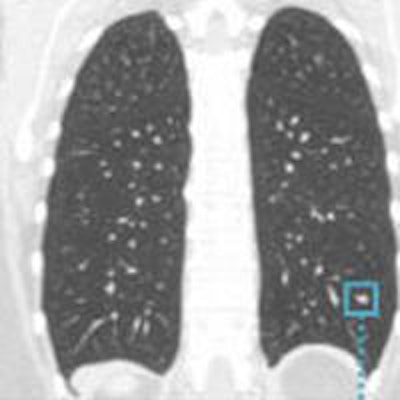
The performance of an automated nodule classification scheme doesn't vary depending on how the nodule is segmented, whether manually or semiautomatically, concluded new research from the U.K.'s Oxford University and Mirada Medical.
The retrospective study looked at thin-section CT images from more than 300 pulmonary nodules at a single hospital region, determining ground truth diagnosis and then comparing the performance of the two segmentation methods in achieving it. There was no discernable difference in performance, and agreement with nodule feature values was high regardless of segmentation method.
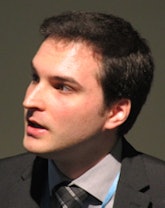
"We found no difference between manual segmentation and [semiautomated] segmentation with an area under the curve of 0.85 in both cases, and if we combined training and test datasets in a different fashion, by training on the manual and testing on the [automated] or doing the opposite," results were unchanged, said Julien Willaime, PhD, a research scientist with Mirada Medical, in a presentation at this month's ECR 2016.
Pulmonary nodules are common findings both in lung cancer screening and as incidental findings, so the stratification of nodules on chest CT is an important step for clinical management, he said.
The CADx computer-aided detection (CAD) system supports radiologists in assessing the malignancy potential of nodules by assessing nodule texture and assigning a malignancy score. But does the segmentation method affect the classification results? The answer is unknown.
Willaime and colleagues Drs. Lyndsey Pickup and Djamal Boukerroui, Mark Gooding, and Timor Kadir from Mirada, and Ambika Talwar and Fergus Gleeson from Oxford, aimed to learn the effect of segmentation technique on classification by retrospectively evaluating 322 solid pulmonary nodules from 186 patients to determine if segmentation method played a role in the CAD's malignancy probability scoring.
"We used a training dataset for which the ground truth was known. We then applied this model to new, unseen data in order to estimate the probability of malignancy," Willaime said. "But as you can appreciate, this pipeline is actually composed of many different automatic and user-dependent steps. One such task is segmentation, and in this work we were interested in comparing the use of manual segmentation versus a semiautomated manual segmentation tool on the classification performance of our system."
Summary of results
The CT data from the Oxford University Hospital National Health Service (NHS) Trust region included all pulmonary nodules detected from January to April 2014. The investigators determined ground truth diagnosis either with histology or by observing a two-year stable follow-up.
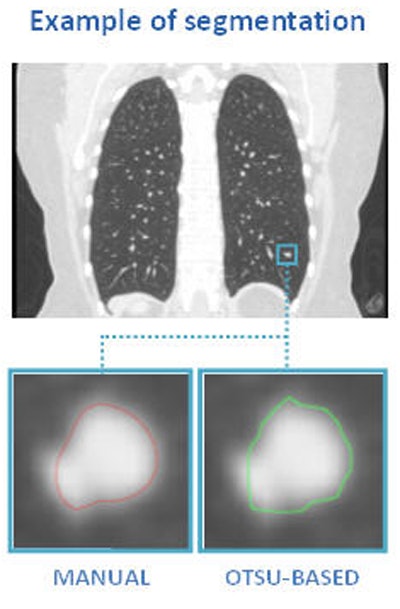
For evaluation, they contoured each nodule manually using a commercial software program (XD3, Mirada Medical), and also using a proprietary semiautomatic Otsu thresholding method. In all, the investigators extracted 792 texture features from each contour. The most discriminative 20 features were then chosen for each segmentation technique, and a support vector regressor function was used to map the features onto malignancy probabilities (method validated by Lee et al, RSNA 2014).
These 20 features were then combined in the model by means of a leave-one-out training and validation method to compare overall performance via area under the curve (AUC). Also compared was the contour overlap between the two methods measured using the DICE score, calculating 95% confidence intervals (CI) of mean differences in normalized Bland-Altman feature values, Willaime said.
Overall, they found that volumes derived from manual (mean 945 mm3) were slightly greater and more varied than the volumes derived from the Otsu method (mean 632.66 mm3).
Moderate agreement, but no difference in classification
"In terms of agreement, we had moderate agreement between the two segmentation techniques," with a median DICE score of 0.82, Willaime said.
When the investigators compared malignancy scores with the ground truth results, they found no difference in classification performance for manual (AUC = 0.85 ± 0.02) and automatic Otsu-based (AUC = 0.85 ± 0.02) segmentation.
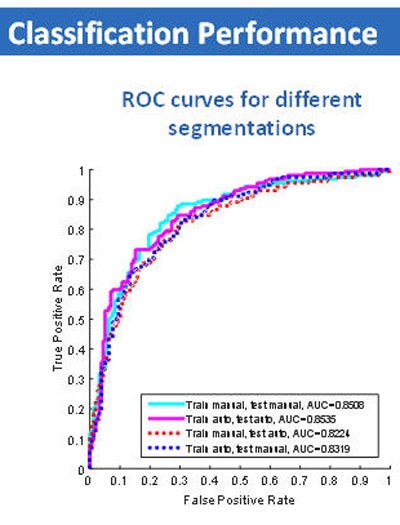
"And if we combined training and testing datasets in different fashions, by training on manual, testing on Otsu, or doing the opposite," the results remained the same, he said.
The mean DICE score was 0.76 ± 0.21 (mean ± SD), and agreement between feature values was high (95% CI was less than 0.23 in all cases).
"We found moderate agreement between the contours derived from both segmentation techniques, but the overall classification performance was not affected by segmentation technique," concluded Willaime, adding that the study was funded by a grant from Innovate U.K.
There was not enough data to test and train on different datasets, a shortcoming that will be corrected in future analyses, Willaime said in response to a question following the talk.