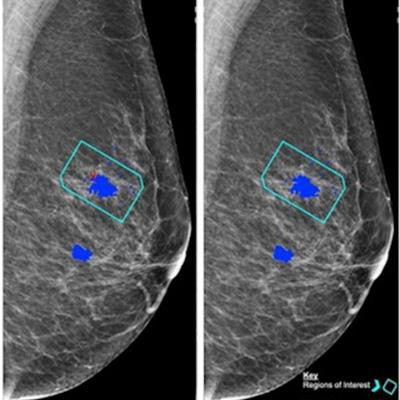
AI showed broadly similar performance levels when compared to breast radiologists for evaluating cases from enriched test sets in a study led by a research group at the University of Nottingham in the U.K.
Scientists led by Yan Chen, PhD, found that a commercially available AI algorithm could go toe-to-toe with human readers when assessing cases from the Personal Performance in Mammographic Screening (PERFORMS) evaluation scheme.
"The use of external quality assessment schemes like PERFORMS may provide a model for regularly assessing the performance of AI in a way similar to the monitoring of human readers," Chen and co-authors wrote in an article published on 5 September in Radiology.
AI continues to gain ground toward everyday clinical use in breast imaging. In the U.K., the National Health Service Breast Screening Program measures the performance of all readers who interpret screening mammograms. It does so via a combination of individual reader real-life performance data as well as data from PERFORMS.
PERFORMS is a requirement for all readers in the program. Each test consists of 60 examinations from the program with abnormal, benign, and normal findings. Readers record their opinions for each case on a web-based reporting platform. They are given individual feedback, including a comparison with all other readers, upon test completion.
Chen and colleagues hypothesized that such performance metrics could be obtained for AI using similar methods. It sought to use test sets from the PERFORMS scheme to compare the performance of a commercially available AI algorithm (Lunit Insight MMG, version 1.1.7.1; Lunit) to that of radiologists reading the same test sets.
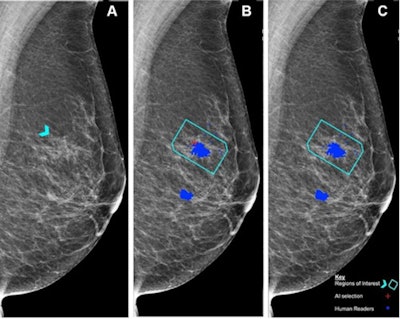
For the study, the investigators included 552 human readers who evaluated the test sets between 2018 and 2021; they used the AI algorithm to evaluate the test sets in 2022. The algorithm considered each breast separately, assigning a suspicion of malignancy score to features detected. The test sets consisted of 161 normal breasts, 70 malignant breasts, and nine benign breasts.
The researchers found no significant difference area under the curve (AUC) at the breast level between the algorithm and the radiologists.
Comparison between AI, radiologist readers for evaluating breast imaging exams | |||
Measure | Radiologists | AI | p-value |
AUC | 88% | 93% | 0.15 |
Sensitivity (using developer's suggested recall score threshold) | 90% | 84% | 0.34 |
Specificity (using developer's suggested recall score threshold) | 76% | 89% | 0.003 |
The researchers noted that when using the developer's suggested recall score threshold, it was not possible to show equivalence due to the size of the test sets.
The team also used recall thresholds to match the average human reader performance, which was 90% sensitivity and 76% specificity. It found that the AI showed no significant differences in performance, with a sensitivity of 91% (p =. 73) and a specificity of 77% (p = .85).
The study authors suggested that their study could be used as a model for assessing AI performance in a real-world setting, noting that performance monitoring is likely to have a role in assessing AI algorithms that are deployed into clinical practice. This is because of product upgrades and concerns that AI performance can decrease over time.
Still, the authors called for more studies to make sure this evaluation model could work for other AI algorithms, screening populations, and readers.
In an accompanying editorial, Liane Philpotts, MD, from Yale University in New Haven, CT, wrote that while AI's exact role in breast imaging is yet to be determined, studies such as the Chen team's "will help move the field in a positive direction."
"As a second reader, it appears AI has a definite role that should ease the demanding job of reading large volumes of screening mammograms," she wrote.
The full study can be found here.