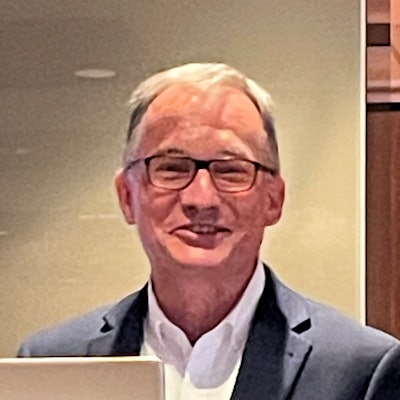
VALENCIA -- The next generation of radiology AI systems needs to focus much more on workflow and efficiency gains to make them affordable and attractive to use in clinical practice, according to Dutch expert Prof. Dr. Mathias Prokop, PhD.
With the recent success of deep learning and convolutional neural networks, AI systems have made a massive leap forward, he said on 14 October at the annual congress of the European Society of Medical Imaging Informatics (EuSoMII). While the ultimate goal is autonomous machines, the urgent need is for systems that can speed up reporting, increase consistency of reporting, and make structured reporting easier and faster.
"AI can do astounding things, like create simulated chest radiographs, remove pulmonary vessels from chest CTs to better detect nodules, speed up MR data acquisition, and many more improvements," he said. "In the future, AI systems will notably enable us to speed up and automate almost every step within lung cancer screening exams, from planning to acquisition and reporting. We're not here yet, but it's just a question of time."
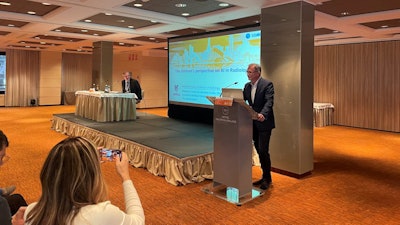
The two things that radiologists need to focus on when planning to implement AI are a good application and the money to pay for it. "We don't usually think about money when we start with test installations, but it's one of the most important factors for a sustainable solution," said Prokop, head of radiology at Radboud University Medical Center in Nijmegen, the Netherlands, and president of the Dutch Society of Radiology (NVvR).
A good application should do what it is supposed to do and do it well, and it should integrate in the radiological workflow. While improving patient outcomes is the ultimate goal for good AI systems, the focus right now should be on workflow, he added.
"Extra reimbursement for AI hinges on improved outcome for patients and requires good clinical studies. That's why very little direct reimbursement for the use of AI is available, at least in Europe. AI business cases need to build on the monetary value of their time savings," Prokop said.
In Europe, direct reimbursement for AI is very rare. "I don't see it coming within the next few years. In most countries, it's not going to happen," he told EuSoMII delegates.
If radiologists want to pay for AI, they need to finance it via savings in radiologist's time or higher throughput -- for example, a new MR scanner featuring AI-boosted reconstruction that makes it possible to scan more patients in the same time.
AI for data acquisition and reconstruction
AI in radiology is working really well in all tasks involved in scanning -- workflow automation, image acquisition, and image reconstruction, according to Prokop.
"Next year I'll try to get a CT scanner with lots of automated features so that I don't need technologists to do a lot of individual tweaking of protocols. Techs can use automated workflows for standardizable care and scan much faster. This lets me provide care to substantially more patients without having to buy more scanners," he said.
"My goal is to have a 5- to 8-minute-long scan slot per patient. Five minutes is something you should be able to consistently do with those systems."
But AI-based image improvement techniques have a limit, Prokop conceded. "If you overdo it, you create great images that don't show what's in the patient. In CT, that means you may get great images in which a stenosis in a coronary artery is cleaned up or a septum in a cyst is removed. This happens if AI is used to reconstruct data with too low a dose. And in MR, you suddenly may no longer see a meniscal tear if you try to speed up the scan too much."
Radiologists will have to experiment where exactly that limit is, he explained.
Looking to the future
Most of the buzz now is in image analysis and reporting, in which multimillion investments have been made, but there has been a bit of a disillusionment lately, Prokop pointed out. "There's been a bit of disenchantment. You can't really earn the huge amount of money that people thought they could earn."
Another drawback is that most AI tools lack validation. "The evidence base of most of the stuff that's out there is doubtful. But we realize it's important, and external validation is increasingly demanded," he said.
About 135 image processing or quantification software are currently available, while 40 solutions have been developed for triage of time-sensitive information. Only 20 systems are available for detection or diagnosis purposes.
"Most of the detection tools that you read about in the literature are still in the experimental phase and going through the approval process. That's the area where most research is done now," Prokop stated.
While a stand-alone performance test is required for image processing and triage tools, clinical studies are required for detection systems to be approved by the regulatory bodies. "That probably adds a zero to the costs and makes it really expensive. It also makes entering the market more difficult."
Some tools already work really well and become interesting especially in settings with a high volume of studies, for instance, breast cancer screening. "We have thousands of patients and the systems work really well, sometimes within or above the range of human observers," he said.
Improved lung nodule classification systems would be a really nice development, Prokop suggested. Nodules can be classified in different clusters depending on their type and their malignancy potential. The closer the features of a nodule are to the typical feature of each cluster, the better the performance of the AI system for this particular nodule.
"If it fits in the typical pattern, then the system often is really sure of what nodule it should be. I'd like to see an AI system that has a level of certainty and says how certain it is. Most don't do that today or don't say it in an easily understandable fashion."
The trend will be for more AI systems that perfectly integrate with PACS and RIIS to make radiology practices more cost-effective. "The more automation we have, the higher the cost we'll be able to save." But even in this setting, human vigilance will be required, for example to correct results if necessary, Prokop concluded.