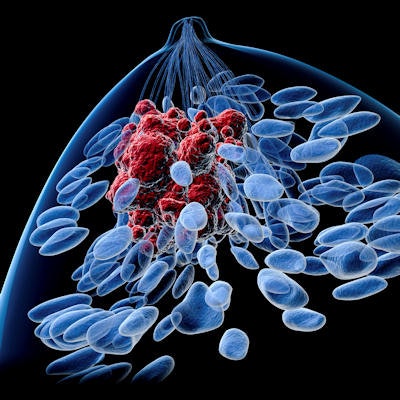
A machine-learning algorithm that analyzes radiomics features on dynamic contrast-enhanced (DCE) MRI images shows potential for differentiating between benign and malignant breast lesions, according to research published on 7 February in Radiography.
The team, led by Nikolaos Stogiannos from University College Cork in Ireland, found that their algorithm achieved moderate accuracy and high negative predictive value.
"The results of this study will enhance knowledge around application and performance of radiomics in breast MRI, thus helping MRI radiographers who use artificial intelligence [AI]-enabled technologies to better delineate the pros and cons of these procedures," Stogiannos and colleagues wrote.
Breast MRI is often praised for its high sensitivity in supplemental breast imaging. However, more recent advances in MRI have added to its lesion-detecting strengths. These include DCE imaging, diffusion-weighted imaging (DWI), and improvements in radiomics, according to the researchers.
In the study, Stogiannos and colleagues sought to utilize a machine-learning algorithm to assess radiomics features on postcontrast T1-weighted MRI sequences and apparent diffusion coefficient map. They trained and tested their model using five-fold cross-validation on MRI data from 52 women with a total of 54 breast lesions.
All patients received DWI as a standard MRI protocol, including DCE-MRI. The participants were examined on a Magnetom Avanto 1.5-tesla MRI scanner (Siemens Healthineers), and radiomics features were extracted from DCE-MRI images. The researchers also performed histological analysis of the breast lesions.
The team reported that the algorithm achieved an overall accuracy of 67% in differentiating malignant lesions from benign ones. This included a sensitivity of 70%, specificity of 66%, and a negative predictive value of 82%.
Additionally, the area under the curve (AUC) was 70%, while the false positive rate and false negative rates were 33% and 30%, respectively.
The study authors reported that these results are comparable to previous studies. However, they pointed out that the other studies used different approaches to separate malignant from benign lesions.
"Hence, it is not safe to adopt a single strategy related to the application of radiomics in breast MRI," they wrote. "The results of this study clearly indicate that extraction of radiomic features derived only from the first series of DCE images and the apparent diffusion coefficient map have the potential to develop into a valuable tool in clinical practice."
However, the authors also noted that there is still an "important translational gap" that needs to be addressed before implementing this latest machine-learning technique into clinical practice. They called for prospective studies with large and representative samples.
"This will facilitate a safe and successful clinical adoption of radiomics and enhance trust between clinicians and the patients," they added.