contour shape.
"The proposed algorithms work fast and reliably, which makes them adequate for online evaluation of image quality during acquisition," the group wrote.
The concept can be extended to other modalities, with promising early results in MRI. The prototype the group installed at Radboud University Medical Center in Nijmegen took about two minutes per scan to produce a quality rating, Schwaab wrote, a reasonable time.
Still, half the computing time was due to data transfer and the "indirect" connection to the clinic network, and could be reduced by direct coupling of the system to the scanner and the PACS, Schwaab wrote.
"This is a great opportunity to improve the overall quality of 3D breast ultrasound images in daily clinical care, for screening purposes as well as for diagnostic imaging," Schwaab told AuntMinnieEurope.com. "On the one hand, this will facilitate the image read for the radiologists and potentially save time and money. The greatest effect we observed in a first clinical study with a prototype is, however, that the acquiring technicians get sensitized to the aspect of image quality. Our system gave them additional motivation to produce high quality scans."
Importantly, she said, it will be possible to review images automatically before woman leaves a breast ultrasound appointment, she continued.
The algorithm needs fine-tuning, particularly regarding preferences and practice patterns of the individual clinicians, before it can be commercialized, Schwaab said.
"We are working on algorithms that complement the existing system," Schwaab wrote. "The modular structure of our system allows to add further algorithms at any time and to adapt it to the preferences of the user, not only with respect to the covered image quality aspects but also concerning the sensitivity of the system."
Going forward, the group is looking at automatic detection of air artifacts that produce deep shadows in the images due to a lack of ultrasound contact fluid between skin and transducer, Schwaab explained. These artifacts can cover up to several cubic centimeters of the image and thus have to the potential to mask lesions.