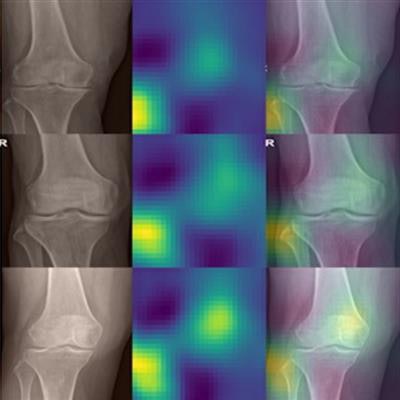
A deep-learning algorithm can help radiographers place anatomical side markers (ASM) on knee x-rays, according to a study performed by German researchers published in Scientific Reports.
The model is based on an off-the-shelf deep learning network and could be used to help prevent manual placement mistakes or missing side markers, which have profound effects on patient safety, the authors wrote.
"Because a wrong ASM can have severe consequences and deep-learning networks are in general black boxes, the prediction of the network could be presented to the radiographer for a second check, making mistakes even less probable," wrote lead author Anton Quinsten, chief radiology technologist at University Hospital Essen.
Left and right anatomical sides are difficult to identify on x-rays of the knees, chest, and other body parts due to symmetrical human anatomy. ASMs are placed in images manually for reliable identification of the anatomical side.
Despite their clinical importance, incorrect or missing ASMs can occur in clinical practice. To highlight this, the authors noted a 2001 Swedish case of a thoracostomy performed on the wrong side in a premature baby with pneumothorax. The lack of a marker on the x-ray resulted in the child's death, they noted in the Scientific Reports article, which went live on 7 March.
"Unfortunately, this is not an isolated case, and many case reports can be found in which a missing ASM in radiographs has led to malpractice," the authors added.
The German group hypothesized that a deep learning model could aid with ASM placements by identifying the left and right sides of the knee in x-rays.
The group used a standard deep learning network (ResNet-34) and trained it on a set of 2,892 x-rays from 2,540 patients culled from University Hospital Essen's radiological records between January 2009 and December 2018. All patients underwent x-rays of the knee in the anterior-posterior direction on x-ray systems from Siemens Healthineers, Agfa HealthCare, or Canon Medical Systems.
The internal validation cohort consisted of 932 knee x-rays from 816 patients, while the external validation cohort consisted of a set of 490 x-rays from 462 patients obtained from a neighboring hospital.
Also, all ASMs were stripped from images for the training of the algorithm, with care taken not to leave visible evidence (black rectangles) that could be picked up by the model.
In the training cohort, the model correctly identified 1,403 left and 1,489 right knees, and 457 right and 475 left knees in the internal validation dataset, according to the findings. Similarly, in the external validation cohort, the model identified 260 left and 229 right knees.
"The final model did not misclassify any image on both the internal and external validation cohorts, thus showing perfect accuracy on all the 932 and 489 images," the researchers stated.
To understand how the network made its decisions from the x-rays, the researchers applied occlusion sensitivity maps for rough estimates of which parts of the knee x-ray contributed to a given decision. As expected, the fibula seemed to be the most important part for determining the anatomical side of the x-ray, the authors wrote.
Unexpectedly, in many images a second hot spot was visible on the opposite side of the fibula and above the intercondylar area. This indicates the model seemed to take into consideration the slant of the lower end of the femur, which seems also to be a rather good indication of whether the knee is a left or right one, the researchers wrote.
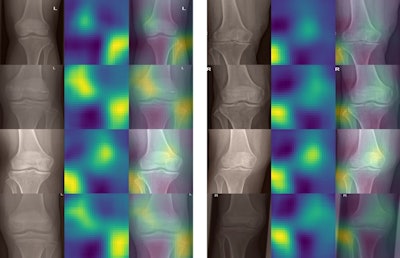
Ultimately, the authors restricted the study to knee radiographs but suggested they have little doubt that similar networks can be developed for identifying anatomical sides for other body parts, such as the chest, abdomen, spine, and upper and lower extremities.
Such studies should be performed in the future, Quinsten and colleagues concluded.