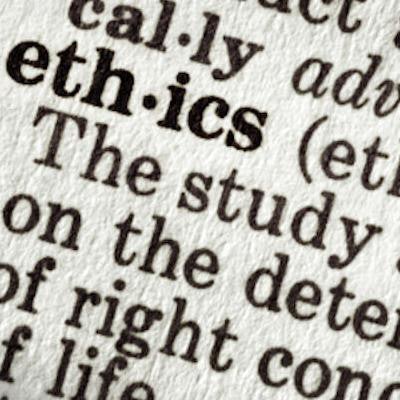
The ethical use of artificial intelligence (AI) in radiology is a complex but necessary endeavor. Dr. Adrian Brady explored the key ethical issues during a presentation on 5 April at the European Society of Radiology (ESR) artificial intelligence course in Barcelona, Spain, which we're covering in a two-part series.
The nature of AI algorithms necessitates awareness of the ethical issues that arise from their training and use, according to Brady of Mercy University Hospital in Cork, Ireland. Before entering clinical practice, the average human physician goes through a prolonged period of training during which he or she is also influenced by family and culture, as well as natural empathy and interaction with other humans, Brady said. AI has not experienced the same influences.
"We can't be quite sure that the robot doctor will bring all of the good baggage of empathy and family and education with him when he starts becoming a practitioner," he said. "So it's our responsibility to ensure that those ethics are imbued into AI when it's utilized on humans."
To address this responsibility, a number of organizations have published papers on the issue of ethics in AI -- both in radiology and in many other applications. For example, the ESR collaborated with six other prominent radiology and imaging informatics societies to publish a draft statement in March on the ethics of AI.
Data, algorithms, and practice
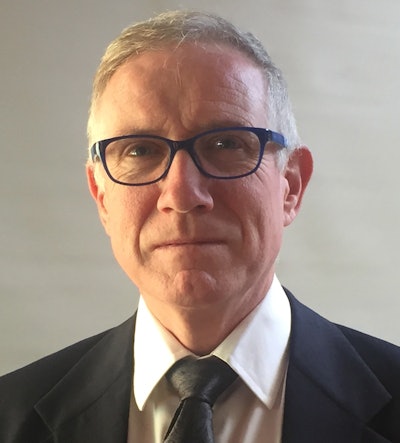
Papers on the ethics of AI in radiology commonly break down the topic into three areas: data, algorithms, and practice. There is a lot of crossover among the three areas, however, Brady said.
Data ownership policies vary around the world. In the U.S., the entity performing the imaging owns the data, although patients have the legal right to a copy of their imaging data. Many hospitals include permission to use data retrospectively for research in their general consent forms for treatment, and consent is not required for deidentified retrospective studies, Brady said. In Canada, healthcare providers that produce medical images own the physical record, while patients share rights to access it. Consent is not required for research relying exclusively on the secondary use of nonidentifiable information.
The situation is very different in the European Union (EU), according to Brady. The General Data Protection Regulation (GDPR) ensures that patients own and control their sensitive, personal, and/or identified medical and nonmedical data. A patient's explicit consent is required for re-use or sharing of data, and consent may be later withdrawn. In addition, the sites where the imaging is performed can also be subject to ownership and copyright regulation, according to Brady.
"So if you use data within the EU for AI applications, for example, it may require consent from imaging facilities and from patients," he said. "It complicates the issue somewhat."
Data elements
Furthermore, Brady noted that CT scanners acquire raw data, which are generally not interpretable by the human eye. Raw data are converted into pixel data, which radiologists view. In turn, pixel data can be augmented by labels such as annotations, radiologist reports, and nonimage clinical data.
These data elements may have different ownership issues, however. The pixel data might be owned by the owner of the imaging machine, for example, while radiologists may own the labels, according to Brady.
"So if data is to be utilized, we have to address the issue of who actually owns it," he said. "This is particularly important if the data is used to build a highly profitable AI product. Who has the rights?"
Brady discussed the data ownership issues that could occur if a hospital sold exclusive rights to imaging data to a company that hopes to build a valuable AI product.
"If patients also retain their right of access, can they also sell their individual data to a rival company?" he said. "Or can they refuse to share their individual data for commercial development, but allow it to be used for nonprofit research? Well, that rather complicates the hospital's arrangement."
That's not just a theoretical issue, Brady said. In 2015, the Royal Free National Health System (NHS) Trust in London agreed to provide Google-owned DeepMind Technologies with access to 1.6 million identifiable patient records at no charge to facilitate the development of a smartphone app for the early detection of kidney disease. The NHS was severely criticized for this arrangement, Brady said.
Data use agreements
Privacy and data protection and ownership are allied issues, he said.
"Is it necessary if patient data is to be utilized to train AI algorithms that each patient would sign a data use agreement with any third-party entity contributing to their health record?" Brady asked. "And we have to ask, [if it is] necessary that their data is to be utilized to train successive iterations of the algorithm, must they give consent for that algorithm? I don't know the answer to this, but data use agreements probably need to explicitly specify what involved parties can and can't do with the dataset, and what they must do to dispose of the data once the agreement expires."
Data use agreements should also be updated regularly to reflect new uses as well as version control specifications, he said. It's probably true, however, that exclusive-use data use agreements are contrary to the common good, as they may prevent a significant amount of useful patient radiology data from being used to develop other AI tools or utilized in other research, Brady said.
Data privacy
DICOM deidentification removes all protected health information from images, but patients can -- with appropriate approval -- be re-identified with DICOM tags, Brady said. DICOM anonymization is a further process that irreversibly breaks the link to the original identification.
That anonymization may not be totally secure, however. For example, a patient head and neck CT that receives 3D reconstruction might be identifiable via spatial recognition software, he said. Furthermore, radiographs may also contain identifying patient information such as personal jewelry or serial numbers on implanted devices.
"It's been said that entities facile with manipulating massive data can likely re-identify just about any radiology exam," he said. "Well, this is a problem if patient data is to be utilized for training commercial products."
In addition, social media companies are adept at identifying individual software usage and online search histories, Brady said.
"It's not beyond the bounds of possibility that a social media company could tie smartphone usage in a particular location to a particular imaging study and thereby identify the patient to whom the images refer," he said. "And that could lead to patients being bombarded with unwelcome advertising or, even worse, extortion if they don't want their medical information made available or known publicly. All of these are issues that must be addressed in terms of data privacy."
Data bias
A variety of data biases can occur that adversely affect AI algorithm training. The key one is selection bias, according to Brady, in which the training sample doesn't accurately represent the patient population. This leads to difficulties in applying the algorithm to the general population. In addition, some training datasets may overrepresent positive or interesting exams, which results in what's known as a negative set bias. As a result, the algorithm might not be adequately trained to recognize normal cases.
Issues can also arise in ground-truth data labeling. For example, a plain x-ray of a wrist performed in an emergency department might be normal; however, if the patient receives another x-ray two weeks later that shows a subtle scaphoid fracture, should the first exam be labeled as "scaphoid fracture present" even if it wasn't visible by the human eye?
"It's not clear," he said. "These are slightly tricky issues."
Above all else, the ethical duty is to minimize bias in AI algorithms, Brady said. There should be transparency about how ground truth is determined for each dataset, and models should be trained using datasets that truly represent the data they will see in practice. Furthermore, it's also important to be aware of automation bias -- the tendency of humans to favor machine-generated decisions and ignore contrary data or conflicting human decisions. This could lead to overreliance on computers and blind agreement with the output of algorithms, he said.
Part 2 of our coverage of Dr. Brady's talk at the ESR AI course in Spain will highlight additional ethical concerns for radiology AI related to transparency, interpretability, conflicts of interest, and workforce disruption.