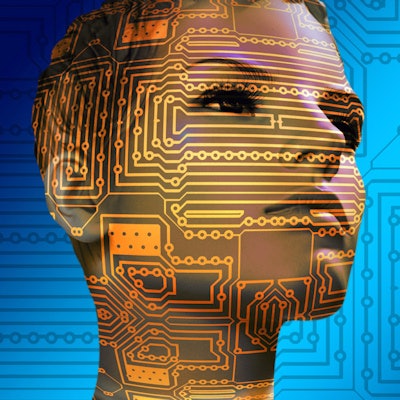
Many research studies claim that artificial intelligence (AI) algorithms are better or equal to human experts for interpreting medical images. But many of these studies are of poor quality and exaggerate claims -- fueling hype around AI and potentially endangering patients, according to research published on 25 March in the BMJ.
A team of researchers from the U.K. and the U.S. reviewed studies that compared the performance of deep-learning algorithms with that of expert clinicians for medical image interpretation tasks involving specialties such as radiology, ophthalmology, dermatology, gastroenterology, pathology, and orthopedics.
They found significant shortcomings in the studies, including a paucity of randomized clinical trials, a low number of prospective nonrandomized studies, limited availability of datasets and code, and descriptive language that suggested comparable or better performance for AI despite significant study limitations.
"Overpromising language could mean that some studies might inadvertently mislead the media and the public and potentially lead to the provision of inappropriate care that does not align with patients' best interests," wrote the authors, led by Dr. Myura Nagendran of Imperial College London.
The methods and risk of bias behind studies on AI performance have not previously been examined in detail, according to the group of researchers, which included evidence-based medicine researcher Dr. John Ioannidis of Stanford University and prominent AI researchers such as Dr. Hugh Harvey of Hardian Health and Dr. Eric Topol of Scripps Research Translational Institute.
To address this shortcoming, the researchers searched several online databases for studies published from 2010 to 2019 that compared the performance of deep-learning algorithms with groups of one or more expert clinicians for predicting -- from medical images -- the absolute risk of existing disease or classification into diagnostic groups such as disease or nondisease.
They found only 10 records for randomized clinical trials for deep learning, including eight related to gastroenterology, one to ophthalmology, and one to radiology. Only two have been published so far: an ophthalmology study and a gastroenterology trial.
Of the 81 nonrandomized trials, nine (11%) were prospective. Only six of these were tested in a real-world clinical environment, however. Radiology was the most common specialty involved in these studies, with 36 (44%) of the studies.
In 77 of the studies, a specific comment was included in the abstract comparing the performance of the AI with that of the clinicians. Of these, AI was reported to be superior to the clinician in 23 (30%) of the cases, comparable or better in 13 (17%), comparable in 25 (32%), able to help a clinician perform better in 14 (18%), and not superior in two (3%). However, only 31 (38%) of the studies included a call for further prospective studies or trials.
After assessing the adherence of these studies to reporting standards and risk of bias, the researchers found a number of issues:
- The median number of experts in the comparator group was only four.
- Full access to all datasets was unavailable in 95% of studies.
- Full access to the code for preprocessing of data and modeling was unavailable in 93% of studies.
- The risk of bias was high in 58 (72%) of the 81 nonrandomized trials.
- Adherence to reporting standards was suboptimal overall, including a less than 50% adherence to 12 of the 29 items in the Transparent Reporting of a Multivariable Prediction Model for Individual Prognosis or Diagnosis (TRIPOD) statement.
The authors called for the development of a higher quality and more transparently reported evidence base in order to help "avoid hype, diminish research waste, and protect patients."