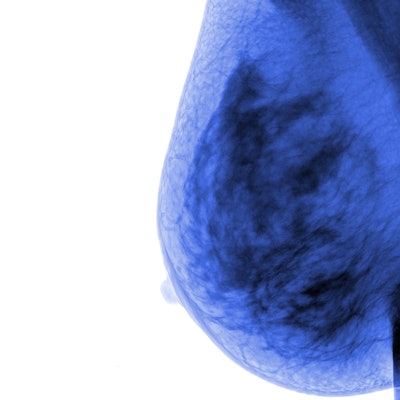
An artificial intelligence (AI)-based software application can reduce inter-reader variability in breast density classifications, enabling more consistent mammography reports, according to research presented at ECR 2021.
After performing a reader study involving nearly 800 mammograms, researchers from the University of Southern California (USC) and AI software developer CureMetrix found that the algorithm yielded significantly more consistent breast density assessments compared with seven experienced breast radiologists.
"This AI-based breast density model, which addresses the subjective and qualitative goals of the BI-RADS fifth edition, shows higher reliability compared to the readers and can reduce subjective reporting variability," said presenter Dr. Alyssa Watanabe of USC School of Medicine in Los Angeles. "This tool can be used to sort cases on the worklist by density, to auto-populate structured reports and tracking systems, and can also be useful in retrieving cases for [Mammography Quality Standards Act] purposes,"
Women with dense breast tissue have a higher lifetime risk of developing breast cancer, and density is included as a variable in version 8 of the Tyrer-Cuzick risk calculator. High tissue density has a masking effect, which decreases mammographic accuracy, said Watanabe, who is also chief medical officer at CureMetrix. The company developed the software -- cmDensity -- that was used in the study.
"This masking effect on mammograms is qualitative and subjective, but it is the recommended methodology based on the current BI-RADS 5th edition," she said.
With the fifth edition of BI-RADS, more mammograms are categorized as dense.
"Also, reader variability is increased due to the increased subjectivity of the assessment," she said. "The percentage system of the 4th edition has been eliminated."
As quantitative breast density methods don't translate well to the qualitative BI-RADS 5th edition objectives, the software developers utilized a semisupervised deep-learning approach, according to Watanabe.
"The model learns to make subjective assessments without the bias of human labeling for training, but with some guidance and therefore not completely unsupervised learning," she said.
The software was assessed in a reader study using a set of 792 screening mammograms that included many challenging borderline samples and came from three institutions, two continents, and three vendors, according to Watanabe. The seven radiologists in the reader study had spent at least 75% of their time reading mammograms for the last three years and read more than 5,000 mammograms each year.
The readers had significant inter-reader variability in their density assessments, producing a kappa of 0.35 for the specific BI-RADS A-D category assessments, as well as a kappa of 0.6 in the less-challenging binary classification of dense versus nondense breast tissue, according to Watanabe.
The AI software also demonstrated a level of agreement with the reader results that correlated with the degree of reader consensus.
"In cases where there was 100% reader agreement, cmDensity was near perfect and was perfect for four-class and two-class assessments, respectively, with kappas of 0.97 and 1.0," she said.
The few outlier assessments for the specific BI-RADS categories were off by just one BI-RADS class, Watanabe said.
The software was also superior in terms of intra-reader variability, yielding an intraclass correlation coefficient (ICC) of 0.99, compared with an ICC range of 0.70 to 0.82 for the radiologists, according to the researchers.