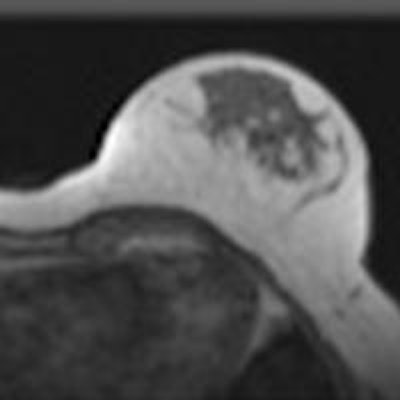
A study using a new computer-aided detection (CAD) algorithm found breast cancers in women whose dynamic contrast-enhanced (DCE) MRI breast exams were thought to be negative, conclude authors in European Journal of Radiology. The research could potentially lead to better cancer detection in women with dense breasts.
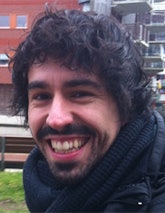
The cohort included 160 patients, consisting of 40 women who were ultimately diagnosed with breast cancer after negative DCE-MRI exams, and another 120 patients whose scans were considered normal after DCE-MRI.
Among the overlooked malignancies, CAD showed 71% sensitivity for visible lesions that turned out to be malignant, and 31% sensitivity for cancers that were minimally visible in the prior scans, also deemed negative.
Many cancers that were overlooked or misinterpreted as negative were found by CAD in exams that were originally considered negative, wrote Dr. Albert Gubern-Mérida from Radboud University Medical Center in the Netherlands and the University of Girona in Spain, along with colleagues from both institutions (European Journal of Radiology, February 2016, Vol. 85:2, pp. 472-479).
"This would appear to offer a real benefit to women with dense breasts who are already at higher risk of malignancy," Gubern-Mérida wrote in an email to AuntMinnieEurope.com.
Screening tool
DCE-MRI has been used for 15 years to screen women with an increased risk of breast cancer, and has been found more sensitive than mammography, especially among women with dense breasts. It is now routinely prescribed in women undergoing screening with breast cancer risk exceeding 20% to 25% by both U.S. and European Union (EU) guidelines, the authors wrote.
It is common practice to search for occult breast cancers among women who were found negative at mammography and eventually developed breast cancer, but reviews of DCE-MRI studies for potentially missed cancers have been rare. A small prior study that did look at negative DCE-MRI among women eventually diagnosed with breast cancer found that 56% of the tumors were identifiable retrospectively.
"The high frequency of positive findings in prior MRI scans reported to be negative (BI-RADS 1 or 2) indicates the importance of developing additional tools to aid radiologists in analyzing DCE-MRI," the authors wrote. A CAD system to highlight abnormalities "could draw the attention of the radiologists to a tumor that might otherwise be overlooked or misinterpreted," they wrote.
The current study tested the performance of CAD for detecting cancers that were previously overlooked in an MRI screening program for women at elevated risk who underwent periodic DCE-MRI scans.
Two groups
The first group consisted of 40 women with increased risk of cancer, defined as 20% to 25% lifetime risk, who were diagnosed with breast cancer a mean 12 months after a negative DCE-MRI exam.
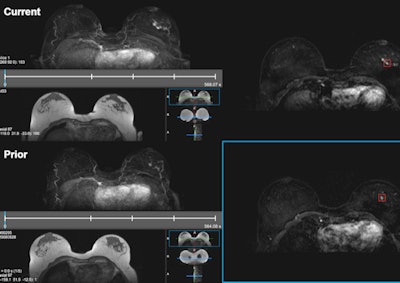
In all, there were 33 invasive ductal carcinomas, five ductal carcinomas in situ, three invasive lobular carcinomas, and one secretory carcinoma. Twenty-four of the lesions were visible or minimally visible in the initial exams, and the remaining 18 lesions were scored as not visible.
The second group of 120 women (median age 46) had no indication of breast cancer or breast surgery. Indications for MRI screening included a personal or family history of breast cancer in 28 patients, and BRCA mutations in 83.
All DCE-MRI exams were acquired on 1.5- or 3-tesla MRI scanners (Magnetom Avanto, Magnetom Sonata, Magnetom Symphony, or MagnetomTrio, Siemens Healthcare) using a dedicated bilateral breast surface coil. T1-weighted gradient-echo dynamic sequences were performed before contrast administration, followed by four or five postcontrast acquisitions.
CAD review
The researchers applied a previously validated CAD system that was developed in-house (Gubern-Mérida et al,Medical Image Analysis, February 2015, Vol. 20:1, pp. 265-274) to all 160 datasets.
The CAD scheme was trained to detect both mass-like and nonmass-like cancers in DCE-MRI data, achieving 89% sensitivity with four false positives in a dataset of 114 normal cases and 95 malignant lesions in the validation study.
The CAD first corrects for motion artifacts and segments the breast. Next, it detects lesion candidates using relative enhancement and descriptors representing a wide variety of local contrast patterns, finally computing malignancy scores for candidate lesions using region-based morphological and kinetic features.
Finding missed cancers
At four false positives per scan, data analysis using free response operating curve (FROC) showed a mean sensitivity of 71% for breast cancers that were visible and 31% for breast cancers that were minimally visible in prior exams, with overall sensitivity of 49% for both, Gubern-Mérida and colleagues reported. Also at four false positives per normal case, CAD's mean sensitivity was 82% for detecting breast cancers in positive MRI scans.
"These results suggest that a substantial fraction of the overlooked or misinterpreted cancers can be detected by an automated CAD system alone," Gubern-Mérida and colleagues wrote. "Based on our findings, one could speculate that a proportion of these overlooked and misinterpreted lesions could be detected if a CAD system ... would be used in clinical practice."
It seems reasonable to hypothesize that CAD would probably contribute more to avoiding oversight errors than to reducing misinterpretation errors, they added, depending on how CAD was applied and the results interpreted.
Setting the system at two to four false positives per scan would probably be acceptable for breast MRI, though the sensitivity of this system would probably need to improve in order to use CAD in this way, they noted.
CAD false positives increased slightly, generating 1.38 extra false positives per case when applied to false-negative prior exams. These exams might contain more suspicious areas such as benign lesions, or other enhancing structures such as annotated tumors that were not included in the original ground-truth assessment.
There are no current plans to commercialize the CAD software -- it doesn't even have a name yet -- but given these positive results, that assessment could change, Gubern-Mérida said. First, the authors aim to do additional research on improving CAD detection.
"We would like to improve our algorithm by training it with a larger amount of screening data," Gubern-Mérida wrote. "Furthermore, given the clear evidence on deep learning technology outperforming all other techniques, we are also currently investigating which steps of our CAD pipeline deep learning could help to improve the overall performance."
Study limitations include a wide variety of MRI acquisition parameters, and among the 97 lesions used to train the CAD, only 18 were detected in screening settings.
"We believe that the performance of the CAD system can improve when trained and tested in more homogenous datasets, and if we specifically train it with screen-detected lesions or on misinterpreted or overlooked breast cancer lesions as examples," they wrote.